Benchmarking Deep Spiking Neural Networks on Neuromorphic Hardware.
ICANN (2)(2020)
摘要
With more and more event-based neuromorphic hardware systems being developed at universities and in industry, there is a growing need for assessing their performance with domain specific measures. In this work, we use the methodology of converting pre-trained non-spiking to spiking neural networks to evaluate the performance loss and measure the energy-per-inference for three neuromorphic hardware systems (BrainScaleS, Spikey, SpiNNaker) and common simulation frameworks for CPU (NEST) and CPU/GPU (GeNN). For analog hardware we further apply a re-training technique known as hardware-in-the-loop training to cope with device mismatch. This analysis is performed for five different networks, including three networks that have been found by an automated optimization with a neural architecture search framework. We demonstrate that the conversion loss is usually below one percent for digital implementations, and moderately higher for analog systems with the benefit of much lower energy-per-inference costs.
更多查看译文
关键词
Spiking neural networks, Neural architecture search, Benchmark
AI 理解论文
溯源树
样例
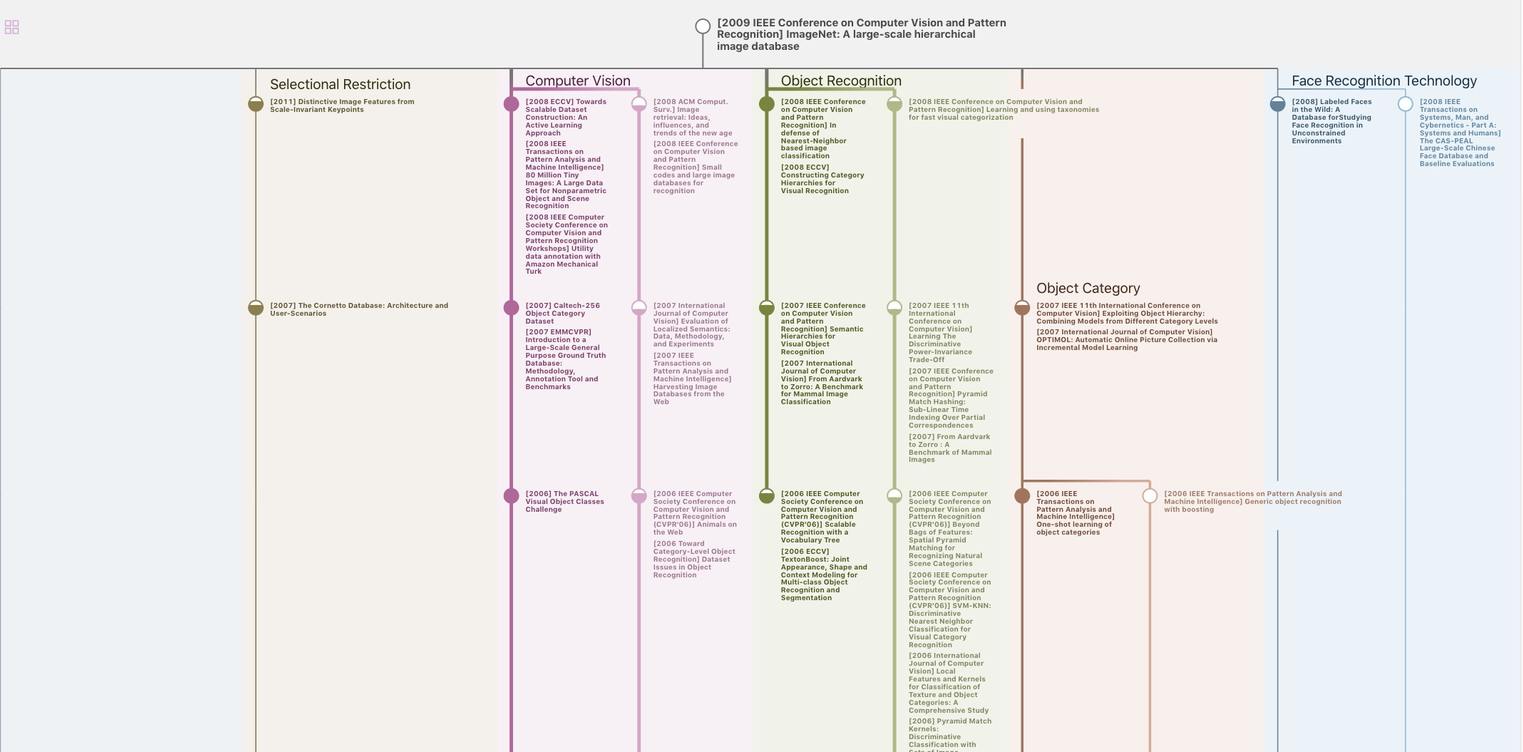
生成溯源树,研究论文发展脉络
Chat Paper
正在生成论文摘要