Prediction of soil adsorption coefficient in pesticides using physicochemical properties and molecular descriptors by machine learning models.
ENVIRONMENTAL TOXICOLOGY AND CHEMISTRY(2020)
摘要
The soil adsorption coefficient (K-OC) plays an important role in environmental risk assessment of pesticide registration. Based on this risk assessment, applied and registered pesticides can be allowed in the European Union. Almost 1 yr is required to study and obtain theK(OC)value of a pesticide. Furthermore, acquiring theK(OC)requires a large cost. It is necessary to efficiently estimate theK(OC)value in the early stages of pesticide development. In the present study, the experimental values of physicochemical properties and molecular descriptors of chemical structures were collected to develop a quantitative structure-property relationship (QSPR) model, and the prediction performance of the model was evaluated. More specifically, we compared the accuracies of models based on a gradient boosting decision tree, multiple linear regression, and support vector machine. The experimental results suggest that it is possible to develop a QSPR model with high accuracy using both the molecular descriptors calculated from the structural formula and experimental values of physicochemical properties from open literature and databases. Comparing to the previously established models, we achieved high prediction accuracy, fitness, and robustness by only using freeware. Therefore, our developed QSPR models can be useful preliminary risk assessment in the early developmental stages of pesticides.Environ Toxicol Chem2020;00:1-9. (c) 2020 SETAC
更多查看译文
关键词
Environmental fate,Pesticide risk assessment,Multivariate statistics,Machine learning,K-OC,Quantitative structure-activity relationship
AI 理解论文
溯源树
样例
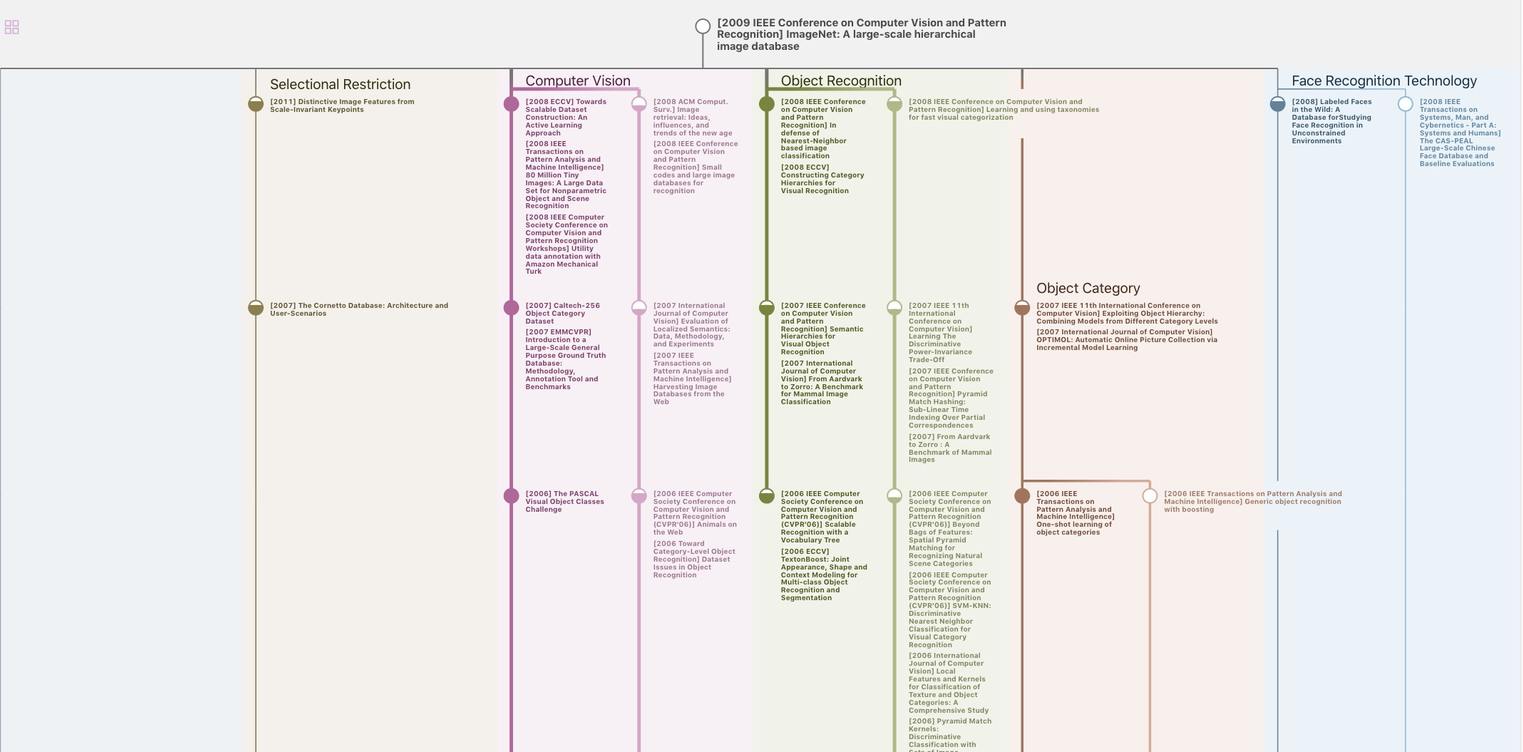
生成溯源树,研究论文发展脉络
Chat Paper
正在生成论文摘要