Privacy Preserving Feature Selection And Multiclass Classification For Horizontally Distributed Data
MATHEMATICAL FOUNDATIONS OF COMPUTING(2018)
摘要
In the last two decades, a lot of scientific fields have experienced a huge growth in data volume and data complexity, which brings data miners lots of opportunities, as well as many challenges. With the advent of the era of big data, applying data mining techniques on assembling data from multiple parties (or sources) has become a leading trend. However, those data mining tasks may divulge individuals' privacy, which leads to the increased concerns in privacy preserving. In this work, a Privacy Preserving feature selection method (PPFS-IFW) and Multiclass Classification method (PPM2C) are proposed. Experiments had been conducted to validate the performance of the proposed approaches. Both PPFS-IFW and PPM2C were tested on six benchmark datasets. The testing results demonstrate PPFS-IFW's capability in enhancing the classification performance at the level of accuracy by selection informative features. PPFS-IFW can not only preserve private information but also outperform some other state-of-the-art feature selection approaches. Experimental results also show that the proposed PPM2C method is workable and stable. Particularly, It reduces the risk of over-fitting when compared with the regular Support Vector Machine. In the meantime, by employing the Secure Sum Protocol to encrypt data at the bottom layer, users' privacy is preserved.
更多查看译文
关键词
Classification, selection, privacy, feature selection
AI 理解论文
溯源树
样例
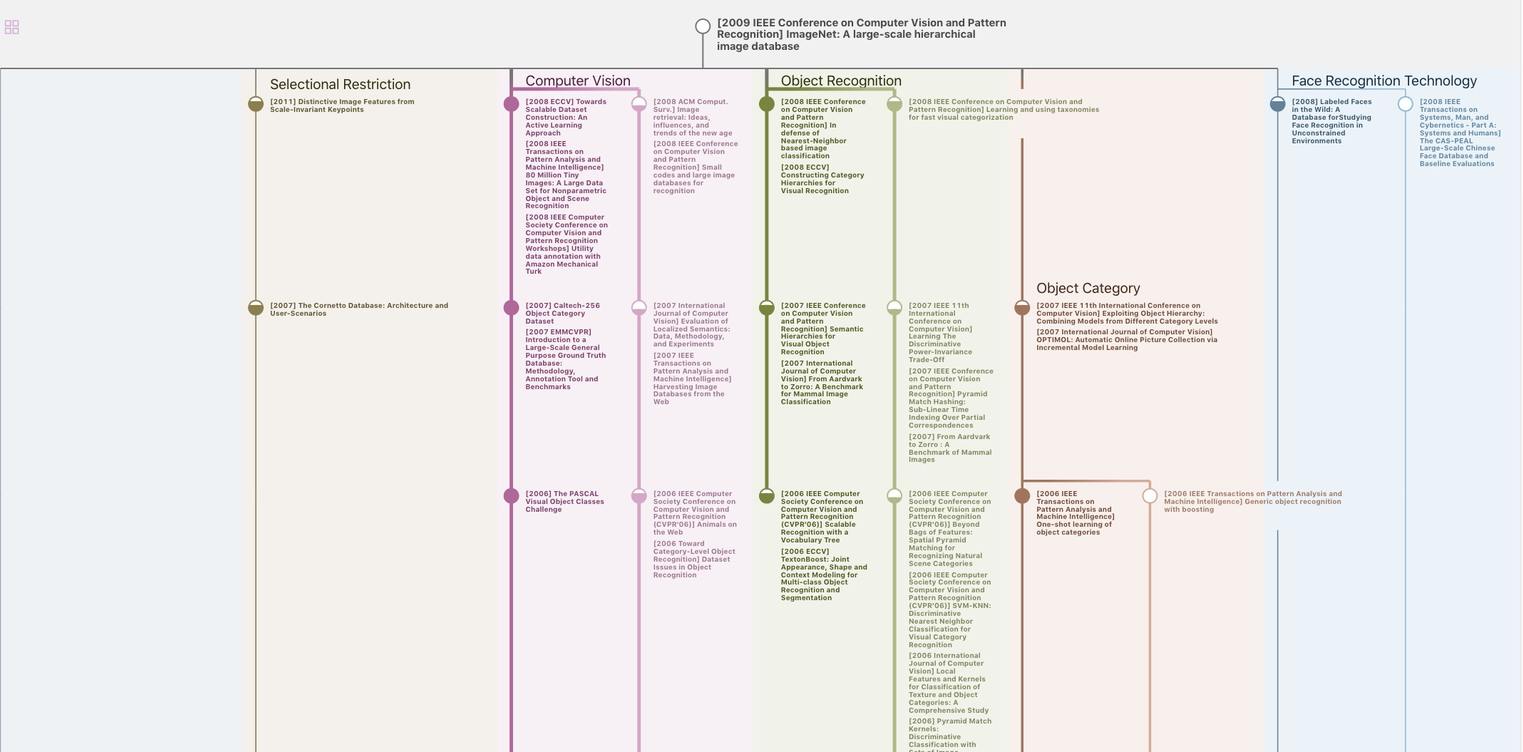
生成溯源树,研究论文发展脉络
Chat Paper
正在生成论文摘要