Manipulation-Proof Machine Learning
arxiv(2020)
摘要
An increasing number of decisions are guided by machine learning algorithms. In many settings, from consumer credit to criminal justice, those decisions are made by applying an estimator to data on an individual's observed behavior. But when consequential decisions are encoded in rules, individuals may strategically alter their behavior to achieve desired outcomes. This paper develops a new class of estimator that is stable under manipulation, even when the decision rule is fully transparent. We explicitly model the costs of manipulating different behaviors, and identify decision rules that are stable in equilibrium. Through a large field experiment in Kenya, we show that decision rules estimated with our strategy-robust method outperform those based on standard supervised learning approaches.
更多查看译文
关键词
learning,machine,manipulation-proof
AI 理解论文
溯源树
样例
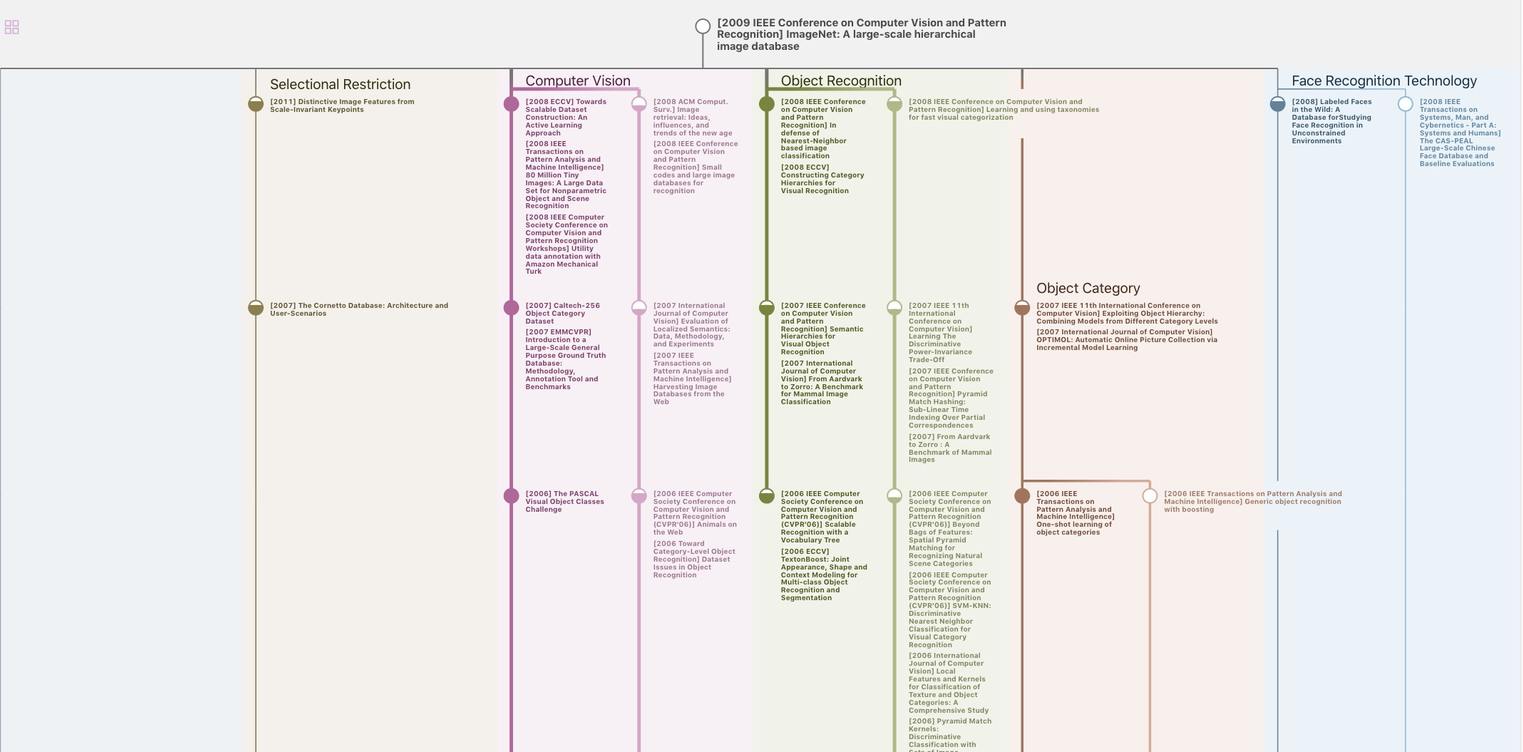
生成溯源树,研究论文发展脉络
Chat Paper
正在生成论文摘要