Neuro-Evolutionary Transfer Learning Through Structural Adaptation.
EvoApplications(2020)
摘要
Transfer learning involves taking an artificial neural network (ANN) trained on one dataset (the source) and adapting it to a new, second dataset (the target). While transfer learning has been shown to be quite powerful and is commonly used in most modern-day statistical learning setups, its use has generally been restricted by architecture, i.e., in order to facilitate the reuse of internal learned synaptic weights, the underlying topology of the ANN to be transferred across tasks must remain the same and a new output layer must be attached (entailing removing the old output layer's weights). This work removes this restriction by proposing a neuro-evolutionary approach that facilitates what we call adaptive structure transfer learning, which means that an ANN can be transferred across tasks that have different input and output dimensions while having the internal latent structure continuously optimized. We test the proposed optimizer on two challenging real-world time series prediction problems - our process adapts recurrent neural networks (RNNs) to (1) predict coal-fired power plant data before and after the addition of new sensors, and to (2) predict engine parameters where RNN estimators are trained on different airframes with different engines. Experiments show that not only does the proposed neuro-evolutionary transfer learning process result in RNNs that evolve and train faster on the target set than those trained from scratch but, in many cases, the RNNs generalize better even after a long training and evolution process. To our knowledge, this work represents the first use of neuro-evolution for transfer learning, especially for RNNs, and is the first methodological framework capable of adapting entire structures for arbitrary input/output spaces.
更多查看译文
关键词
adaptation,transfer,learning,neuro-evolutionary
AI 理解论文
溯源树
样例
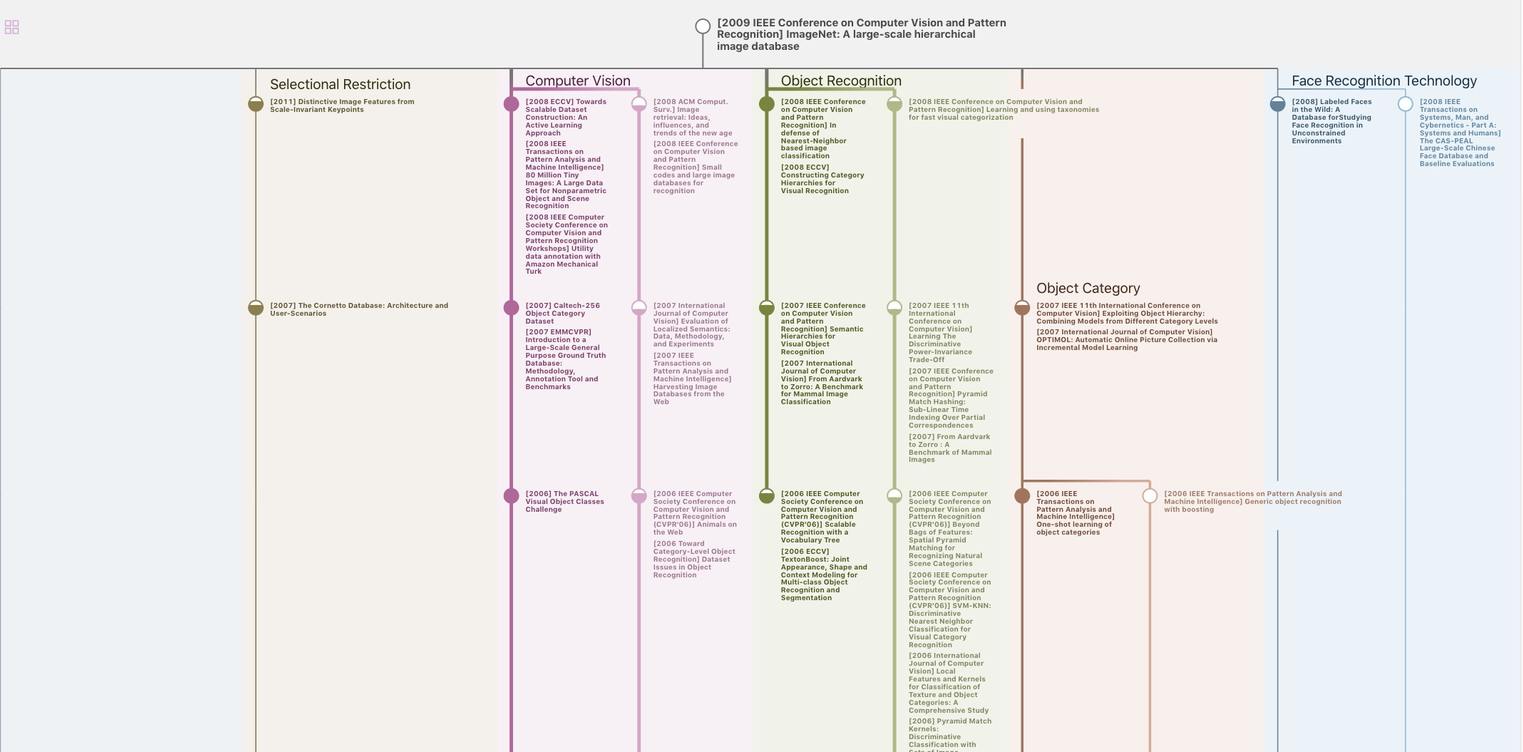
生成溯源树,研究论文发展脉络
Chat Paper
正在生成论文摘要