Improving Disfluency Detection by Self-Training a Self-Attentive Model.
58TH ANNUAL MEETING OF THE ASSOCIATION FOR COMPUTATIONAL LINGUISTICS (ACL 2020)(2020)
摘要
Self-attentive neural syntactic parsers using contextualized word embeddings (e.g. ELMo or BERT) currently produce state-of-the-art results in joint parsing and disfluency detection in speech transcripts. Since the contextualized word embeddings are pre-trained on a large amount of unlabeled data, using additional unlabeled data to train a neural model might seem redundant. However, we show that self-training - a semi-supervised technique for incorporating unlabeled data - sets a new state-of-the-art for the self-attentive parser on disfluency detection, demonstrating that self-training provides benefits orthogonal to the pre-trained contextualized word representations. We also show that ensembling self-trained parsers provides further gains for disfluency detection.
更多查看译文
关键词
disfluency detection,self-training,self-attentive
AI 理解论文
溯源树
样例
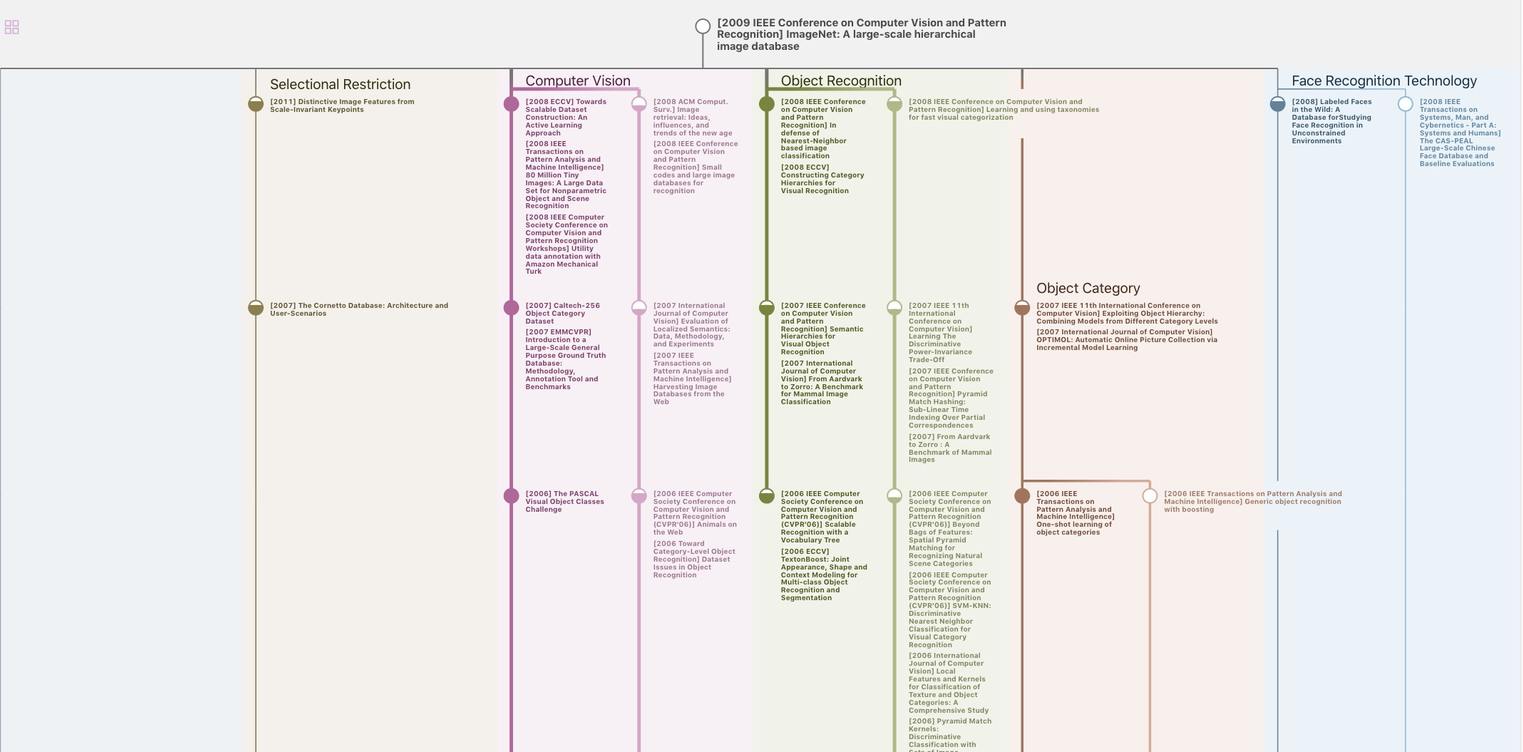
生成溯源树,研究论文发展脉络
Chat Paper
正在生成论文摘要