Semi-supervised acoustic and language model training for English-isiZulu code-switched speech recognition
CodeSwitch@LREC(2020)
摘要
We present an analysis of semi-supervised acoustic and language model training for English-isiZulu code-switched ASR using soap opera speech. Approximately 11 hours of untranscribed multilingual speech was transcribed automatically using four bilingual code-switching transcription systems operating in English-isiZulu, English-isiXhosa, English-Setswana and English-Sesotho. These transcriptions were incorporated into the acoustic and language model training sets. Results showed that the TDNN-F acoustic models benefit from the additional semi-supervised data and that even better performance could be achieved by including additional CNN layers. Using these CNN-TDNN-F acoustic models, a first iteration of semi-supervised training achieved an absolute mixed-language WER reduction of 3.4%, and a further 2.2% after a second iteration. Although the languages in the untranscribed data were unknown, the best results were obtained when all automatically transcribed data was used for training and not just the utterances classified as English-isiZulu. Despite reducing perplexity, the semi-supervised language model was not able to improve the ASR performance.
更多查看译文
关键词
speech recognition,language model training,semi-supervised,english-isizulu,code-switched
AI 理解论文
溯源树
样例
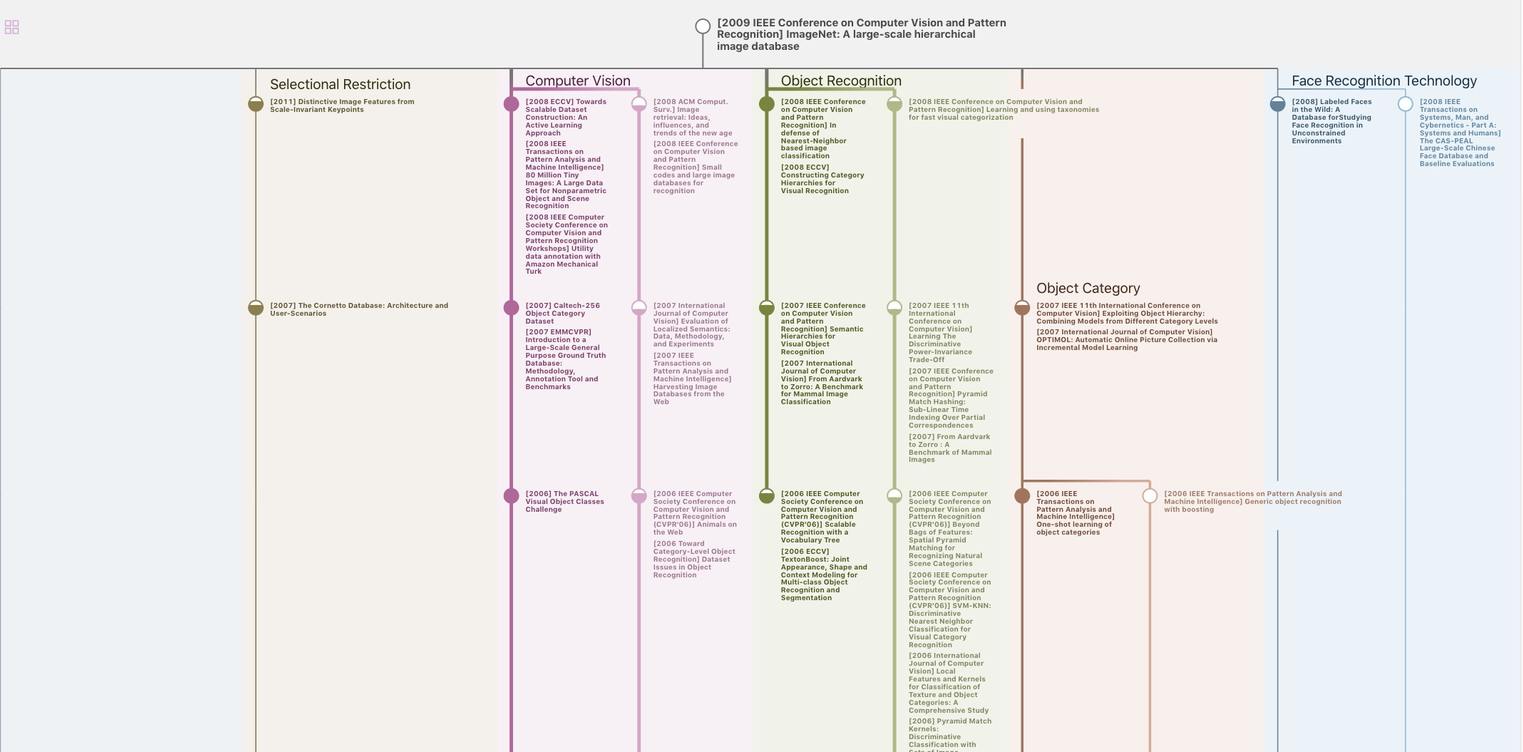
生成溯源树,研究论文发展脉络
Chat Paper
正在生成论文摘要