A Fast And Intelligent Open-Circuit Fault Diagnosis Method For A Five-Level Nnpp Converter Based On An Improved Feature Extraction And Selection Model
IEEE ACCESS(2020)
摘要
The open-circuit faults of power semiconductor devices in multilevel converters are generally diagnosed by analyzing circuit signals. For converters with five or more levels, the difficulty of fault detection increases with increasing topological complexity, the number of switching devices and the number of candidate signal parameters. In this paper, a complete solution for open-circuit fault detection for a five-level nested neutral-point piloted (NNPP) converter is proposed based on improved unsupervised feature learning algorithms. Feature engineering and machine learning algorithms are applied for feature extraction and selection and the construction of classification models. Circuit signals are monitored, and their time-domain characteristics are extracted for fault recognition. An unsupervised feature learning selector, which combines a dependence-guided unsupervised feature selection (DGUFS) filter and a random forest feature selection (RFFS) wrapper to automatically select parameters and generate the optimal feature subset for fault detection, is proposed. The random forest (RF) algorithm is used to build a classifier. The experimental results show that the solution framework presented has the advantages of high efficiency, high flexibility, a superior fault recognition rate and good generalization ability.
更多查看译文
关键词
Fault diagnosis, feature extraction and selection, filter-wrapper method, DGUFS-RFFS, open-circuit fault, five-level NNPP converter
AI 理解论文
溯源树
样例
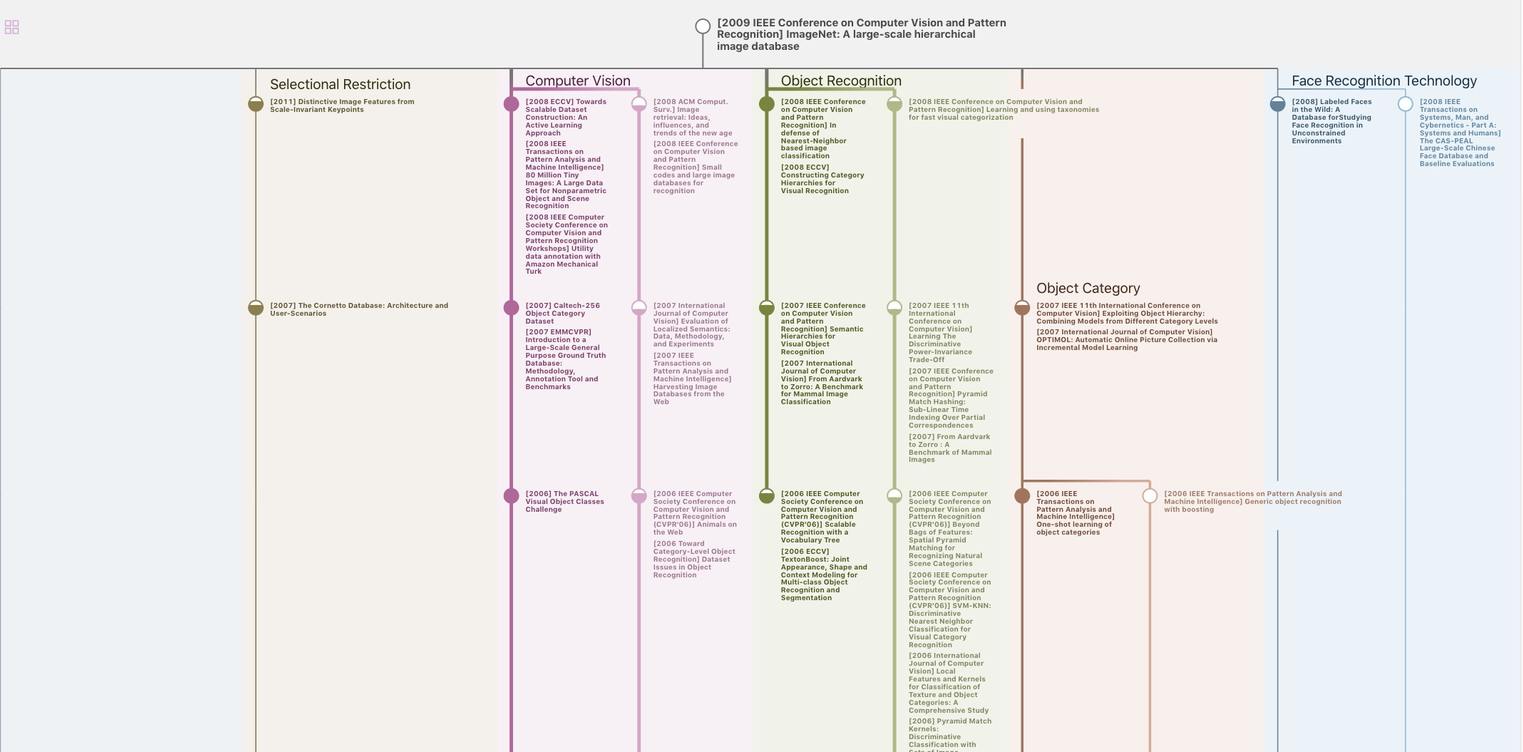
生成溯源树,研究论文发展脉络
Chat Paper
正在生成论文摘要