Learning to Ask Medical Questions using Reinforcement Learning
MLHC(2020)
摘要
We propose a novel reinforcement learning-based approach for adaptive and iterative feature selection. Given a masked vector of input features, a reinforcement learning agent iteratively selects certain features to be unmasked, and uses them to predict an outcome when it is sufficiently confident. The algorithm makes use of a novel environment setting, corresponding to a non-stationary Markov Decision Process. A key component of our approach is a guesser network, trained to predict the outcome from the selected features and parametrizing the reward function. Applying our method to a national survey dataset, we show that it not only outperforms strong baselines when requiring the prediction to be made based on a small number of input features, but is also highly more interpretable. Our code is publicly available at \url{https://github.com/ushaham/adaptiveFS}.
更多查看译文
关键词
reinforcement learning,medical questions
AI 理解论文
溯源树
样例
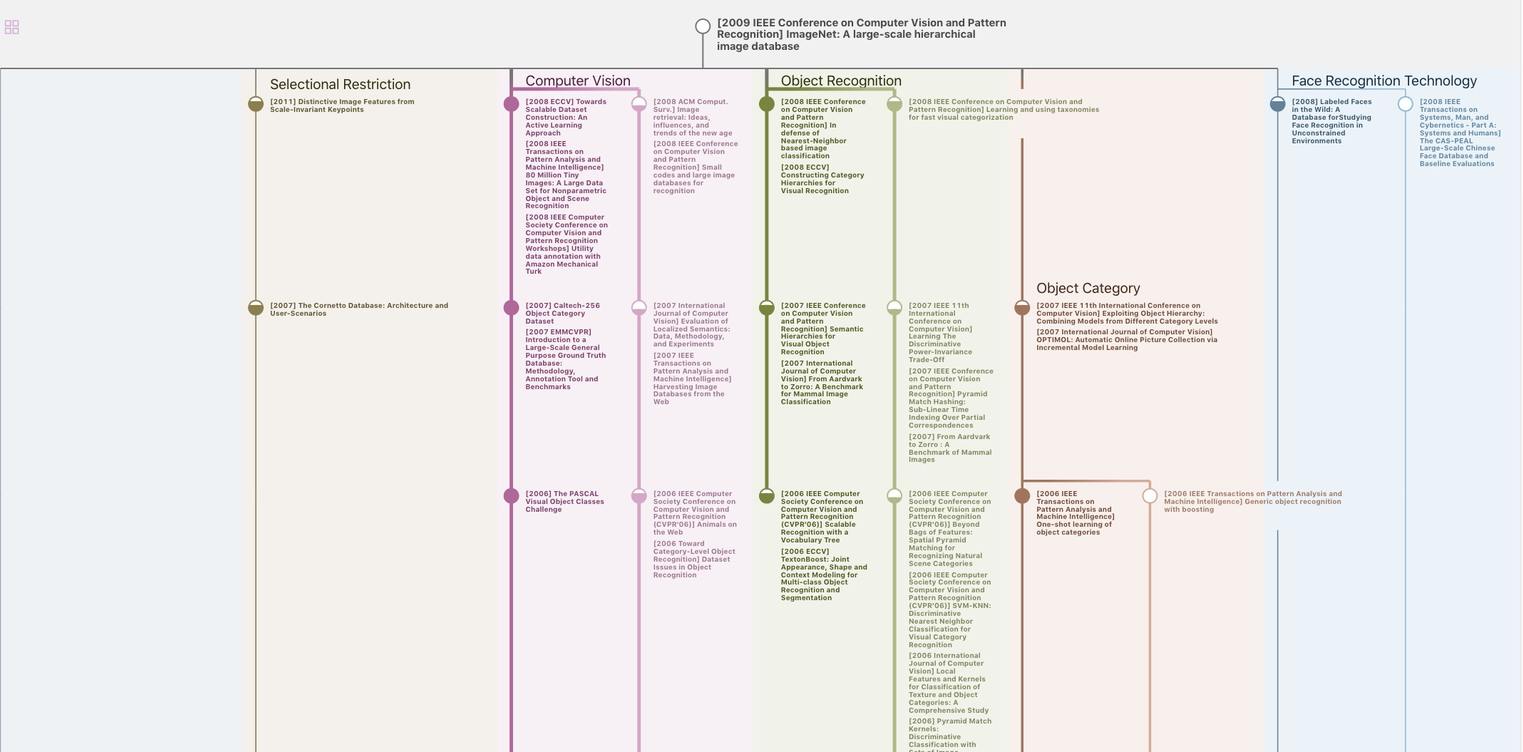
生成溯源树,研究论文发展脉络
Chat Paper
正在生成论文摘要