Event-Triggered Distributed Inference
CDC(2020)
摘要
We study a setting where each agent in a network receives certain private signals generated by an unknown static state that belongs to a finite set of hypotheses. The agents are tasked with collectively identifying the true state. To solve this problem in a communication-efficient manner, we propose an event-triggered distributed learning algorithm that is based on the principle of diffusing low beliefs on each false hypothesis. Building on this principle, we design a trigger condition under which an agent broadcasts only those components of its belief vector that have adequate innovation, to only those neighbors that require such information. We establish that under standard assumptions, each agent learns the true state exponentially fast almost surely. We also identify sparse communication regimes where the inter-communication intervals grow unbounded, and yet, the asymptotic learning rate of our algorithm remains the same as the best known rate for this problem. We then establish, both in theory and via simulations, that our event-triggering strategy has the potential to significantly reduce information flow from uninformative agents to informative agents. Finally, we argue that, as far as only asymptotic learning is concerned, one can allow for arbitrarily sparse communication patterns.
更多查看译文
关键词
inference,private signals,unknown static state,false hypothesis,trigger condition,agent broadcasts,belief vector,adequate innovation,sparse communication regimes,intercommunication intervals,asymptotic learning rate,event-triggering strategy,uninformative agents,informative agents,arbitrarily sparse communication patterns
AI 理解论文
溯源树
样例
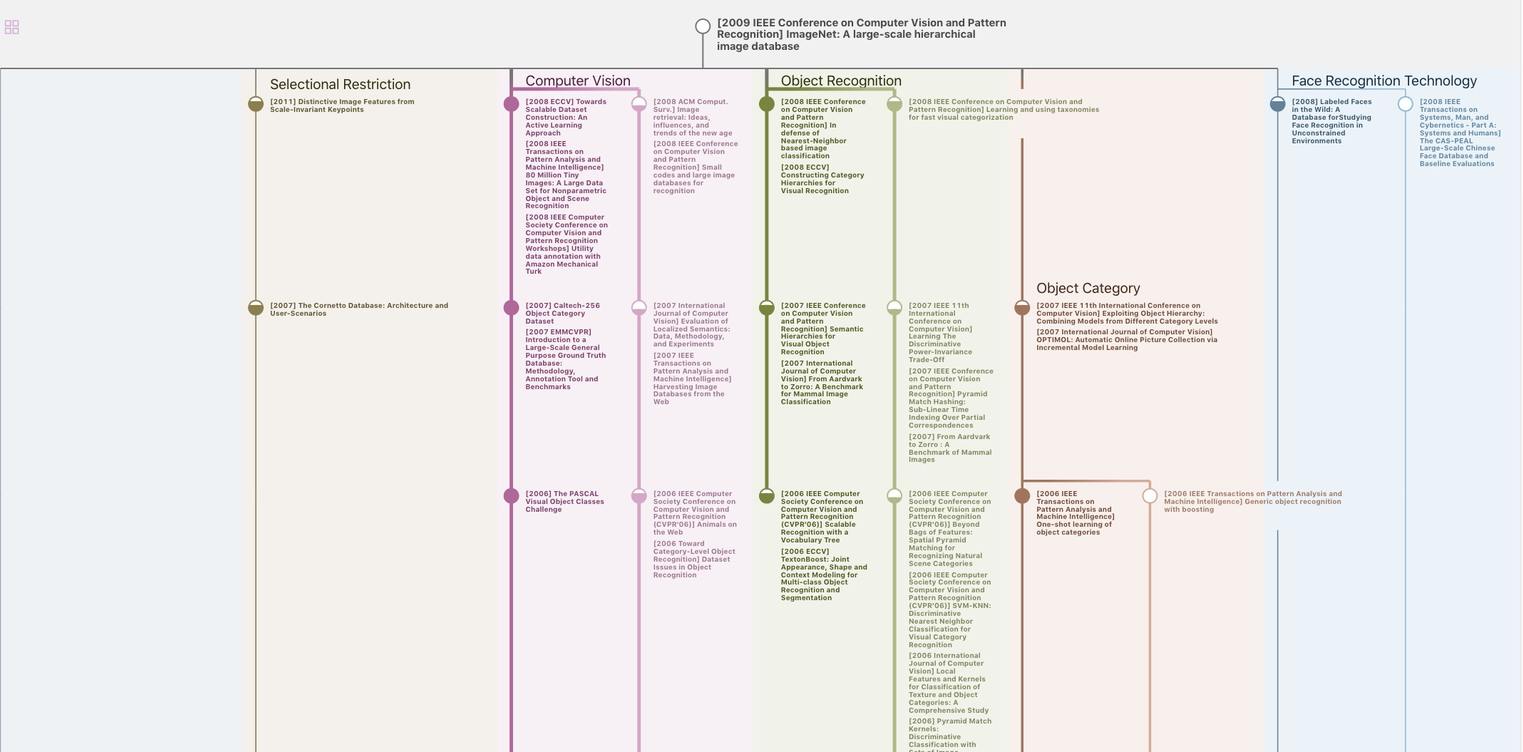
生成溯源树,研究论文发展脉络
Chat Paper
正在生成论文摘要