Sparse Text Generation
Conference on Empirical Methods in Natural Language Processing(2020)
摘要
Current state-of-the-art text generators build on powerful language models such as GPT-2, which have impressive performance. However, to avoid degenerate text, they require sampling from a modified softmax, via temperature parameters or ad-hoc truncation techniques, as in top-$k$ or nucleus sampling. This creates a mismatch between training and testing conditions. In this paper, we use the recently introduced entmax transformation to train and sample from a natively sparse language model, avoiding this mismatch. The result is a text generator with favorable performance in terms of fluency and consistency, fewer repetitions, and n-gram diversity closer to human text. In order to evaluate our model, we propose three new metrics that are tailored for comparing sparse or truncated distributions: $\epsilon$-perplexity, sparsemax score, and Jensen-Shannon divergence. Human-evaluated experiments in story completion and dialogue generation show that entmax sampling leads to more engaging and coherent stories and conversations.
更多查看译文
关键词
text,generation
AI 理解论文
溯源树
样例
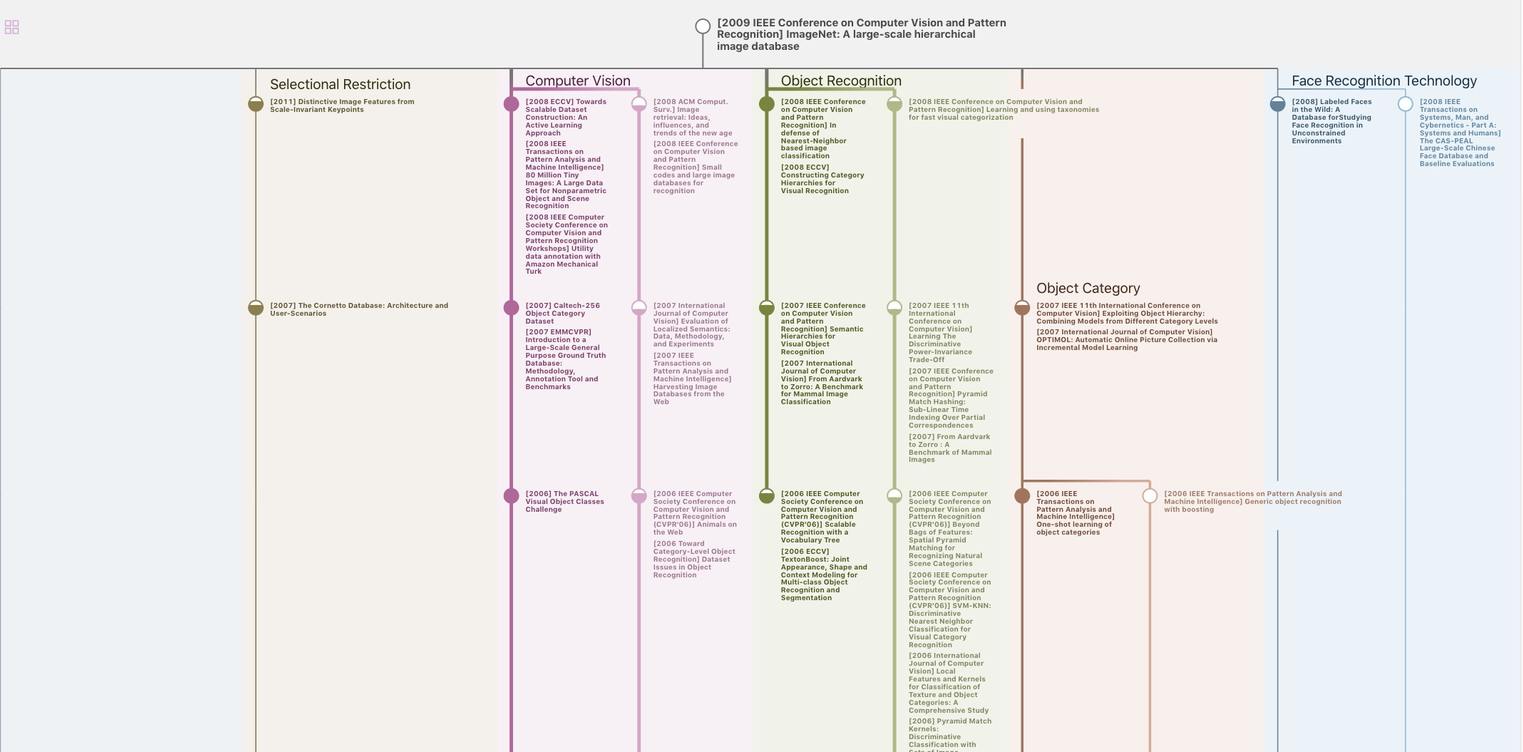
生成溯源树,研究论文发展脉络
Chat Paper
正在生成论文摘要