Real-time Fabric Defect Detection based on Lightweight Convolutional Neural Network.
ICCPR(2019)
摘要
Fabric defect detection is an important link for quality control in a textile factory. Deep convolutional neural network (CNN) has made great progress in the field of target detection, and has proven applicable in fabric defect detection. However, the improvement of detection performance of CNN network mainly depends on complex network structure. This comes the drawbacks of significant increase in computational costs and storage services, which seriously hinders the usages of CNN on resource-limited environments, such as smart industrial cameras and other embedded devices. In this paper, a lightweight CNN model is designed for fabric defect detection, and denoted as DefectNet. It is based on a streamlined architecture that uses depthwise separable convolutions instead of standard convolutions to build a lightweight neural network architecture, and significantly reduce the computational complexity of the model. The multi-scale feature extraction method is used to improve the detection ability of the model for fabric defects of various sizes. Experimental results demonstrate that the proposed scheme has higher detection accuracy and faster detection speed on the basis of fewer network parameters, which can provide real-time fabric defects detection on the embedded devices.
更多查看译文
AI 理解论文
溯源树
样例
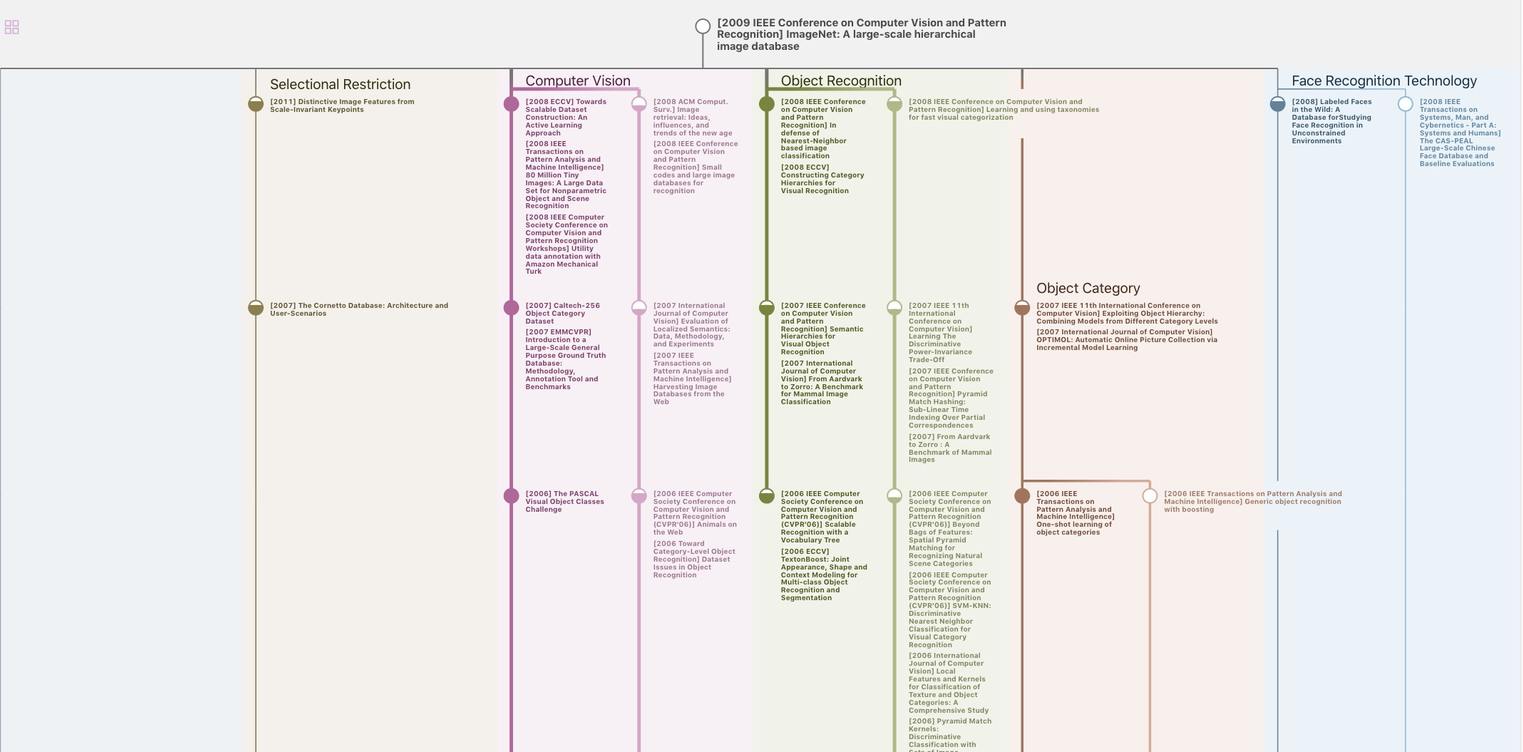
生成溯源树,研究论文发展脉络
Chat Paper
正在生成论文摘要