Unsupervised Fuzzy Measure Learning For Classifier Ensembles From Coalitions Performance
IEEE ACCESS(2020)
摘要
In Machine Learning an ensemble refers to the combination of several classifiers with the objective of improving the performance of every one of its counterparts. To design an ensemble two main aspects must be considered: how to create a diverse set of classifiers and how to combine their outputs. This work focuses on the latter task. More specifically, we focus on the usage of aggregation functions based on fuzzy measures, such as the Sugeno and Choquet integrals, since they allow to model the coalitions and interactions among the members of the ensemble. In this scenario the challenge is how to construct a fuzzy measure that models the relations among the members of the ensemble. We focus on unsupervised methods for fuzzy measure construction, review existing alternatives and categorize them depending on their features. Furthermore, we intend to address the weaknesses of previous alternatives by proposing a new construction method that obtains the fuzzy measure directly evaluating the performance of each possible subset of classifiers, which can be efficiently computed. To test the usefulness of the proposed fuzzy measure, we focus on the application of ensembles for imbalanced datasets. We consider a set of 66 imbalanced datasets and develop a complete experimental study comparing the reviewed methods and our proposal.
更多查看译文
关键词
Fuzzy measures, Choquet integral, aggregation, ensembles, classification
AI 理解论文
溯源树
样例
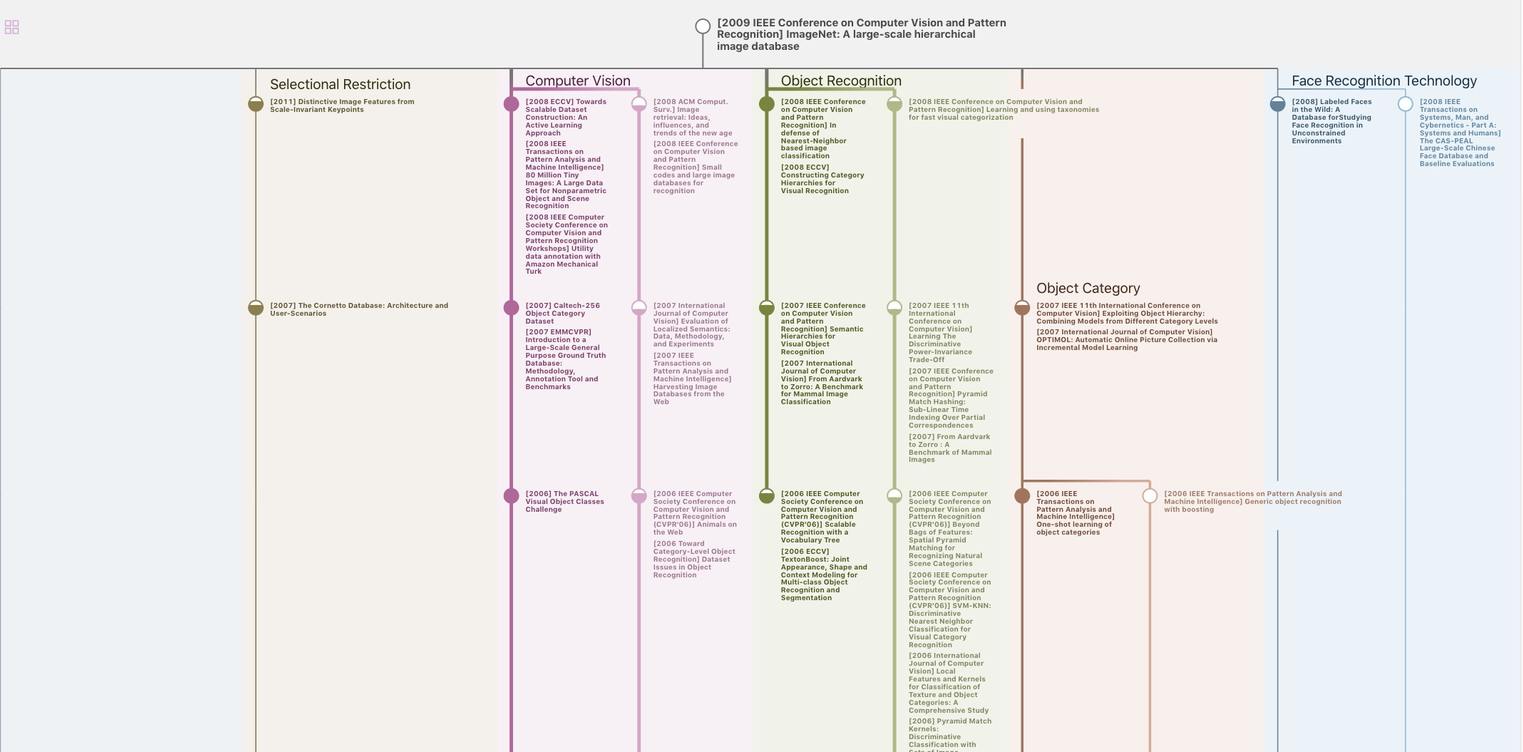
生成溯源树,研究论文发展脉络
Chat Paper
正在生成论文摘要