Spread Sampling and Its Applications on Graphs.
COMPLEX NETWORKS (1)(2019)
摘要
Efficiently finding small samples with high diversity from large graphs has many practical applications such as community detection and online survey. This paper proposes a novel scalable node sampling algorithm for large graphs that can achieve better spread or diversity across communities intrinsic to the graph without requiring any costly pre-processing steps. The proposed method leverages a simple iterative sampling technique controlled by two parameters: infection rate, that controls the dynamics of the procedure and removal threshold that affects the end-of-procedure sampling size. We demonstrate that our method achieves very high community diversity with an extremely low sampling budget on both synthetic and real-world graphs, with either balanced or imbalanced communities. Additionally, we leverage the proposed technique for a very low sampling budget (only 2%) driven treatment assignment in Network A/B Testing scenario, and demonstrate competitive performance concerning baseline on both synthetic and real-world graphs.
更多查看译文
关键词
Graph sampling, Social network analysis
AI 理解论文
溯源树
样例
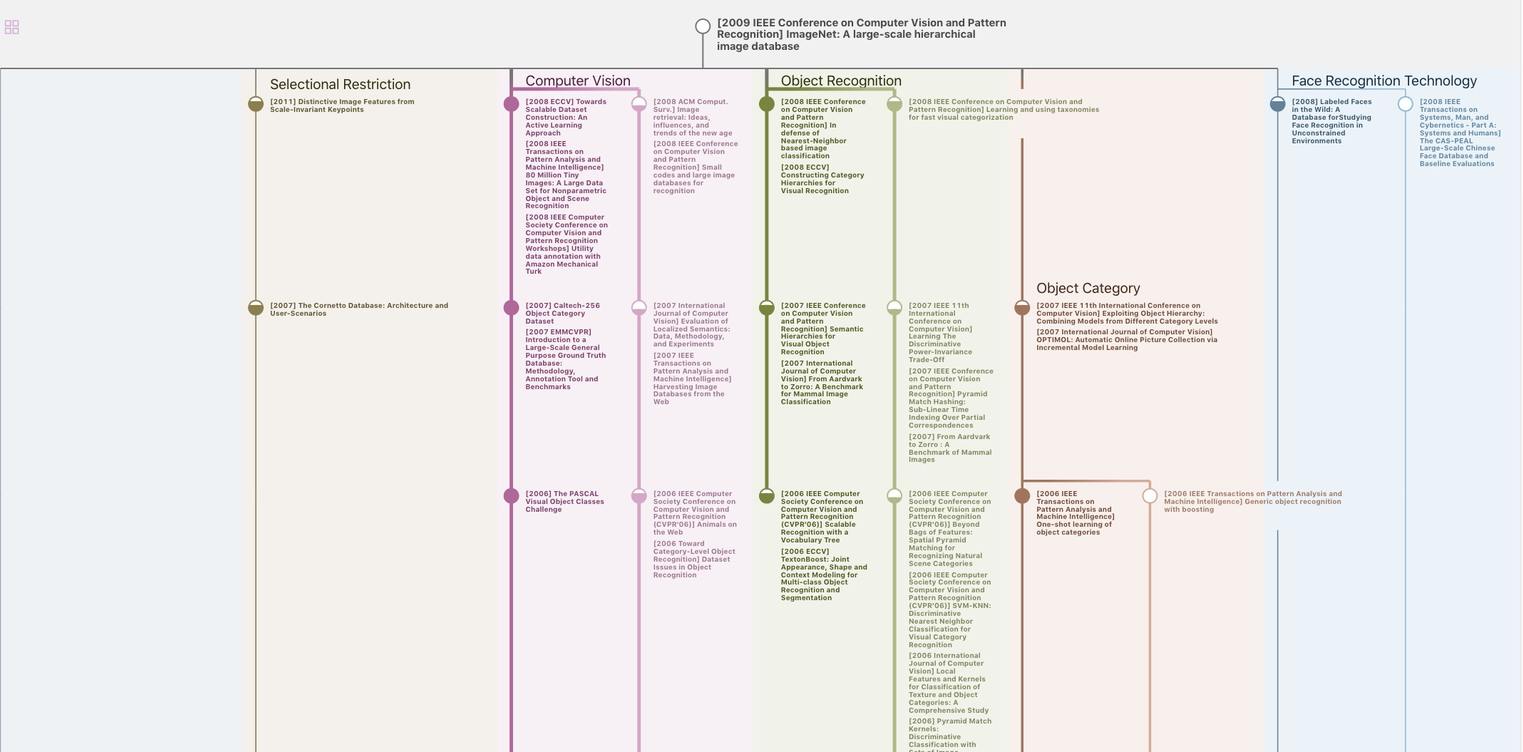
生成溯源树,研究论文发展脉络
Chat Paper
正在生成论文摘要