Uncertainty Estimation for Black-Box Classification Models: A Use Case for Sentiment Analysis.
IbPRIA (1)(2019)
摘要
With the advent of new pre-trained word embedding models like ELMO, GPT or BERT, that leverage transfer-learning to deliver high-quality prediction systems, natural language processing (NLP) methods are reaching or even overtaking human baselines in some applications. The basic principle of these successful models is to train a model to solve a given NLP task, mainly Language Modelling, using significant volumes of data like the whole Wikipedia. The model is then fine-tuned to solve another NLP task, requiring fewer domain-specific data to achieve state-of-the-art accuracies. The method proposed in the present work assists the practitioner in evaluating the quality of the transferred classification models when applied to new data domains. In this case, we consider the original model as a black box. No matter how complex the original model may be, the method only requires access to the output layer to train a measure of the uncertainty associated with the predictions of the original model. This measure of uncertainty is a measure of how well the black-box model accommodates to the new data. Later on, we show how a rejection system can use this uncertainty to improve its accuracy, effectively enabling the practitioner to find the best trade-off between the quality of the model and the number of rejected cases.
更多查看译文
关键词
Sentiment analysis, Transfer learning, Uncertainty estimation, Natural Language Processing
AI 理解论文
溯源树
样例
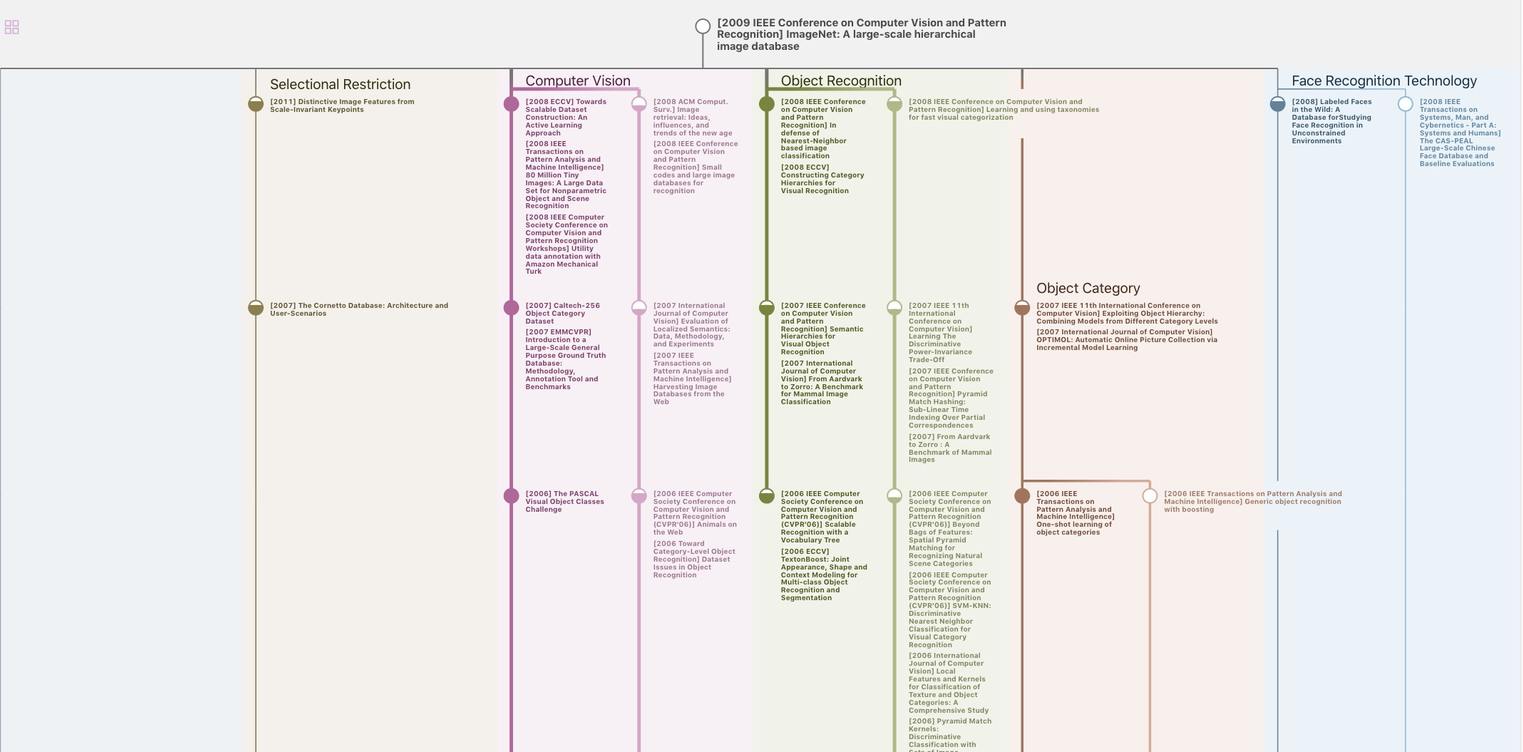
生成溯源树,研究论文发展脉络
Chat Paper
正在生成论文摘要