Learning With Semi-Definite Programming: Statistical Bounds Based On Fixed Point Analysis And Excess Risk Curvature
JOURNAL OF MACHINE LEARNING RESEARCH(2021)
摘要
Many statistical learning problems have recently been shown to be amenable to Semi Definite Programming (SDP), with community detection and clustering in Gaussian mixture models as the most striking instances Javanmard et al. (2016). Given the growing range of applications of SDP-based techniques to machine learning problems, and the rapid progress in the design of efficient algorithms for solving SDPs, an intriguing question is to understand how the recent advances from empirical process theory and Statistical Learning Theory can be leveraged for providing a precise statistical analysis of SDP estimators. In the present paper, we borrow cutting edge techniques and concepts from the Learning Theory literature, such as fixed point equations and excess risk curvature arguments, which yield general estimation and prediction results for a wide class of SDP estimators. From this perspective, we revisit some classical results in community detection from Gue acute accent don and Vershynin (2016) and Fei and Chen (2019b), and we obtain statistical guarantees for SDP estimators used in signed clustering, angular group synchronization (for both multiplicative and additive models) and MAX-CUT. Our theoretical findings are complemented by numerical experiments for each of the three problems considered, showcasing the competitiveness of the SDP estimators.
更多查看译文
关键词
Semi-Definite Programming, Statistical Learning, Group Synchronization, Signed Clustering
AI 理解论文
溯源树
样例
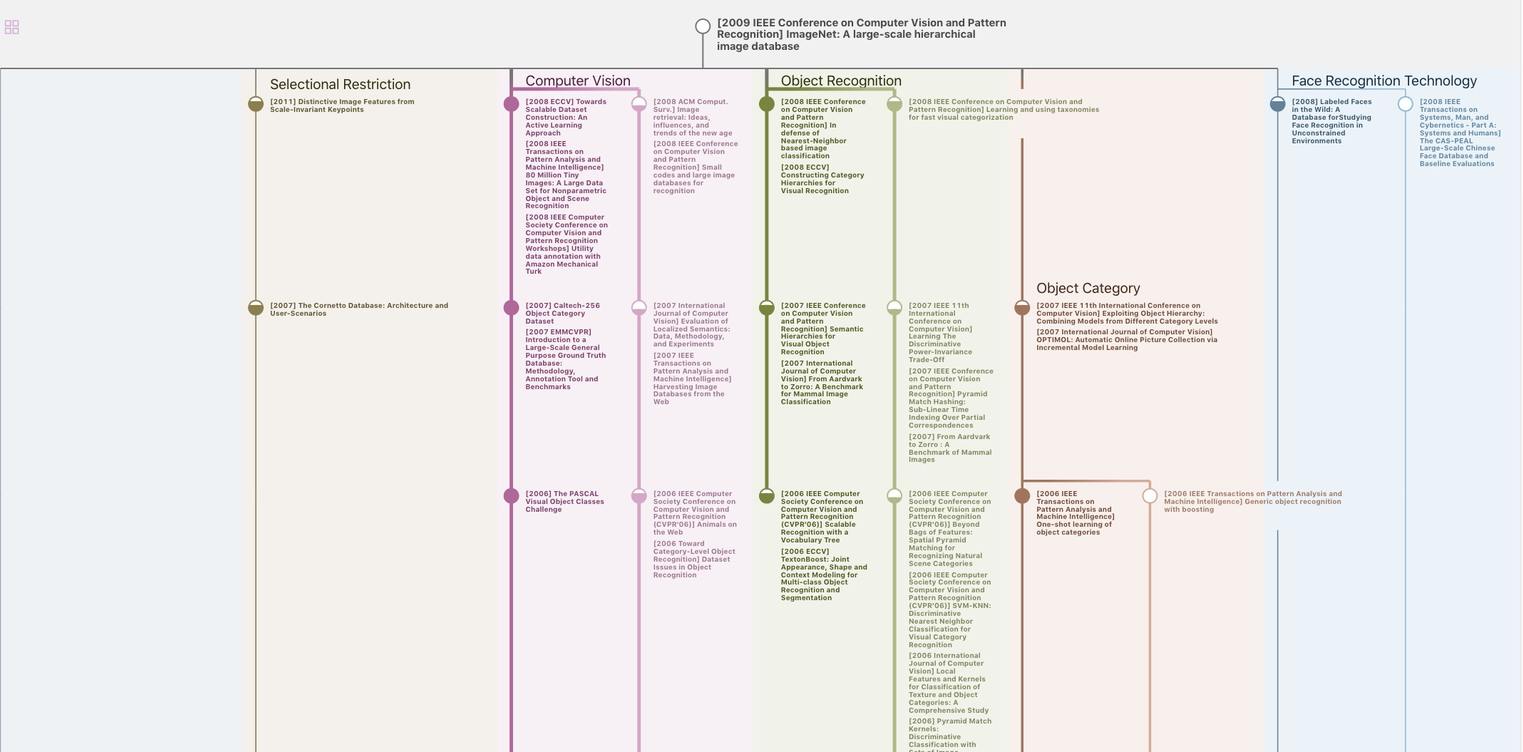
生成溯源树,研究论文发展脉络
Chat Paper
正在生成论文摘要