PrivBUD-Wise: Differentially Private Frequent Itemsets Mining in High-Dimensional Databases.
APWeb/WAIM (1)(2019)
摘要
In this paper, we study the problem of mining frequent itemsets in high-dimensional databases with differential privacy, and propose a novel algorithm, PrivBUD-Wise , which achieves high result utility as well as a high privacy level. Instead of limiting the cardinality of transactions by truncating or splitting approaches, which causes extra information loss and result in unsatisfactory performance in utility, PrivBUD-Wise doesn’t make any preprocessing on original database and guarantees high result utility by reducing extra consumption on irrelevant itemsets as much as possible. To achieve that, we first propose a Report Noisy mechanism with optional number of reported itemsets: SRNM, and what is more important is that we give a strict proof for SRNM in the appendix. Moreover, PrivBUD-Wise first proposes a biased allocation strategy and no assumption or estimation on the maximal cardinality needs to be made. The good performance in utility and efficiency of PrivBUD-Wise is shown by experiments on three real-world datasets.
更多查看译文
关键词
Frequent itemsets mining, Differential privacy, Smart Report Noisy Max (SRNM), Privacy budget allocations
AI 理解论文
溯源树
样例
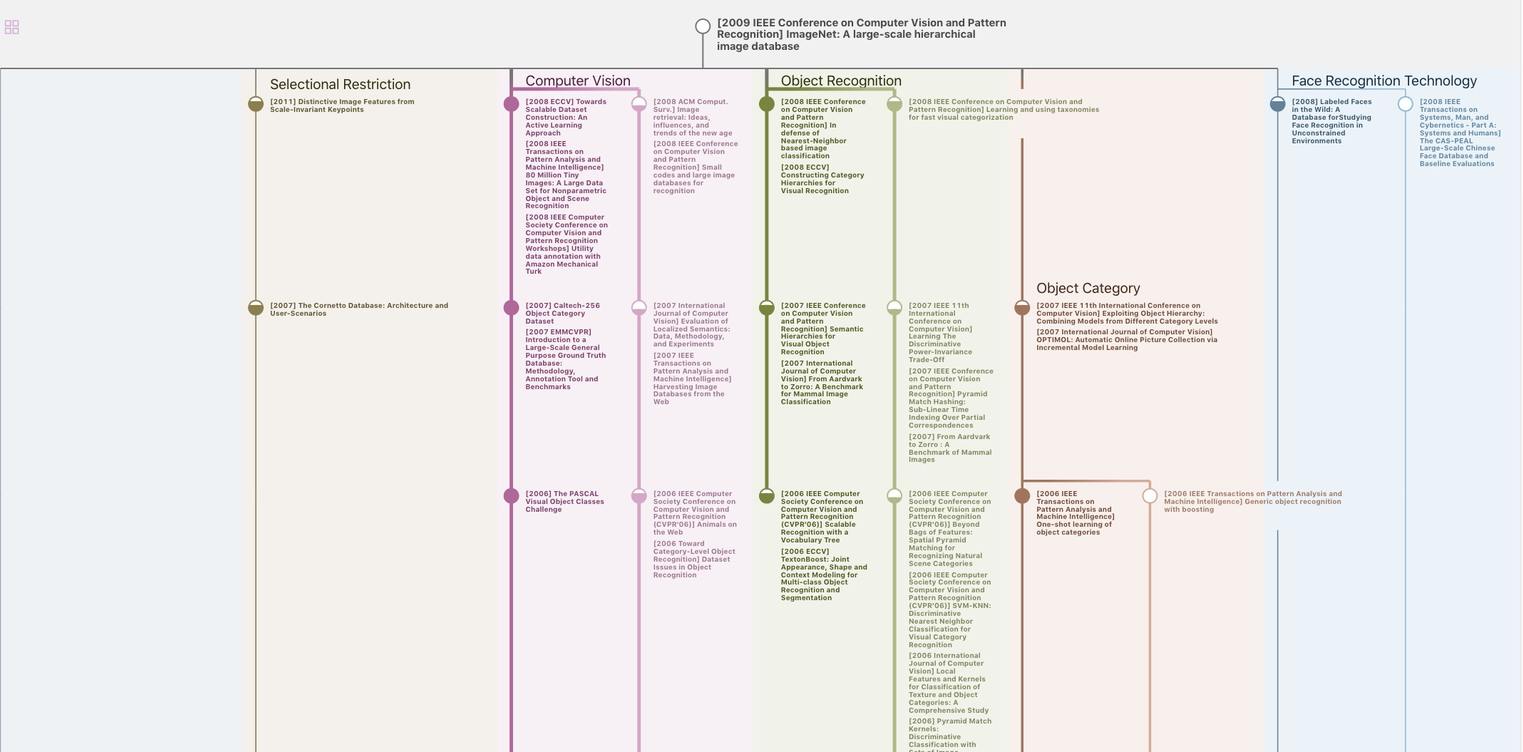
生成溯源树,研究论文发展脉络
Chat Paper
正在生成论文摘要