Efficient learning of donor retention strategies for the American Red Cross
WSC '13: Winter Simulation Conference Washington D.C. December, 2013(2013)
摘要
The American Red Cross (ARC) and many other disaster relief organizations often encounter the problem of retaining one-time disaster donorspeople who have given their first donation in response to a major disaster. Only 30% of these donors return to give a second time after their initial contribution. A critical problem for the ARC is to motivate disaster donors to contribute to long-term operations and post-disaster programs such as community disaster preparation or emergency response training.
In a typical disaster-donor retention campaign, the ARC sends an appeal for funds (usually a mailed letter) to a segment of disaster donors. The appeal is designed using a combination of features related to the letter format and contents, donor and campaign type, and the use of various gift items. The number of possible designs is exponential in the number of features. The outcome is evaluated using the success rate, defined as the proportion of mailings in a campaign that elicited a donation. The goal is to find the best set of campaign attributes that maximize the success rate as quickly as possible, subject to constraints on the number of campaigns that can be conducted.
Linear regression based on historical data has been used to estimate the effects of the campaign attributes on the success rate (Ryzhov et al. 2013). The results of the estimation, however, are subject to change after every new campaign output, which provides new information about the campaign designs. This potential for information should be used as a criterion when designing the next campaign. The new outcome then feeds back into the linear model and guides the next campaign design, thus forming an actionfeedback loop. This learning problem possesses two important characteristics: 1) A single campaign outcome may provide information about many other alternatives since different campaign designs can share a common set of features; 2)The variance of the observed campaign success rates is unknown. Although the variance can be estimated from historical data, an inaccurate estimate may bias the evaluation of new information.
Within the fundraising community, there exist numerous statistical models for predicting charitable giving (see e.g. Tobin 1958 and Lankford and Wyckoff 1991). Statistical models provide insights on decision and policy making; most of them, however, fail to provide a real-time sequential decision model to guide fundraisers' actions that has the ability to dynamically adapt to new information. While various techniques from operations research---dynamic programming, linear or non-linear programming---have been applied to for-profit marketing to support sequential or non-sequential decisions on variables such as price, advertising expenditure or media coverage (see e.g. Leeflang and Wittink 2000 for introductions), less attention has been given to developing mathematical models for sequential decision making in non-profit marketing.
We present a Bayesian approach to this problem, which assigns prior distributions to unknown values, while anticipating the future outcome when making sequential decisions; see Powell and Ryzhov (2012) for a recent and comprehensive review. We adopt the value of information procedure or VIP (Gupta and Miescke 1996), which is based on an expected improvement criterion, and allows one to handle correlated beliefs, where a single new observation provides information about multiple alternatives. Negoescu et al. (2011) shows how expected improvement can be used to learn in Bayesian linear regression, but assumes a known sampling variance. We present a Bayesian learning model that integrates the ability to handle linear regression together with the unknown-variance model. As a result, we capture both the ability to model correlations between regression parameters and the ability to learn an unknown sampling variance simultaneously with those parameters.
The best available algorithm for calculating expected improvement for correlated beliefs has prohibitively high computational complexity in the regression setting. We address this issue in two ways. First, we derive an easier computation in the special case where two-way interaction attributes are absent from the feature space. For more general cases, we apply a quantization procedure and approximate the non-convex value of information by a convex function, and optimize this function using semidefinite programming relaxations. As a result the model can cope with problems in which the number of campaign alternatives is exponentially large in the number of design features.
The present paper contributes to the literature on non-profit marketing as well as optimal learning. We believe that our work offers a rigorous mathematical model for non-profit sequential decision making, and provides a new approach to optimal learning problems with linear regression structure, unknown variance and large decision space.
更多查看译文
AI 理解论文
溯源树
样例
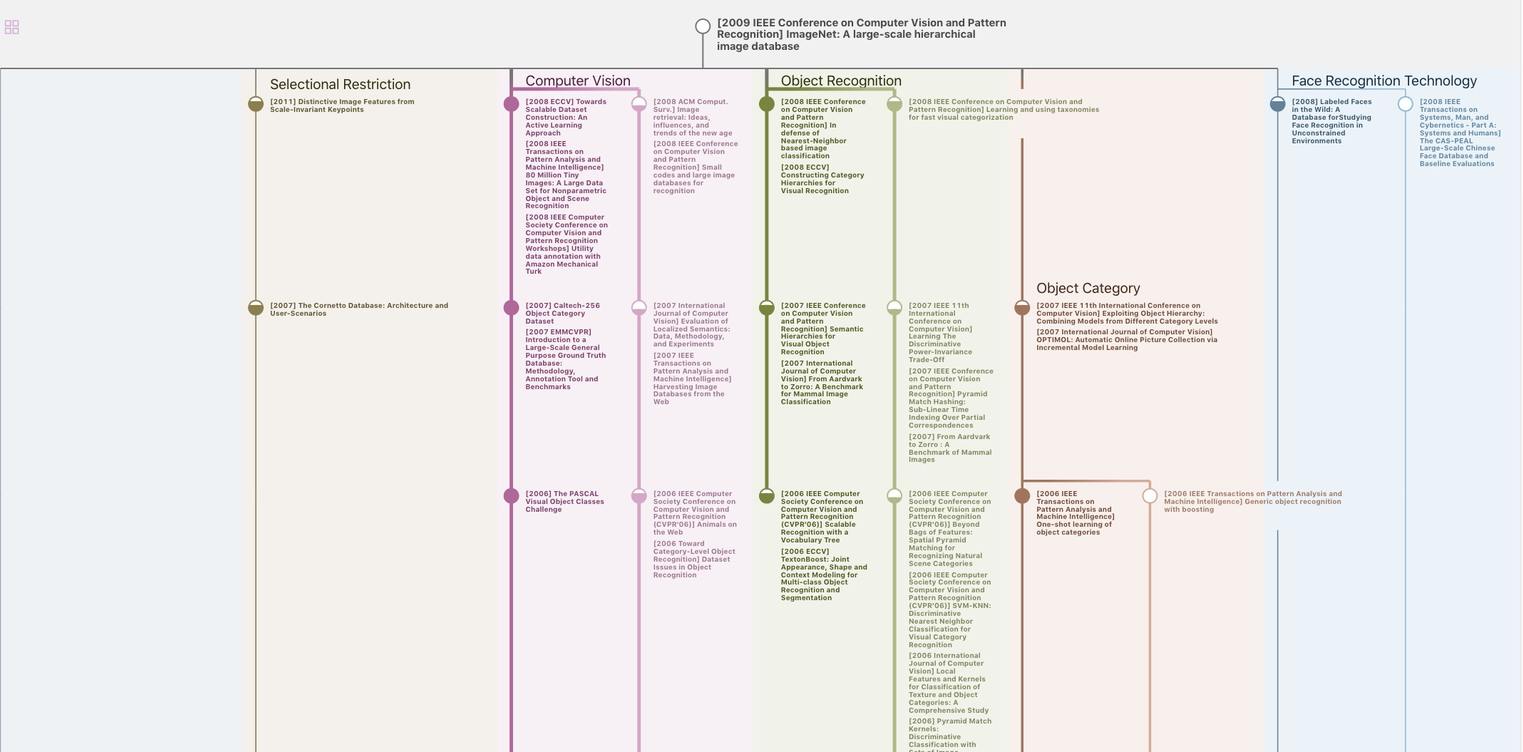
生成溯源树,研究论文发展脉络
Chat Paper
正在生成论文摘要