Active Recursive Bayesian Inference with Posterior Trajectory Analysis Using $\alpha$-Divergence
arxiv(2020)
摘要
Recursive Bayesian inference (RBI) provides optimal Bayesian latent variable estimates in real-time settings with streaming noisy observations. Active RBI attempts to effectively select queries that lead to more informative observations to rapidly reduce uncertainty until a confident decision is made. However, typically the optimality objectives of inference and query mechanisms are not jointly selected. Furthermore, conventional active querying methods stagger due to misleading prior information. Motivated by information theoretic approaches, we propose an active RBI framework with unified inference and query selection steps through Renyi entropy and $\alpha$-divergence. We also propose a new objective based on Renyi entropy and its changes called Momentum that encourages exploration for misleading prior cases. The proposed active RBI framework is applied to the trajectory of the posterior changes in the probability simplex that provides a coordinated active querying and decision making with specified confidence. Under certain assumptions, we analytically demonstrate that the proposed approach outperforms conventional methods such as mutual information by allowing the selections of unlikely events. We present empirical and experimental performance evaluations on two applications: restaurant recommendation and brain-computer interface (BCI) typing systems.
更多查看译文
关键词
active recursive bayesian inference,posterior trajectory analysis
AI 理解论文
溯源树
样例
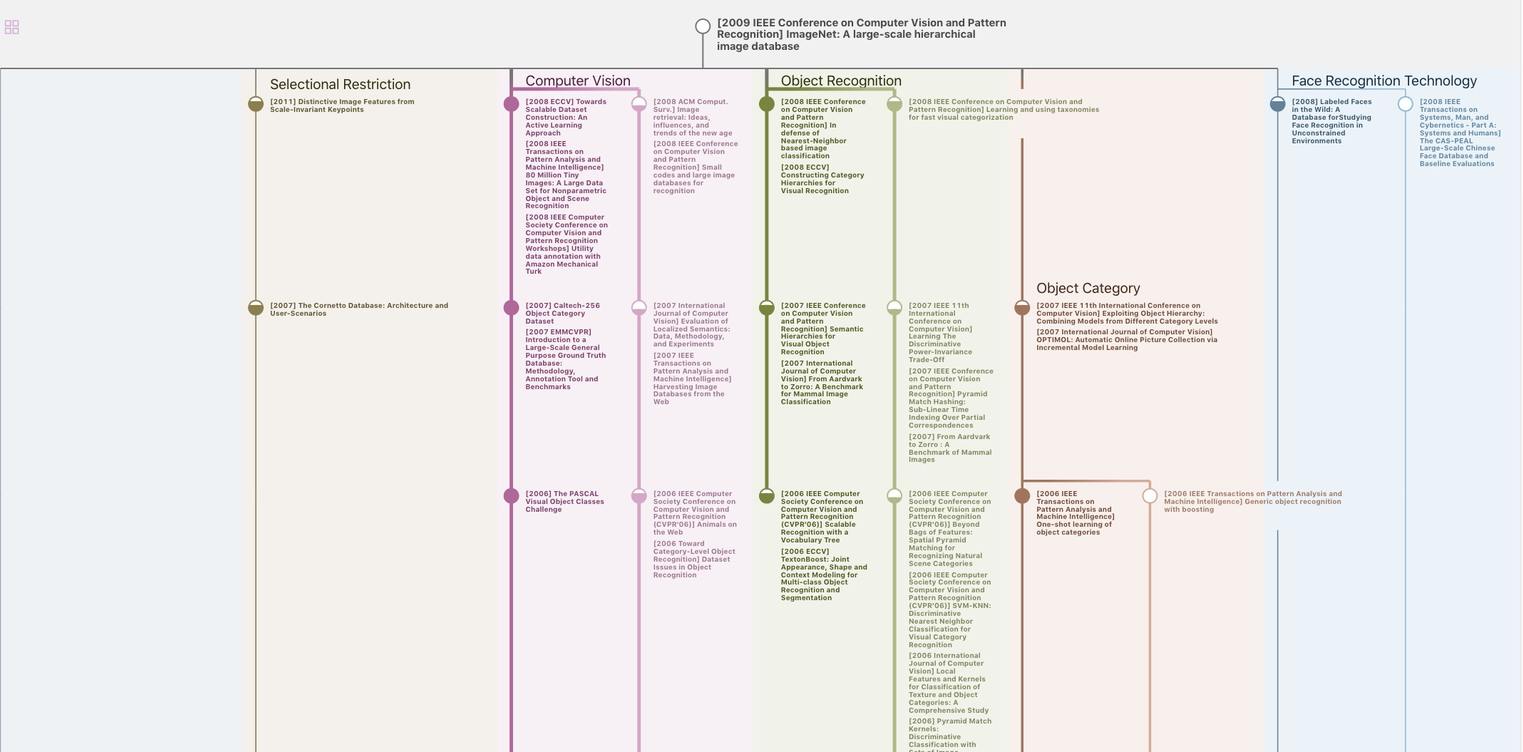
生成溯源树,研究论文发展脉络
Chat Paper
正在生成论文摘要