A Computer-Aided Hybrid Framework for Early Diagnosis of Breast Cancer.
ACSS (2)(2018)
摘要
We have presented here a novel framework for the early diagnosis of breast cancer. The framework is comprised of two major phases. Firstly, the potential suspicious regions are automatically segmented from the breast thermograms. In the second phase, the segmented suspicious regions are diagnostically classified into benign and malignant cases. For the automatic segmentation of the suspicious regions, a new region-based level-set method named GRL-LSM has been proposed. Initially, the potential suspicious regions are estimated by the proposed adaptive thresholding method (ATM), named GRL. Then, a region-based level set method (LSM) is employed to precisely segment the potentially suspicious regions. As initialization plays a vital role in a region-based LSM, so we have proposed a new automatic initialization technique based on the outcome of our adaptive thresholding method. Moreover, a stopping criterion is proposed to stop the LSM. After the segmentation phase, some higher-order statistical and GLCM-based texture features are extracted and fed into a three-layered feed-forward artificial neural network for classifying the breast thermograms. Fifty breast thermograms with confirmed hot spots are randomly chosen from the DMR-IR database for the experimental purpose. Experimental evaluation shows that our proposed framework can differentiate between malignant and benign breasts with an accuracy of 89.4%, the sensitivity of 86%, and specificity of 90%. Additionally, our segmentation results are validated quantitatively and qualitatively with the respective breast thermograms which were manually delineated by two experts and also with some classical segmentation methods.
更多查看译文
关键词
breast cancer,early diagnosis,hybrid,computer-aided
AI 理解论文
溯源树
样例
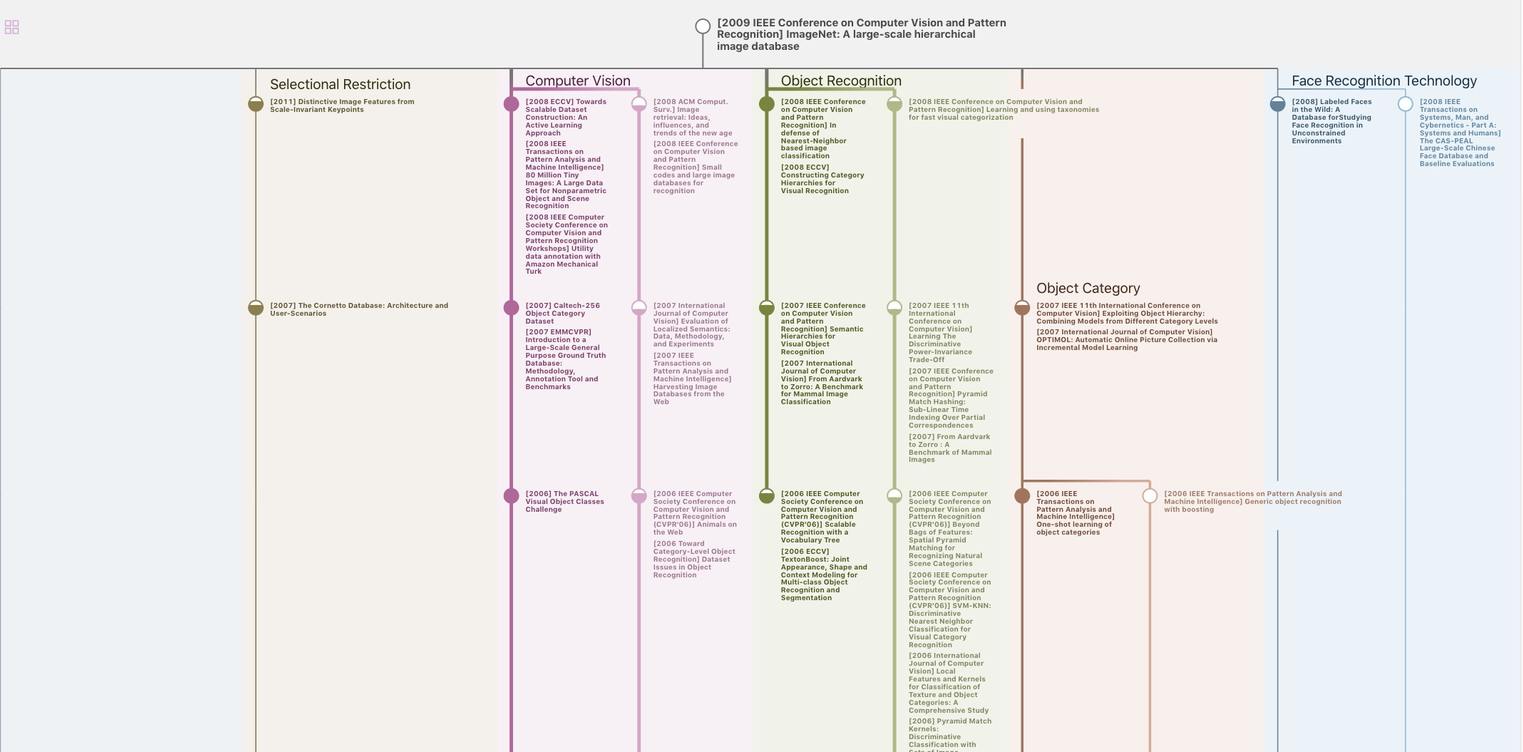
生成溯源树,研究论文发展脉络
Chat Paper
正在生成论文摘要