Effective L-Diversity Anonymization Algorithm Based On Improved Clustering
CYBERSPACE SAFETY AND SECURITY, PT II(2019)
摘要
Mass data has been collected and released everyday, at the same time, the published data contains a lot of sensitive information related to individuals. K-anonymity privacy preserving mechanisms can prevent the disclosure of individual privacy information in the scenarios of data publication. L-diversity further considers the distribution of sensitive attributes in equivalence classes to avoid homogeneity attacks. In this paper, we propose an improved L-diversity algorithm based on clustering, and we consider the L-diversity demand of sensitive attributes while clustering to achieve K-anonymity. We minimize the total information loss of each equivalence class by choosing records which has minimal loss of information, regardless of whether they have different sensitive attributes, until the number of distinct values of sensitive attribute in the equivalence class reaches L. This algorithm we conduct experiments on UCI Adult data set and compared with traditional (K,L)-member algorithm. Theoretical analysis and the experimental results demonstrate that the improved L-diversity algorithm can not only improve the privacy protection degree of sensitive data, but also effectively reduce the information loss.
更多查看译文
关键词
Data anonymization, L-diversity, K-anonymity, Clustering
AI 理解论文
溯源树
样例
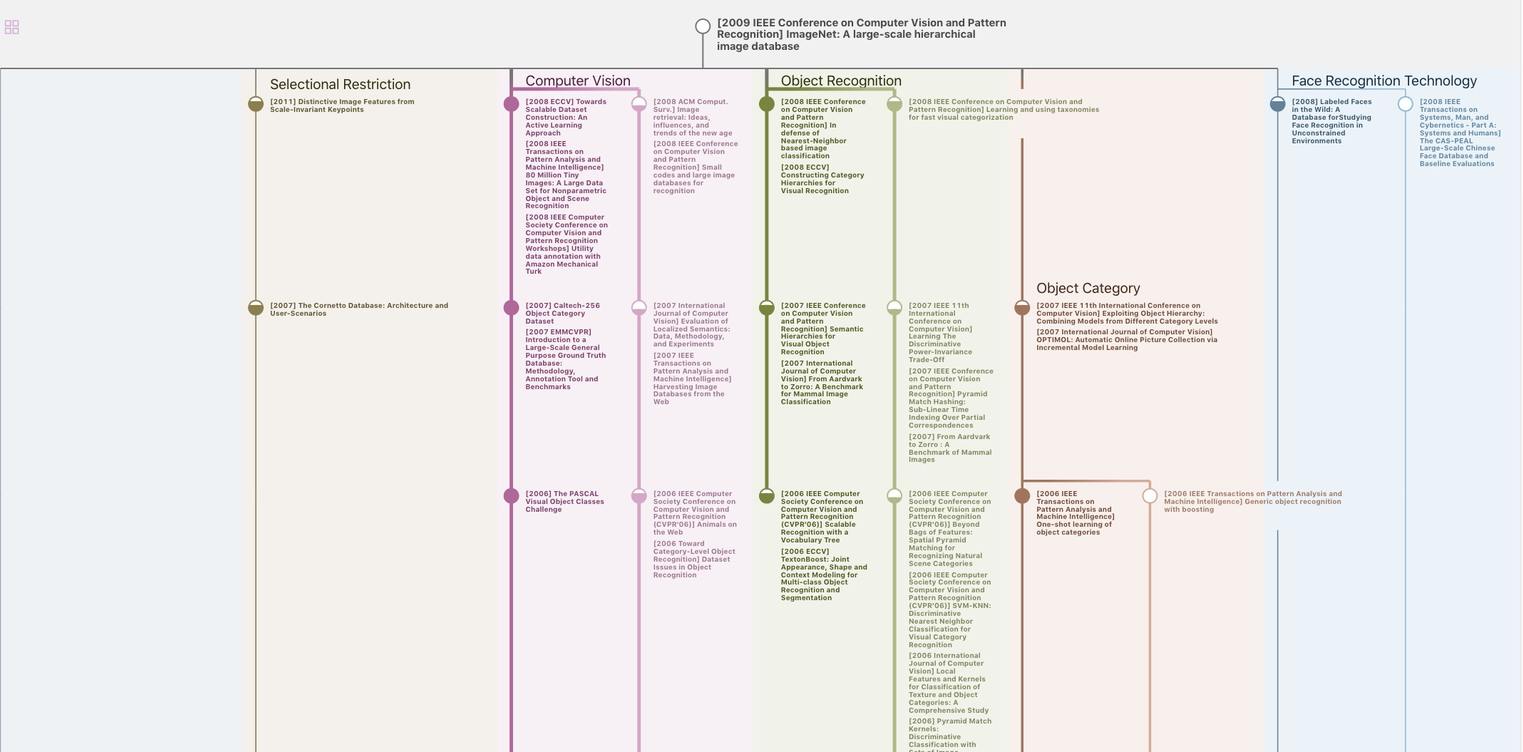
生成溯源树,研究论文发展脉络
Chat Paper
正在生成论文摘要