DMLO: Deep Matching LiDAR Odometry
IROS(2020)
摘要
LiDAR odometry is a fundamental task for various areas such as robotics, autonomous driving. This problem is difficult since it requires the systems to be highly robust running in noisy real-world data. Existing methods are mostly local iterative methods. Feature-based global registration methods are not preferred since extracting accurate matching pairs in the nonuniform and sparse LiDAR data remains challenging. In this paper, we present Deep Matching LiDAR Odometry (DMLO), a novel learning-based framework which makes the feature matching method applicable to LiDAR odometry task. Unlike many recent learning-based methods, DMLO explicitly enforces geometry constraints in the framework. Specifically, DMLO decomposes the 6-DoF pose estimation into two parts, a learning-based matching network which provides accurate correspondences between two scans and rigid transformation estimation with a close-formed solution by Singular Value Decomposition (SVD). Comprehensive experimental results on real-world datasets KITTI and Argoverse demonstrate that our DMLO dramatically outperforms existing learning-based methods and comparable with the state-of-the-art geometry based approaches.
更多查看译文
关键词
deep matching lidar odometry,dmlo
AI 理解论文
溯源树
样例
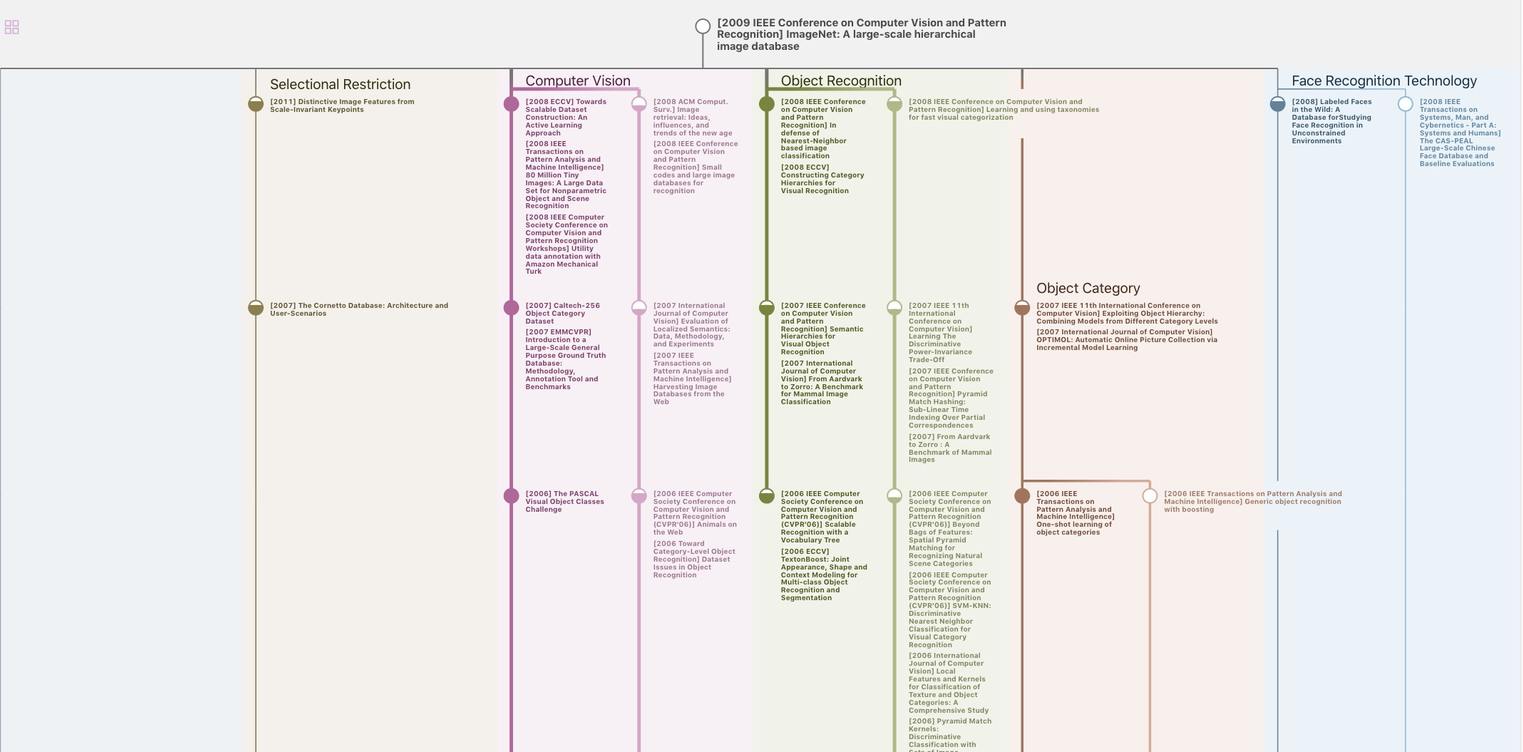
生成溯源树,研究论文发展脉络
Chat Paper
正在生成论文摘要