Deep Learning From Heterogeneous Sequences Of Sparse Medical Data For Early Prediction Of Sepsis
PROCEEDINGS OF THE 13TH INTERNATIONAL JOINT CONFERENCE ON BIOMEDICAL ENGINEERING SYSTEMS AND TECHNOLOGIES, VOL 5: HEALTHINF(2020)
摘要
Sepsis is a life-threatening complication to infections, and early treatment is key for survival. Symptoms of sepsis are difficult to recognize, but prediction models using data from electronic health records (EHRs) can facilitate early detection and intervention. Recently, deep learning architectures have been proposed for the early prediction of sepsis. However, most efforts rely on high-resolution data from intensive care units (ICUs). Prediction of sepsis in the non-ICU setting, where hospitalization periods vary greatly in length and data is more sparse, is not as well studied. It is also not clear how to learn effectively from longitudinal EHR data, which can be represented as a sequence of time windows. In this article, we evaluate the use of an LSTM network for early prediction of sepsis according to Sepsis-3 criteria in a general hospital population. An empirical investigation using six different time window sizes is conducted. The best model uses a two-hour window and assumes data is missing not at random, clearly outperforming scoring systems commonly used in healthcare today. It is concluded that the size of the time window has a considerable impact on predictive performance when learning from heterogeneous sequences of sparse medical data for early prediction of sepsis.
更多查看译文
关键词
Sepsis,Early Prediction,Machine Learning,Deep Learning,Health Informatics,Healthcare Analytics
AI 理解论文
溯源树
样例
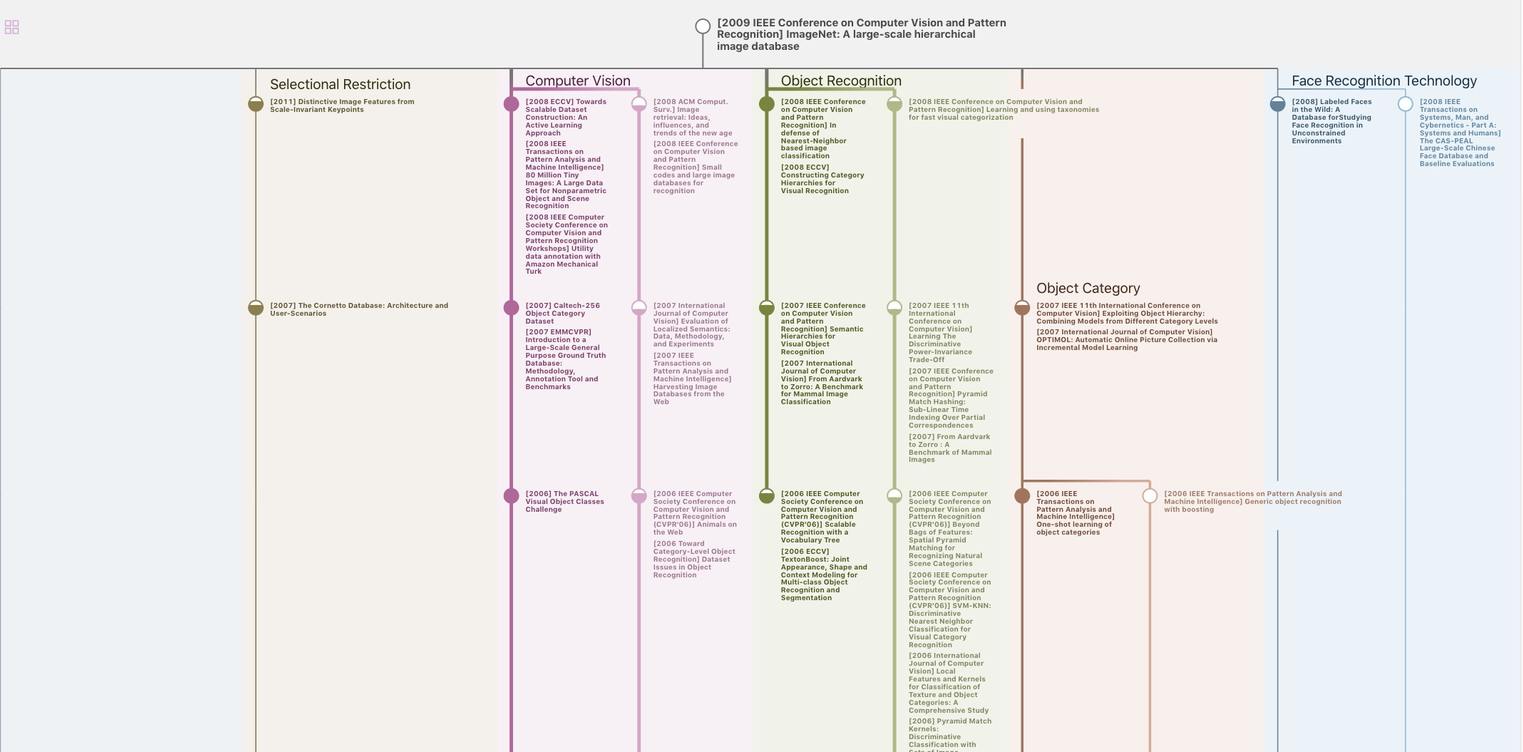
生成溯源树,研究论文发展脉络
Chat Paper
正在生成论文摘要