A Non-Parametric Test to Detect Data-Copying in Generative Models
INTERNATIONAL CONFERENCE ON ARTIFICIAL INTELLIGENCE AND STATISTICS, VOL 108(2020)
摘要
Detecting overfitting in generative models is an important challenge in machine learning. In this work, we formalize a form of overfitting that we call {\em{data-copying}} -- where the generative model memorizes and outputs training samples or small variations thereof. We provide a three sample non-parametric test for detecting data-copying that uses the training set, a separate sample from the target distribution, and a generated sample from the model, and study the performance of our test on several canonical models and datasets. For code \& examples, visit https://github.com/casey-meehan/data-copying
更多查看译文
关键词
models,non-parametric,data-copying
AI 理解论文
溯源树
样例
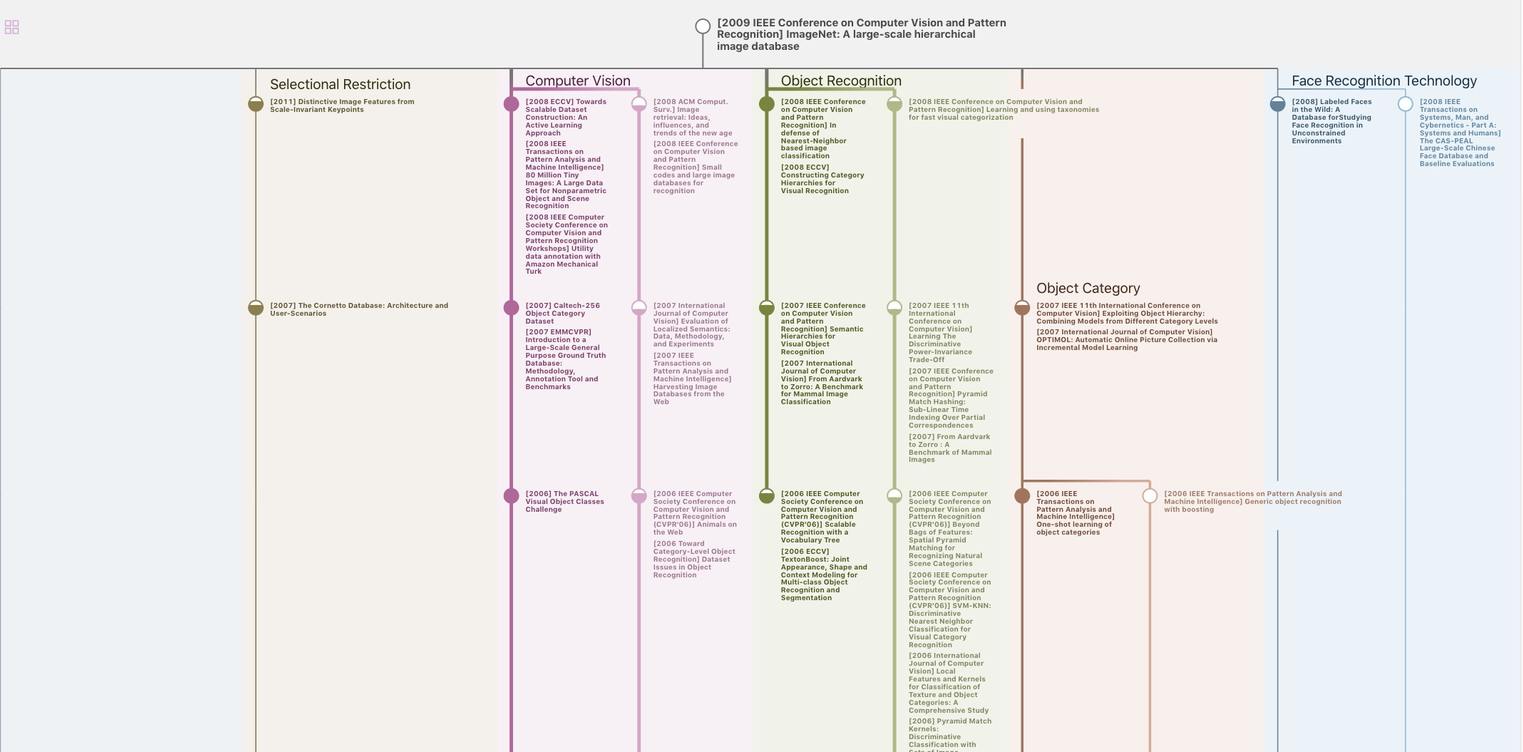
生成溯源树,研究论文发展脉络
Chat Paper
正在生成论文摘要