Dynamic $(\mathrm{Bi}_{\mathrm{x}}\mathrm{Sb}_{1-\mathrm{x}})_{2}\mathrm{Te}_{\mathrm{3}}$ Synaptic Devices with Programmable Spatio-Temporal Responses
2019 Device Research Conference (DRC)(2019)
摘要
Neuromorphic computing has emerged as a new computing paradigm to tackle the von Neumann bottleneck and enable a more energy-efficient processing of today's large-scale datasets, especially for data-intensive applications such as image and pattern recognition, speech recognition, and autonomous machines
1
. Despite the staggering success of deep neural networks (DNNs), their need for labelled data and the energy intensive training process are limiting their widespread usage. Recently, spiking neural networks (SNNs), which resembles more closely to biological neural networks via their coupled spatio-temporal dynamics, have attracted intense research interests
1,2
in hope of mimicking the superb energy efficiency of the human brain, which exceeds the petaflop mark in performance with a power consumption as low as 20 W.
更多查看译文
关键词
coupled spatio-temporal dynamics,programmable spatio-temporal responses,neuromorphic computing,von Neumann bottleneck,energy-efficient processing,large-scale datasets,data-intensive applications,pattern recognition,speech recognition,autonomous machines,deep neural networks,DNNs,labelled data,energy intensive training process,biological neural networks,dynamic synaptic devices,image recognition,spiking neural networks,SNNs,human brain,power consumption,(BixSb1-x)2Te3
AI 理解论文
溯源树
样例
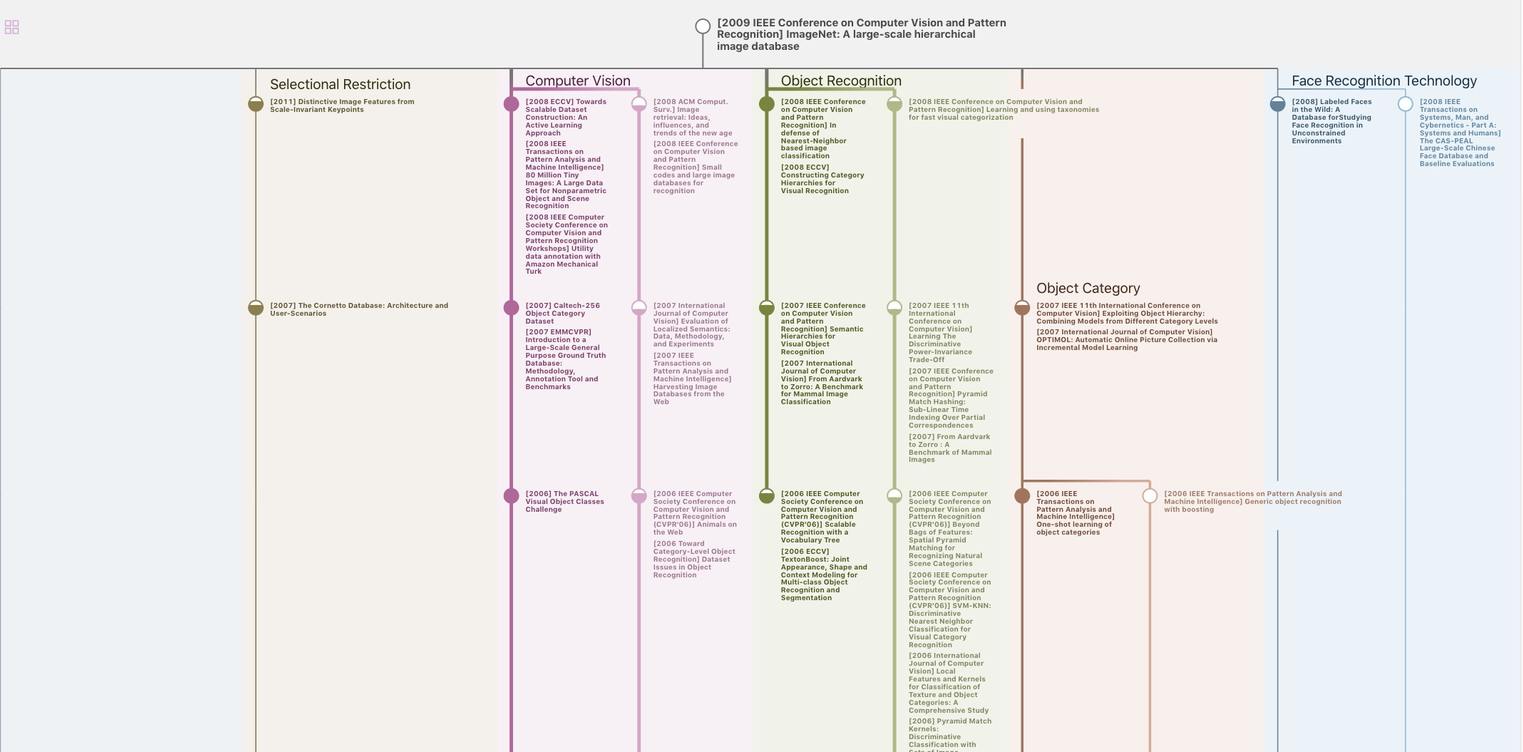
生成溯源树,研究论文发展脉络
Chat Paper
正在生成论文摘要