Instance Segmentation Network With Self-Distillation for Scene Text Detection.
IEEE ACCESS(2020)
摘要
Segmentation based methods have become the mainstream for detecting scene text with arbitrary orientations and shapes. In order to address challenging problems such as separating the text instances that are very close to each other, however, these methods often require time-consuming post-processing. In this paper, we propose an instance segmentation network (ISNet), which simultaneously generates prototype masks and per-instance mask coefficients. After linearly combining the two components, ISNet can implement fast text location. Furthermore, we apply self-distillation to train the ISNet and refine its detection accuracy. We have evaluated the proposed method on four popular benchmarks, i.e., ICDAR2015, ICDAR2017 MLT, CTW1500 and Total-Text, and the experimental results show that it can achieve better tradeoff between accuracy and efficiency for scene text detection.
更多查看译文
关键词
Training,Shape,Prototypes,Feature extraction,Image segmentation,Convolution,Machine learning,Scene text detection,deep learning,self-distillation,instance segmentation
AI 理解论文
溯源树
样例
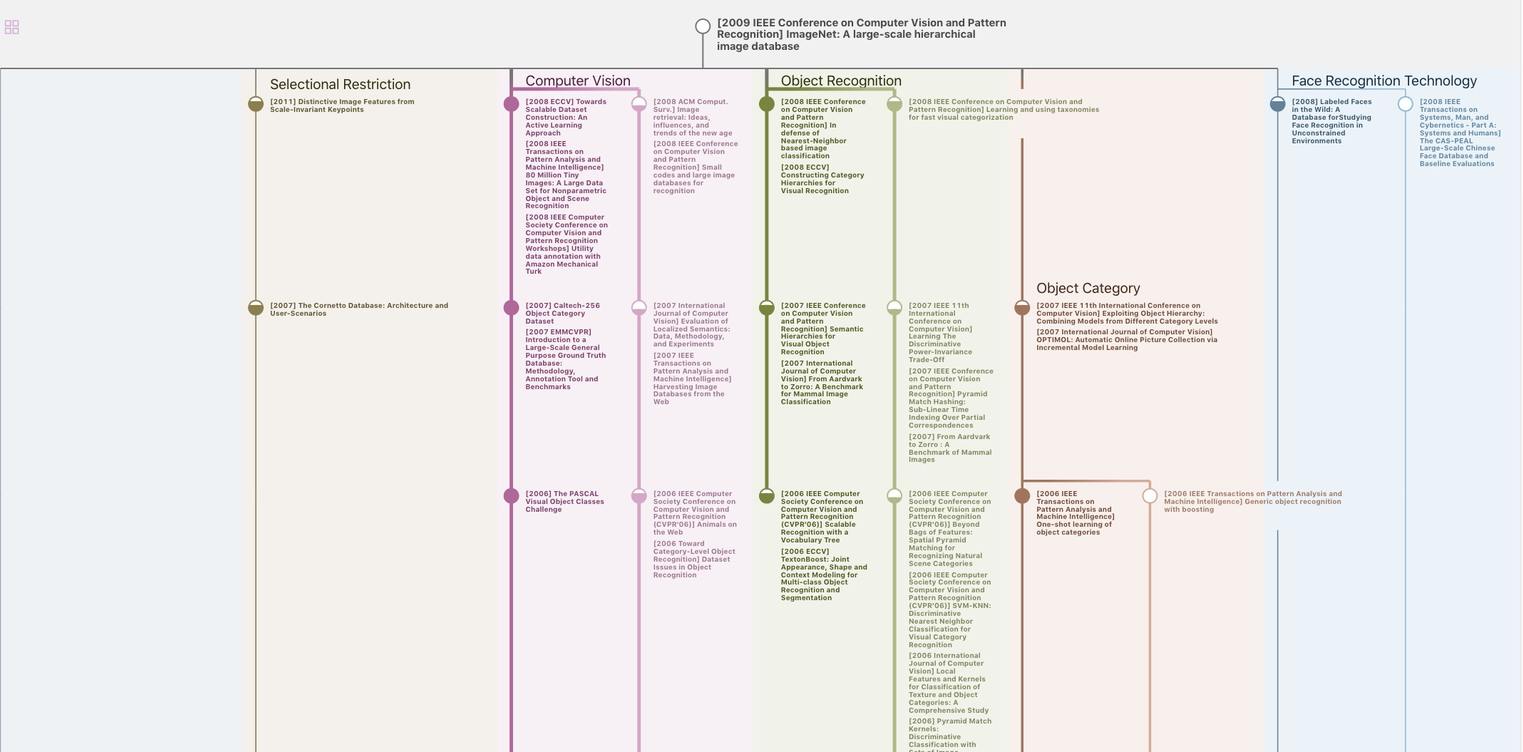
生成溯源树,研究论文发展脉络
Chat Paper
正在生成论文摘要