Leant Network Structure Learning From High-Dimensional Multivariate Point Processes
arxiv(2020)
摘要
Learning the latent network structure from large scale multivariate point process data is an important task in a wide range of scientific and business applications. For instance, we might wish to estimate the neuronal functional connectivity network based on spiking times recorded from a collection of neurons. To characterize the complex processes underlying the observed data, we propose a new and flexible class of nonstationary Hawkes processes that allow both excitatory and inhibitory effects. We estimate the latent network structure using an efficient sparse least squares estimation approach. Using a thinning representation, we establish concentration inequalities for the first and second order statistics of the proposed Hawkes process. Such theoretical results enable us to establish the non-asymptotic error bound and the selection consistency of the estimated parameters. Furthermore, we describe a least squares loss based statistic for testing if the background intensity is constant in time. We demonstrate the efficacy of our proposed method through simulation studies and an application to a neuron spike train dataset. Supplementary materials for this article are available online.
更多查看译文
关键词
Multivariate Hawkes process, Non asymptotic error bound, Nonlinear Hawkes process, Nonstationary, Selection consistency
AI 理解论文
溯源树
样例
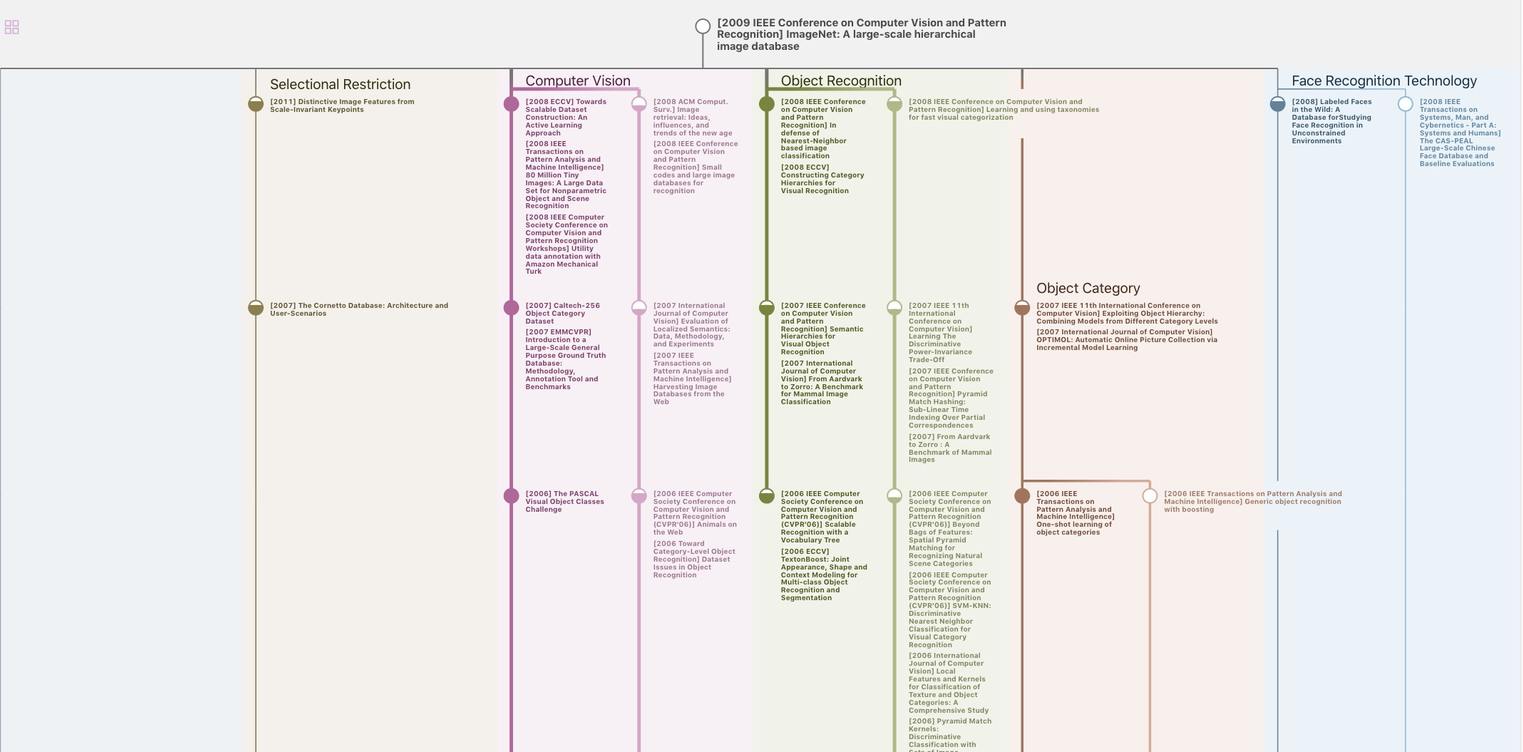
生成溯源树,研究论文发展脉络
Chat Paper
正在生成论文摘要