Emerging Low Dimensional Material Devices for Beyond von-Neumann Computing
2019 Device Research Conference (DRC)(2019)
摘要
Conventional computation hardware based on von Neumann architecture is constrained by the shared bus between data memory and instruction memory, which limits the computation performance and increase energy consumption especially for tasks requiring massive parallel operations. Emerging computation architectures such as neuromorphic electronic systems that can simultaneously process data and instructions efficiently are promising for addressing such issues. Low dimensional materials including emerging classes of 2D and 1D materials offer rich physical properties unfound in conventional semiconductor materials that are particularly attractive for exploring conceptually new electronic devices for many non-von-Neumann electronic systems. In this talk, we will discuss our recent work in developing low dimensional material electronic devices including atomically-thin ultralow power filamentary memristive devices with record sub-femtojoule energy consumption; device concepts with new functionalities including re-configurability, metaplasticity and connetion heterogeneity; and stochastic memristive devices for applications in combinatorial optimization. These devices may contribute to key building blocks for the low power hardware implementation of many emerging computing schemes.
更多查看译文
关键词
massive parallel operations,computation architectures,neuromorphic electronic systems,semiconductor materials,nonvon-Neumann electronic systems,stochastic memristive devices,low power hardware implementation,von-Neumann computing,computation hardware,von Neumann architecture,data memory,instruction memory,sub-femtojoule energy consumption,low dimensional material electronic devices,physical properties,atomically-thin ultralow power filamentary memristive devices,combinatorial optimization
AI 理解论文
溯源树
样例
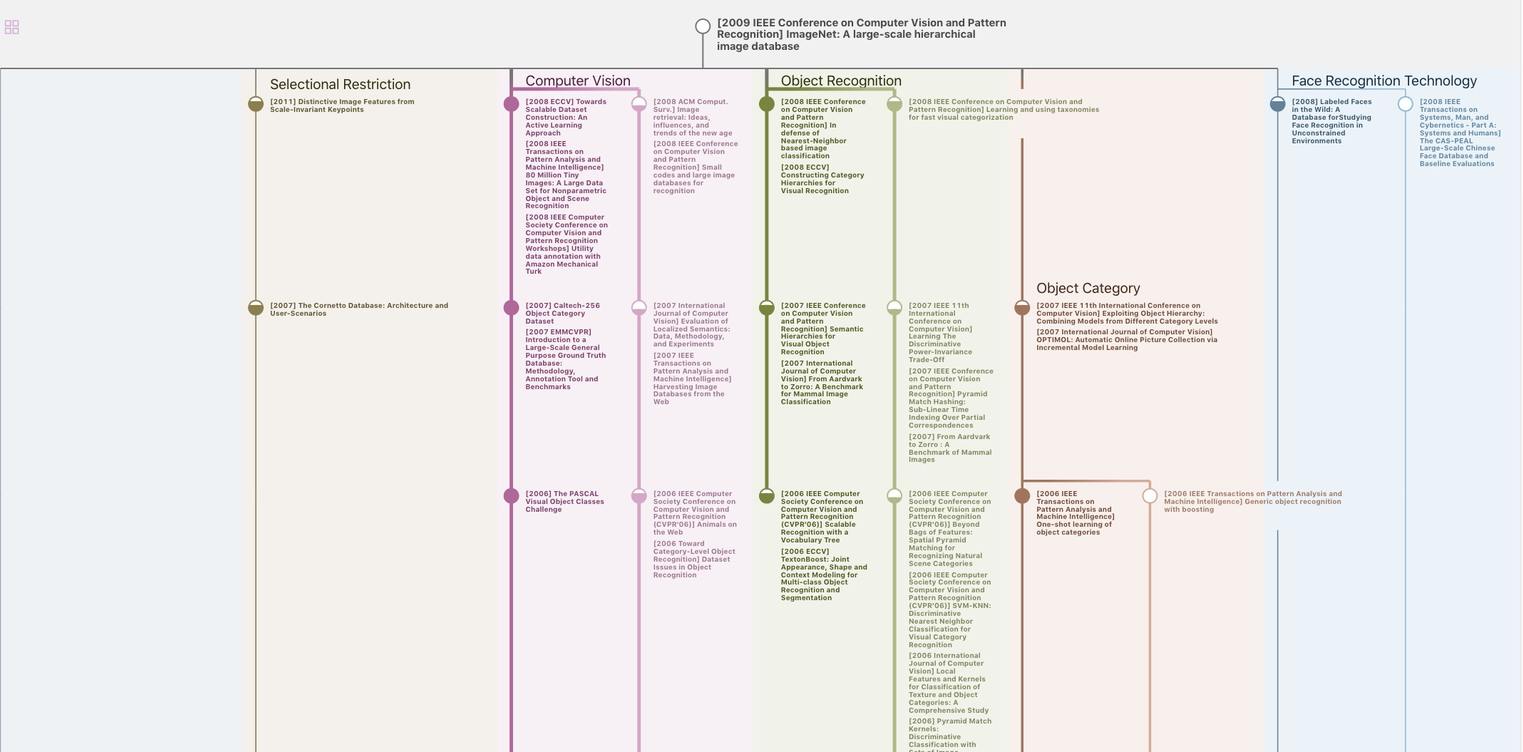
生成溯源树,研究论文发展脉络
Chat Paper
正在生成论文摘要