Hierarchical Bayesian Nearest Neighbor Co-Kriging Gaussian Process Models; An Application To Intersatellite Calibration
SPATIAL STATISTICS(2021)
摘要
Recent advancements in remote sensing technology and the increasing size of satellite constellations allow for massive geo-physical information to be gathered daily on a global scale by numerous platforms of different fidelity. The auto-regressive co-kriging model provides a suitable framework for the analysis of such data sets as it is able to account for cross-dependencies among different fidelity satellite outputs. However, its imple-mentation in multifidelity large spatial data sets is practically infeasible because the computational complexity increases cu-bically with the total number of observations. In this paper, we propose a nearest neighbor co-kriging Gaussian process (GP) that couples the auto-regressive model and nearest neighbor GP by using augmentation ideas. Our model reduces the com-putational complexity to be linear with the total number of spatially observed locations. The spatial random effects of the nearest neighbor GP are augmented in a manner which allows the specification of semi-conjugate priors. This facilitates the design of an efficient MCMC sampler involving mostly direct sampling updates. The good predictive performance of the pro-posed method is demonstrated in a simulation study. We use the proposed method to analyze High-resolution Infrared Radiation Sounder data gathered from two NOAA polar orbiting satellites. (C) 2021 Elsevier B.V. All rights reserved.
更多查看译文
关键词
Augmented hierarchically nested design, Autoregressive co-kriging, Nearest neighbor Gaussian process, Remote sensing
AI 理解论文
溯源树
样例
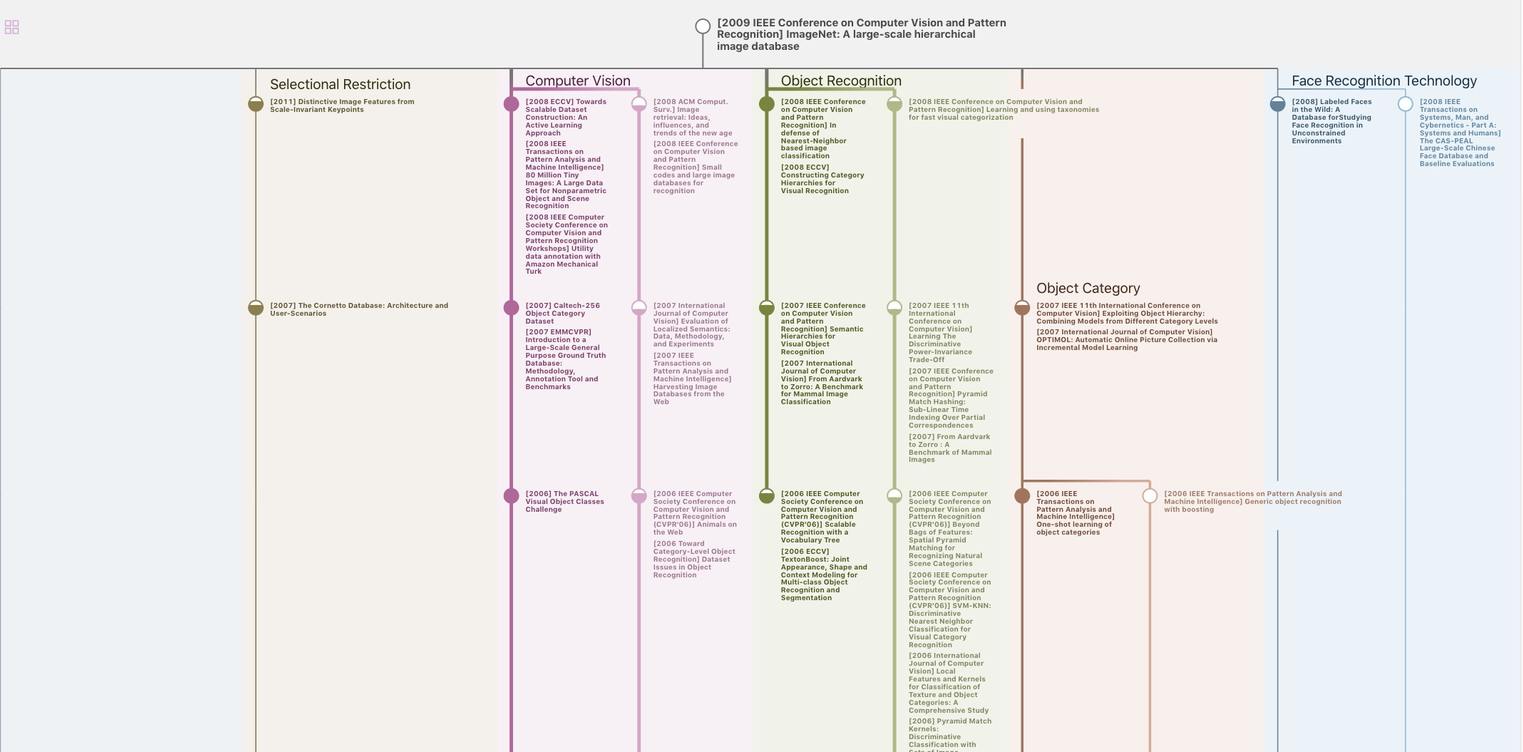
生成溯源树,研究论文发展脉络
Chat Paper
正在生成论文摘要