An Improved Convolution Neural Network-Based Model for Classifying Foliage and Woody Components from Terrestrial Laser Scanning Data.
Remote sensing(2020)
摘要
Separating foliage and woody components can effectively improve the accuracy of simulating the forest eco-hydrological processes. It is still challenging to use deep learning models to classify canopy components from the point cloud data collected in forests by terrestrial laser scanning (TLS). In this study, we developed a convolution neural network (CNN)-based model to separate foliage and woody components (FWCNN) by combing the geometrical and laser return intensity (LRI) information of local point sets in TLS datasets. Meanwhile, we corrected the LRI information and proposed a contribution score evaluation method to objectively determine hyper-parameters (learning rate, batch size, and validation split rate) in the FWCNN model. Our results show that: (1) Correcting the LRI information could improve the overall classification accuracy (OA) of foliage and woody points in tested broadleaf (from 95.05% to 96.20%) and coniferous (from 93.46% to 94.98%) TLS datasets (Kappa ≥ 0.86). (2) Optimizing hyper-parameters was essential to enhance the running efficiency of the FWCNN model, and the determined hyper-parameter set was suitable to classify all tested TLS data. (3) The FWCNN model has great potential to classify TLS data in mixed forests with OA > 84.26% (Kappa ≥ 0.67). This work provides a foundation for retrieving the structural features of woody materials within the forest canopy.
更多查看译文
关键词
point cloud classification,deep learning model,Terrestrial Laser Scanner (TLS),convolution neural network (CNN),hyper-parameter optimization
AI 理解论文
溯源树
样例
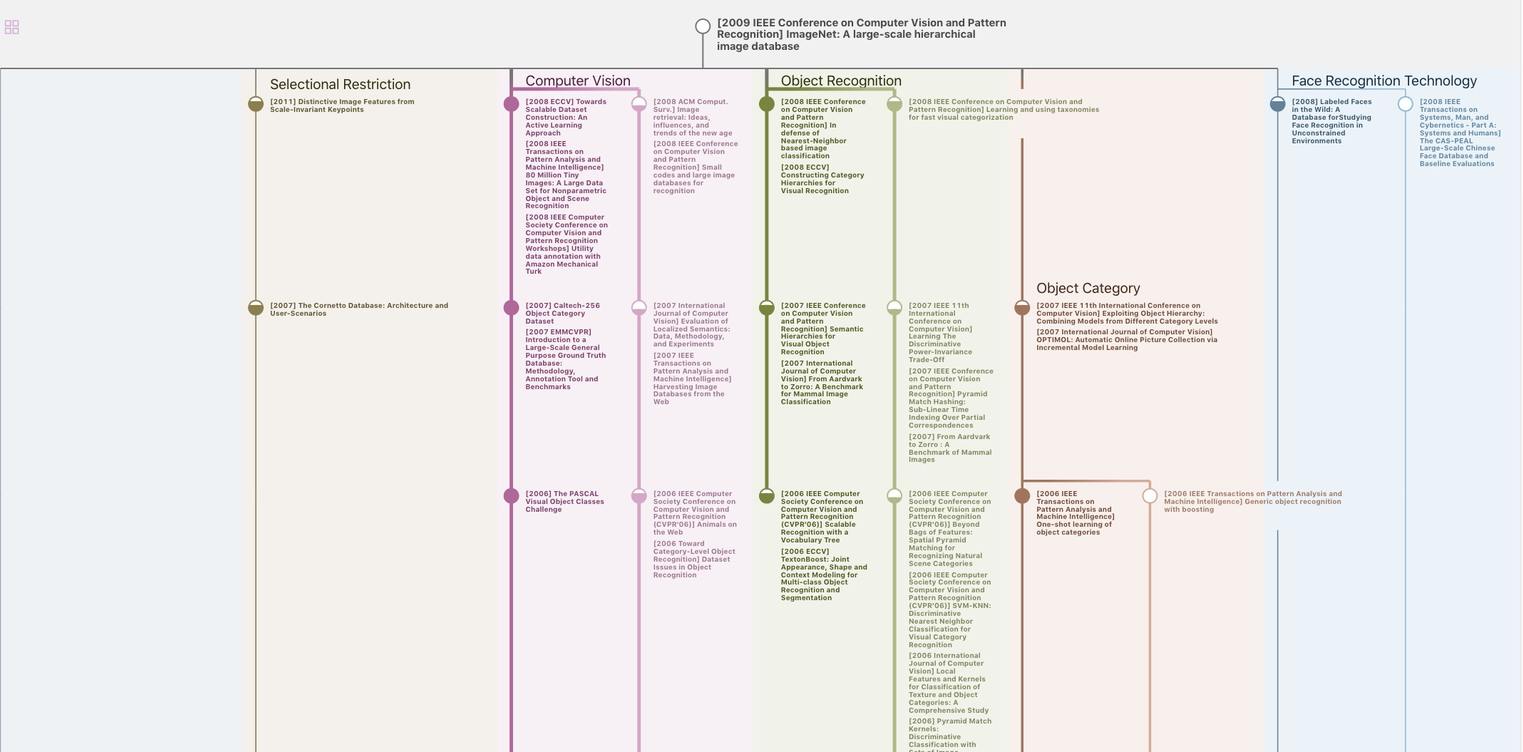
生成溯源树,研究论文发展脉络
Chat Paper
正在生成论文摘要