PointGroup: Dual-Set Point Grouping for 3D Instance Segmentation
2020 IEEE/CVF CONFERENCE ON COMPUTER VISION AND PATTERN RECOGNITION (CVPR)(2020)
摘要
Instance segmentation is an important task for scene understanding. Compared to the fully-developed 2D, 3D instance segmentation for point clouds have much room to improve. In this paper, we present PointGroup, a new end-to-end bottom-up architecture, specifically focused on better grouping the points by exploring the void space between objects. We design a two-branch network to extract point features and predict semantic labels and offsets, for shifting each point towards its respective instance centroid. A clustering component is followed to utilize both the original and offset-shifted point coordinate sets, taking advantage of their complementary strength. Further, we formulate the ScoreNet to evaluate the candidate instances, followed by the Non-Maximum Suppression (NMS) to remove duplicates. We conduct extensive experiments on two challenging datasets, ScanNet v2 and S3DIS, on which our method achieves the highest performance, 63.6 former best solutions in terms of mAP with IoU threshold 0.5.
更多查看译文
关键词
3D instance segmentation,point clouds,PointGroup,semantic labels,S3DIS,dual-set point grouping,instance centroid,non-maximum suppression
AI 理解论文
溯源树
样例
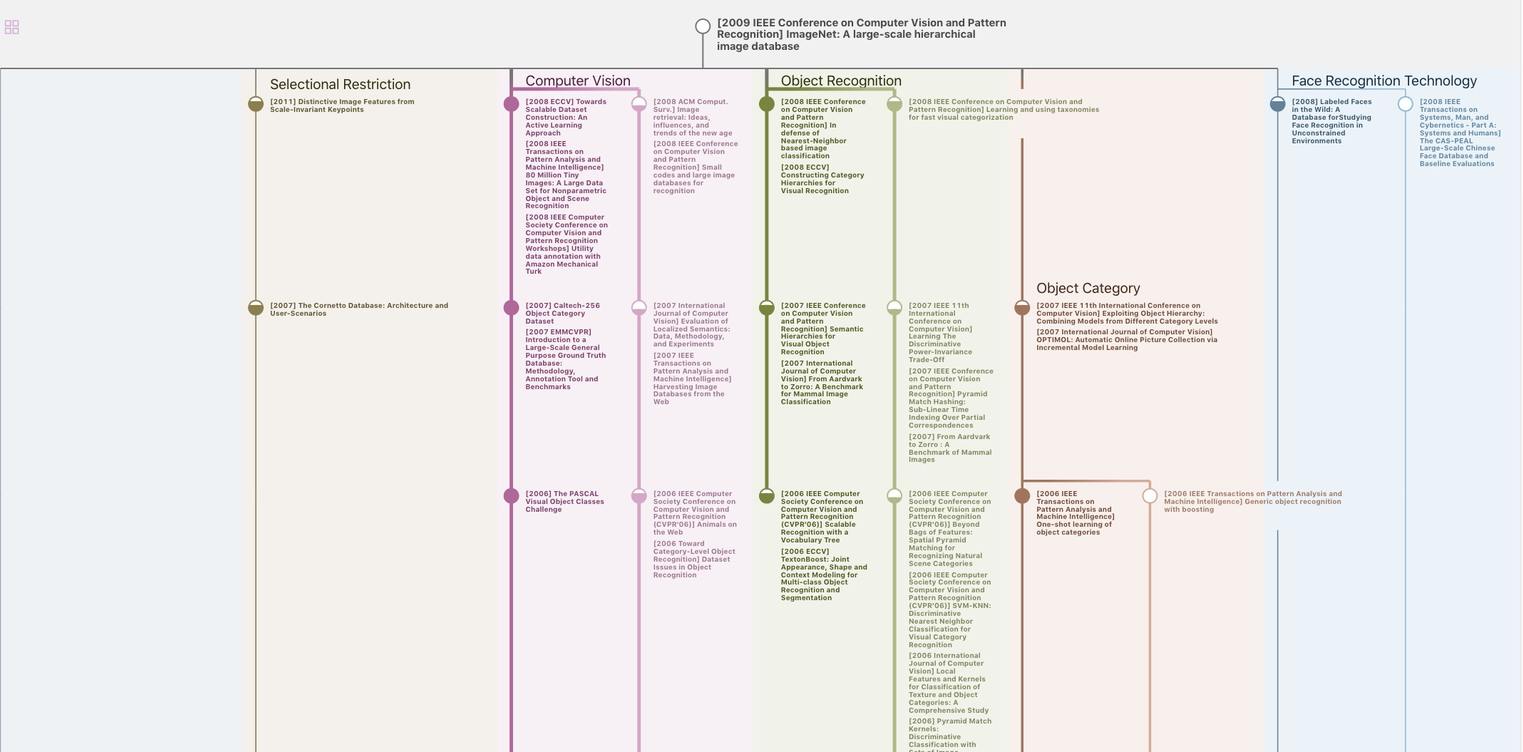
生成溯源树,研究论文发展脉络
Chat Paper
正在生成论文摘要