An Integrated Deep Learning Framework for Joint Segmentation of Blood Pool and Myocardium.
Medical image analysis(2020)
摘要
Simultaneous and automatic segmentation of the blood pool and myocardium is an important precondition for early diagnosis and pre-operative planning in patients with complex congenital heart disease. However, due to the high diversity of cardiovascular structures and changes in mechanical properties caused by cardiac defects, the segmentation task still faces great challenges. To overcome these challenges, in this study we propose an integrated multi-task deep learning framework based on the dilated residual and hybrid pyramid pooling network (DRHPPN) for joint segmentation of the blood pool and myocardium. The framework consists of three closely connected progressive sub-networks. An inception module is used to realize the initial multi-level feature representation of cardiovascular images. A dilated residual network (DRN), as the main body of feature extraction and pixel classification, preliminary predicts segmentation regions. A hybrid pyramid pooling network (HPPN) is designed for facilitating the aggregation of local information to global information, which complements DRN. Extensive experiments on three-dimensional cardiovascular magnetic resonance (CMR) images (the available dataset of the MICCAI 2016 HVSMR challenge) demonstrate that our approach can accurately segment the blood pool and myocardium and achieve competitive performance compared with state-of-the-art segmentation methods.
更多查看译文
关键词
Blood pool,Myocardium,Magnetic resonance image,Segmentation
AI 理解论文
溯源树
样例
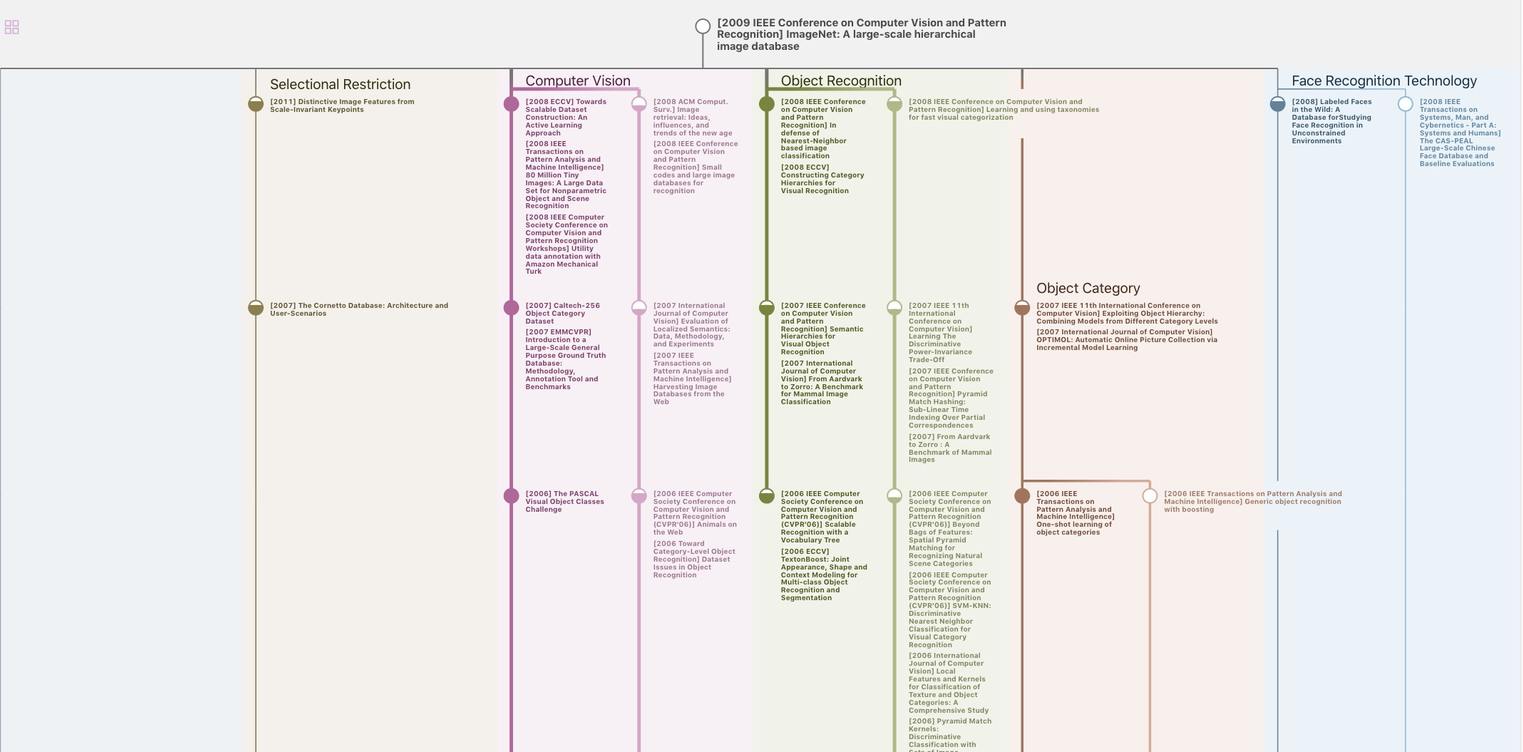
生成溯源树,研究论文发展脉络
Chat Paper
正在生成论文摘要