Modeling, Analysis, and Optimization of Grant-Free NOMA in Massive MTC via Stochastic Geometry
IEEE Internet of Things Journal(2021)
摘要
In the massive machine-type communications (mMTCs) scenario for Internet-of-Things (IoTs) applications, though a large number of devices are registered to an access point (AP), very few of them are active with uplink short packet transmission simultaneously, which requires a novel design of protocols and receivers to enable efficient data transmission and accurate multiuser detection (MUD). Aiming at this problem, grant-free nonorthogonal multiple access (GF-NOMA) is proposed, which enables active devices to directly transmit their preambles and data symbols altogether within one time frame, without grant from the AP. Compressive sensing (CS)-based receivers are adopted for nonorthogonal preambles (NOPs)-based MUD, and successive interference cancellation is exploited to decode the superimposed data signals. In this article, from an aspect of network deployment, we model, analyze, and optimize the CS-based GF-MONA mMTC system via stochastic geometry (SG). Based on the SG network model, we first analyze the success probability as well as the channel estimation error of the CS-based MUD in the preamble phase and then analyze the average aggregate data rate in the data phase. We optimize the energy efficiency and AP coverage efficiency for GF-NOMA via numerical methods to meet the low-energy-consumption and low-infrastructure-cost demands of IoT applications. Our analysis results are verified with Monte Carlo simulations, which show that CS-based GF-NOMA with NOP yields better MUD and data rate performances than contention-based GF-NOMA with orthogonal preambles and grant-free orthogonal multiple access.
更多查看译文
关键词
Compressed sensing,grant-free,massive machine-type communications (mMTCs),nonorthogonal multiple access (NOMA),stochastic geometry (SG)
AI 理解论文
溯源树
样例
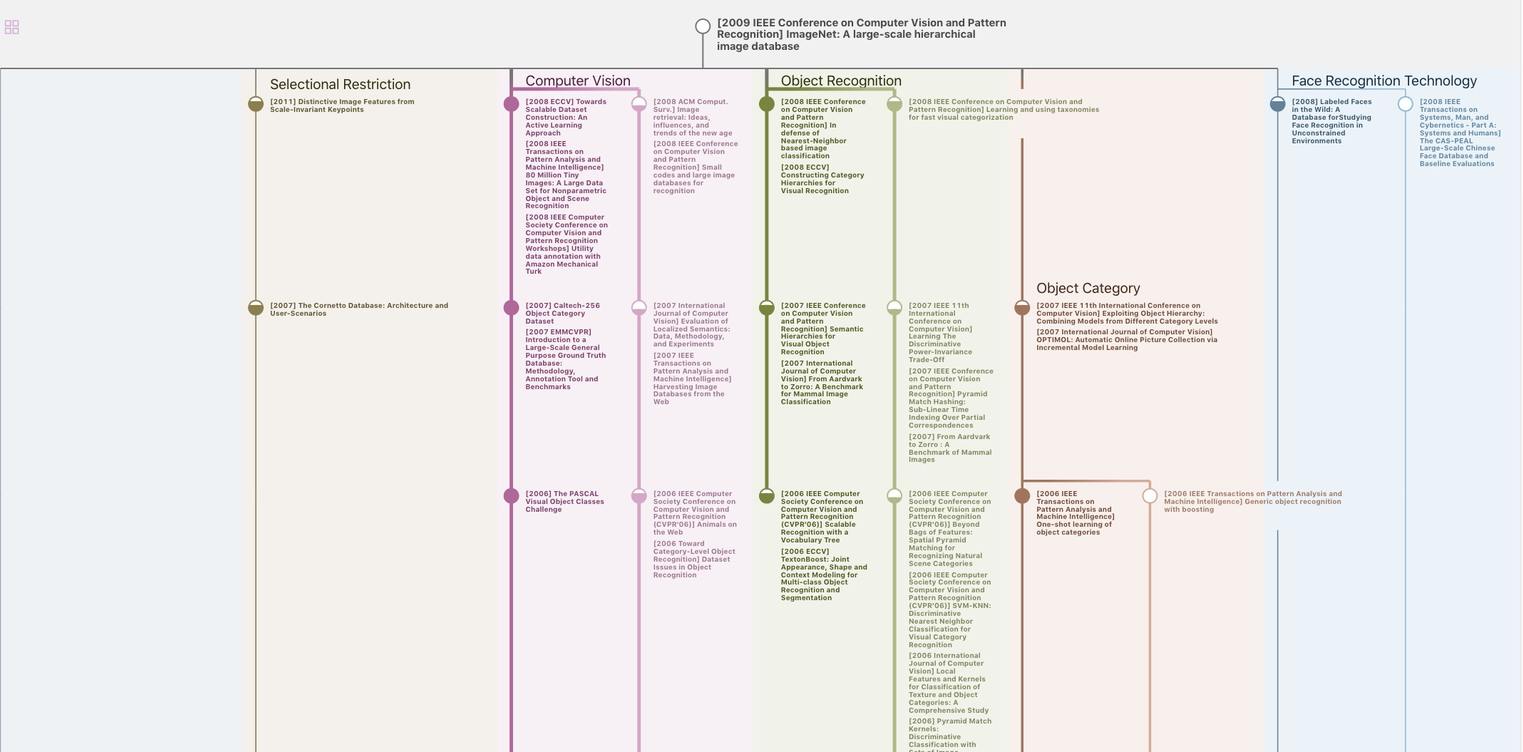
生成溯源树,研究论文发展脉络
Chat Paper
正在生成论文摘要