The Balanced Loss Curriculum Learning
IEEE ACCESS(2020)
摘要
Curriculum Learning (CL) selects the training samples from the easy to difficult to boost the classification results. Most existing variates of CL measure the difficulty level of an example in an intuitive way, i.e., the loss value between the prediction and the ground truth. This way ignores the different distances to every class boundary, which is implied in the prediction vectors. In this paper, we propose a novel CL framework, named Balance Loss Curriculum Learning(BLCL), to reveal the comprehensive difficulty level of an example and improve the curriculum process based on the deep architectures. We follow the teacher-student learning module and train the teacher & student networks simultaneously. The predictions learned by the teacher network indicates the boundary information and is set as the difficulty measurement to guide the student network. The student network focuses on the easy samples according to the boundary information by decreasing the loss from difficult examples as well as increasing the loss from easy examples. Through extensive and rigor experiments, we demonstrate the effectiveness and generality of our framework.
更多查看译文
关键词
Classification, curriculum learning, deep learning
AI 理解论文
溯源树
样例
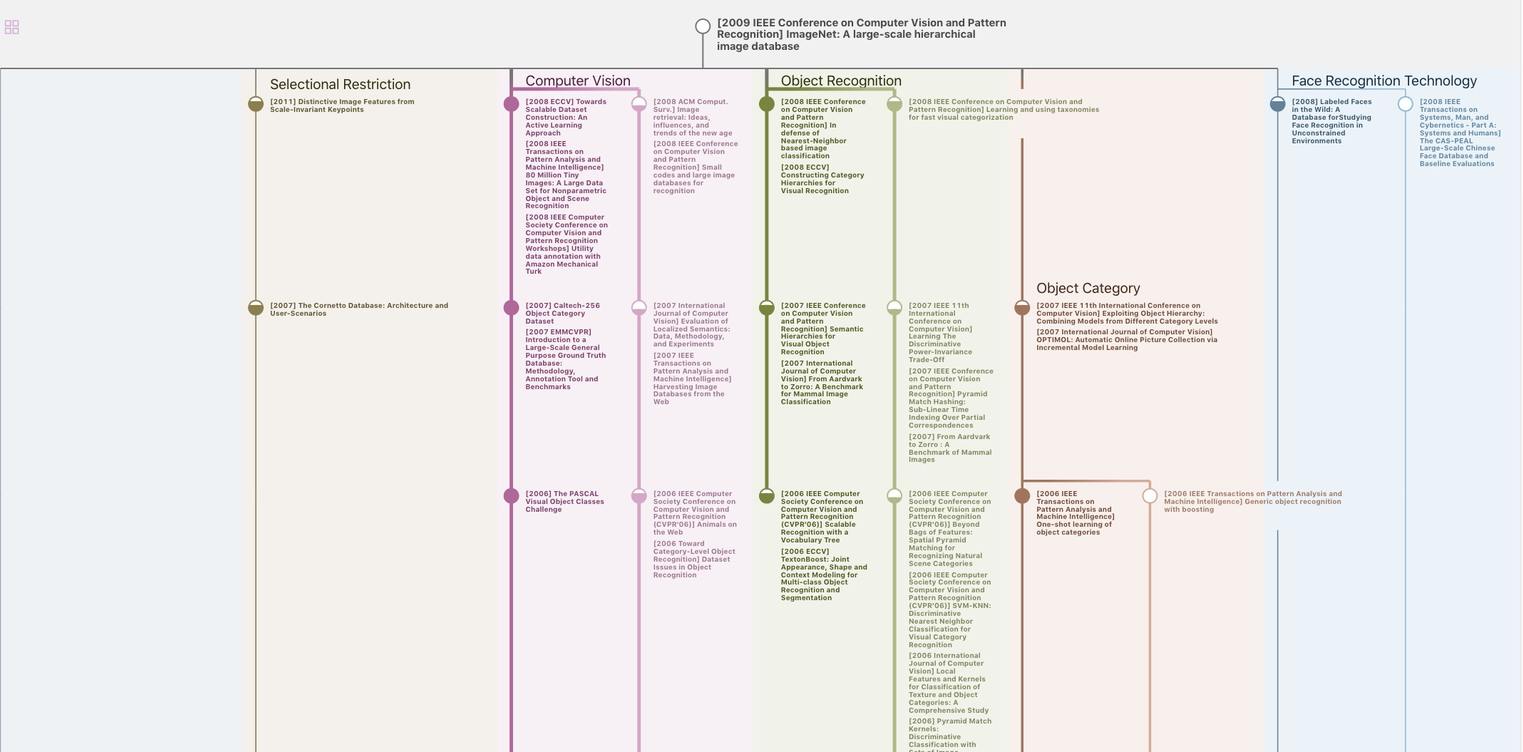
生成溯源树,研究论文发展脉络
Chat Paper
正在生成论文摘要