Low-Overhead Compressibility Prediction for High-Performance Lossless Data Compression
IEEE access(2020)
摘要
As big data has evolved over the past few years, a lack of storage space and I/O bandwidth has become one of the most important challenges to overcome. To mitigate these problems, data compression schemes reduce the amount of data to be stored and transmitted at the cost of additional CPU overhead. Many researchers have attempted to reduce the computational load imposed on the CPU by data compression using specialized hardware. However, space savings through data compression often comes from only a small portion of data. Therefore, compressing all data, regardless of data compressibility, can waste computational resources. Our work aims to decrease the cost of data compression by introducing a selective data compression scheme based on data compressibility prediction. The proposed compressibility prediction method provides more fine-grained selectivity for combinational compression. Additionally, our method reduces the amount of resources consumed by the compressibility predictor, enabling selective compression at a low cost. To verify the proposed scheme, we implemented a DEFLATE compression system on a field-programmable gate array platform. Experimental results demonstrate that the proposed scheme improves compression throughput by 34.15% with a negligible decrease in compression ratio.
更多查看译文
关键词
Data compression,Huffman coding,LZ77 encoding,accelerator architecture,field programmable gate array,estimation,compressibility
AI 理解论文
溯源树
样例
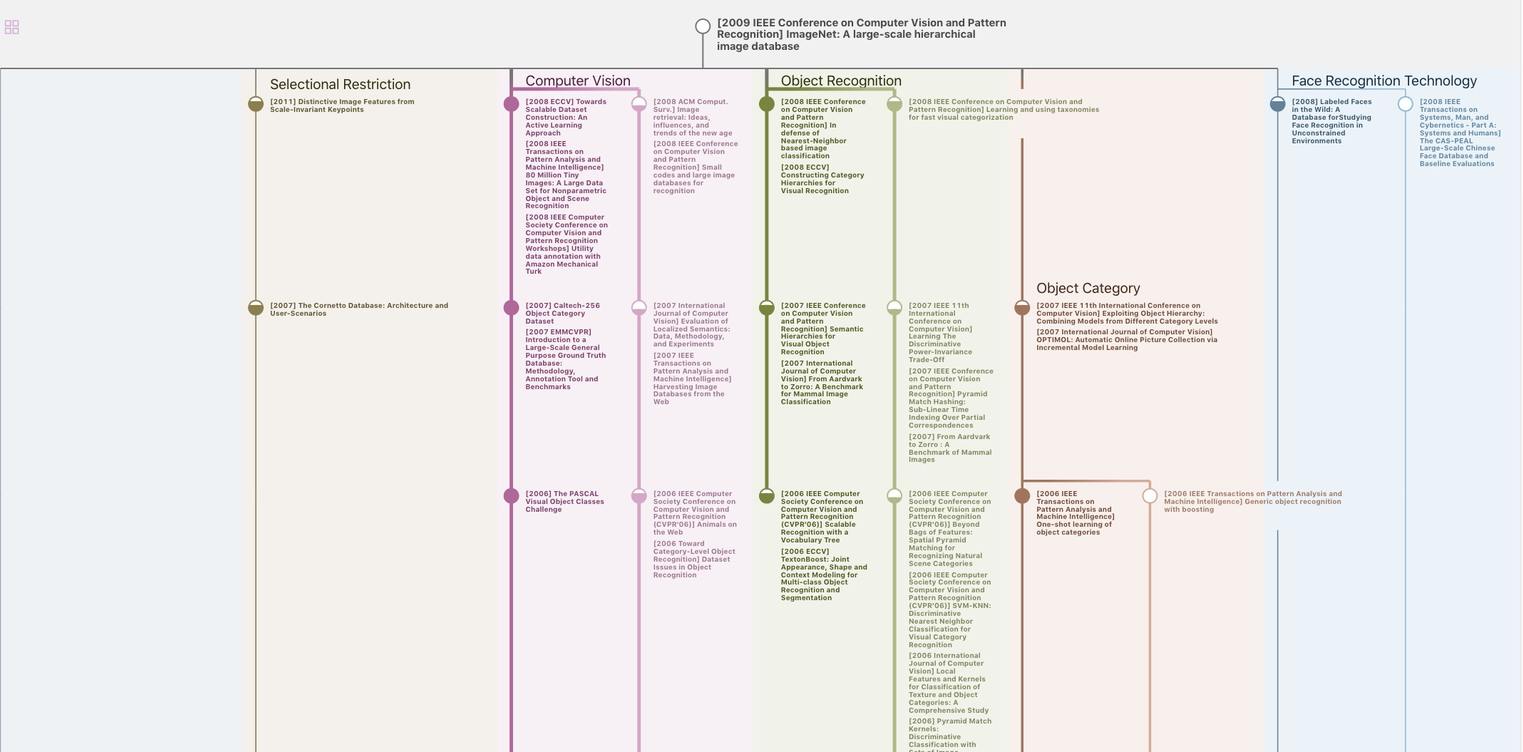
生成溯源树,研究论文发展脉络
Chat Paper
正在生成论文摘要