Implications Tutorial: Assessing The Intersection Of Organizational Structure And Fat* Efforts Within Industry
FAT* '20: PROCEEDINGS OF THE 2020 CONFERENCE ON FAIRNESS, ACCOUNTABILITY, AND TRANSPARENCY(2020)
摘要
The work within the Fairness, Accountability, and Transparency of ML (fair-ML) community will positively benefit from appreciating the role of organizational culture and structure in the effective practice of fair-ML efforts of individuals, teams, and initiatives within industry. In this tutorial session we will explore various organizational structures and possible leverage points to effectively intervene in the process of design, development, and deployment of AI systems, towards contributing to positive fair-ML outcomes. We will begin by presenting the results of interviews conducted during an ethnographic study among practitioners working in industry, including themes related to: origination and evolution, common challenges, ethical tensions, and effective enablers. The study was designed through the lens of Industrial Organizational Psychology and aims to create a mapping of the current state of the fair-ML organizational structures inside major AI companies. We also look at the most-desired future state to enable effective work to increase algorithmic accountability, as well as the key elements in the transition from the current to that future state. We investigate drivers for change as well as the tensions between creating an 'ethical' system vs one that is 'ethical' enough. After presenting our preliminary findings, the rest of the tutorial will be highly interactive. Starting with a facilitated activity in break out groups, we will discuss the already identified challenges, best practices, and mitigation strategies. Finally, we hope to create space for productive discussion among AI practitioners in industry, academic researchers within various fields working directly on algorithmic accountability and transparency, advocates for various communities most impacted by technology, and others. Based on the interactive component of the tutorial, facilitators and interested participants will collaborate on further developing the discussed challenges into scenarios and guidelines that will be published as a follow up report.
更多查看译文
关键词
organizational structure, I/O psychology, empirical study, fair machine learning, need-finding
AI 理解论文
溯源树
样例
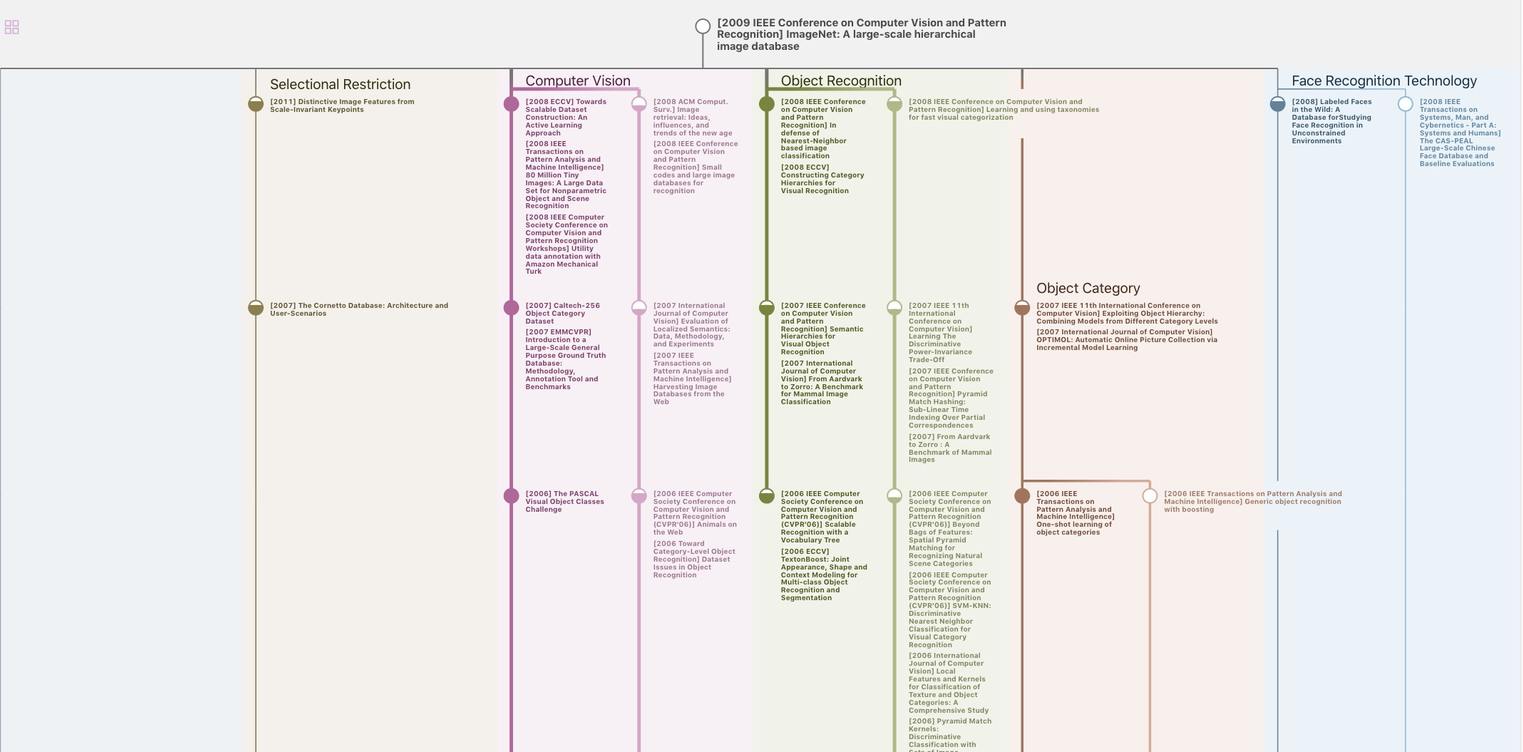
生成溯源树,研究论文发展脉络
Chat Paper
正在生成论文摘要