State-Only Imitation Learning for Dexterous Manipulation
2021 IEEE/RSJ INTERNATIONAL CONFERENCE ON INTELLIGENT ROBOTS AND SYSTEMS (IROS)(2021)
摘要
Modern model-free reinforcement learning methods have recently demonstrated impressive results on a number of problems. However, complex domains like dexterous manipulation remain a challenge due to the high sample complexity. To address this, current approaches employ expert demonstrations in the form of state-action pairs, which are difficult to obtain for real-world settings such as learning from videos. In this paper, we move toward a more realistic setting and explore state-only imitation learning. To tackle this setting, we train an inverse dynamics model and use it to predict actions for state-only demonstrations. The inverse dynamics model and the policy are trained jointly. Our method performs on par with state-action approaches and considerably outperforms RL alone. By not relying on expert actions, we are able to learn from demonstrations with different dynamics, morphologies, and objects. Videos available on the project page.
更多查看译文
关键词
imitation learning,dexterous manipulation,state-only
AI 理解论文
溯源树
样例
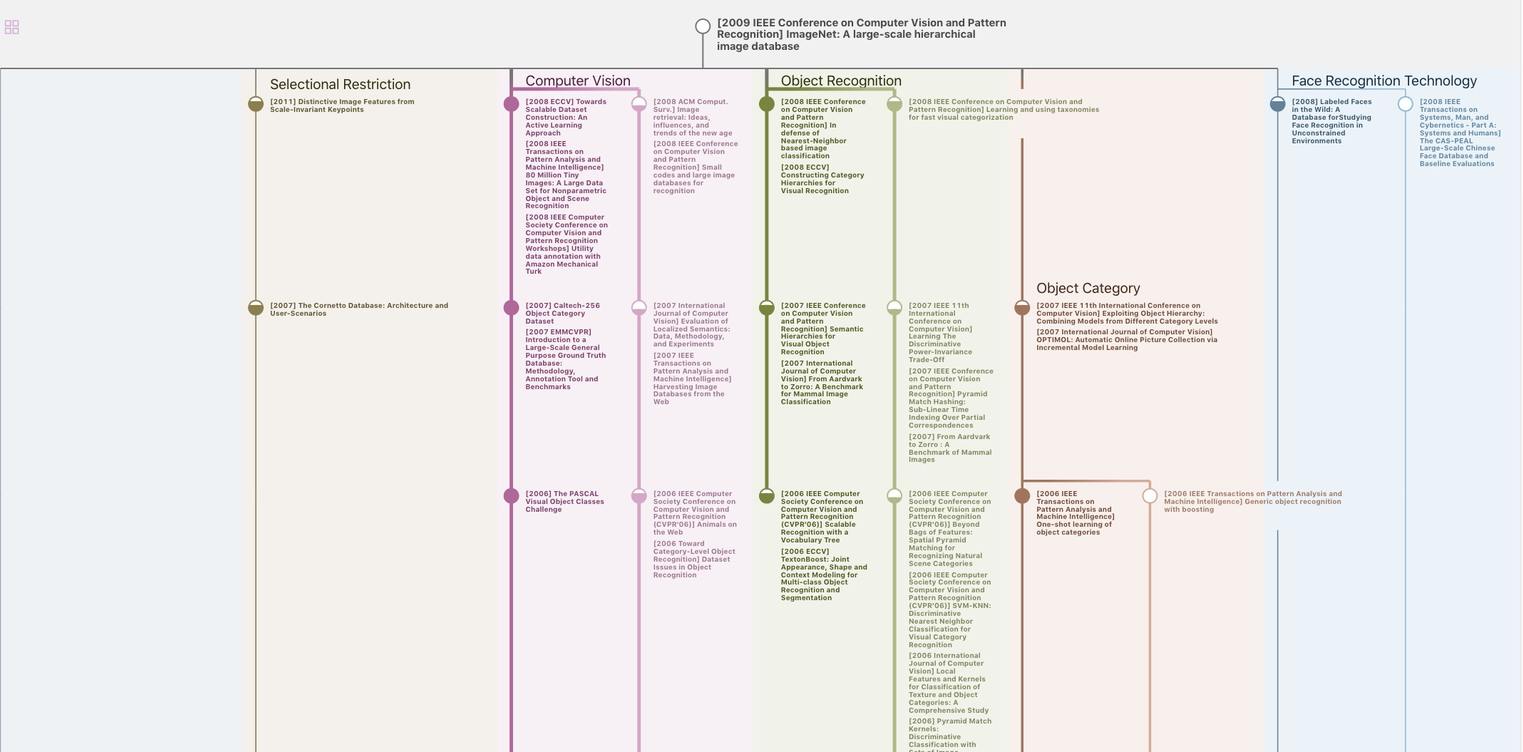
生成溯源树,研究论文发展脉络
Chat Paper
正在生成论文摘要