Enabling Incremental Knowledge Transfer for Object Detection at the Edge
CVPR Workshops(2020)
摘要
Object detection using deep neural networks (DNNs) involves a huge amount of computation which impedes its implementation on resource/energy-limited user-end devices. The reason for the success of DNNs is due to having knowledge over all different domains of observed environments. However, we need a limited knowledge of the observed environment at inference time which can be learned using a shallow neural network (SHNN). In this paper, a systemlevel design is proposed to improve the energy consumption of object detection on the user-end device. An SHNN is deployed on the user-end device to detect objects in the observing environment. Also, a knowledge transfer mechanism is implemented to update the SHNN model using the DNN knowledge when there is a change in the object domain. DNN knowledge can be obtained from a powerful edge device connected to the user-end device through LAN or Wi-Fi. Experiments demonstrate that the energy consumption of the user-end device and the inference time can be improved by 78% and 40% compared with running the deep model on the user-end device.
更多查看译文
关键词
incremental knowledge transfer,shallow neural network,DNNs,deep neural networks,object detection,energy consumption,object domain,DNN knowledge,knowledge transfer mechanism,observing environment,user-end device
AI 理解论文
溯源树
样例
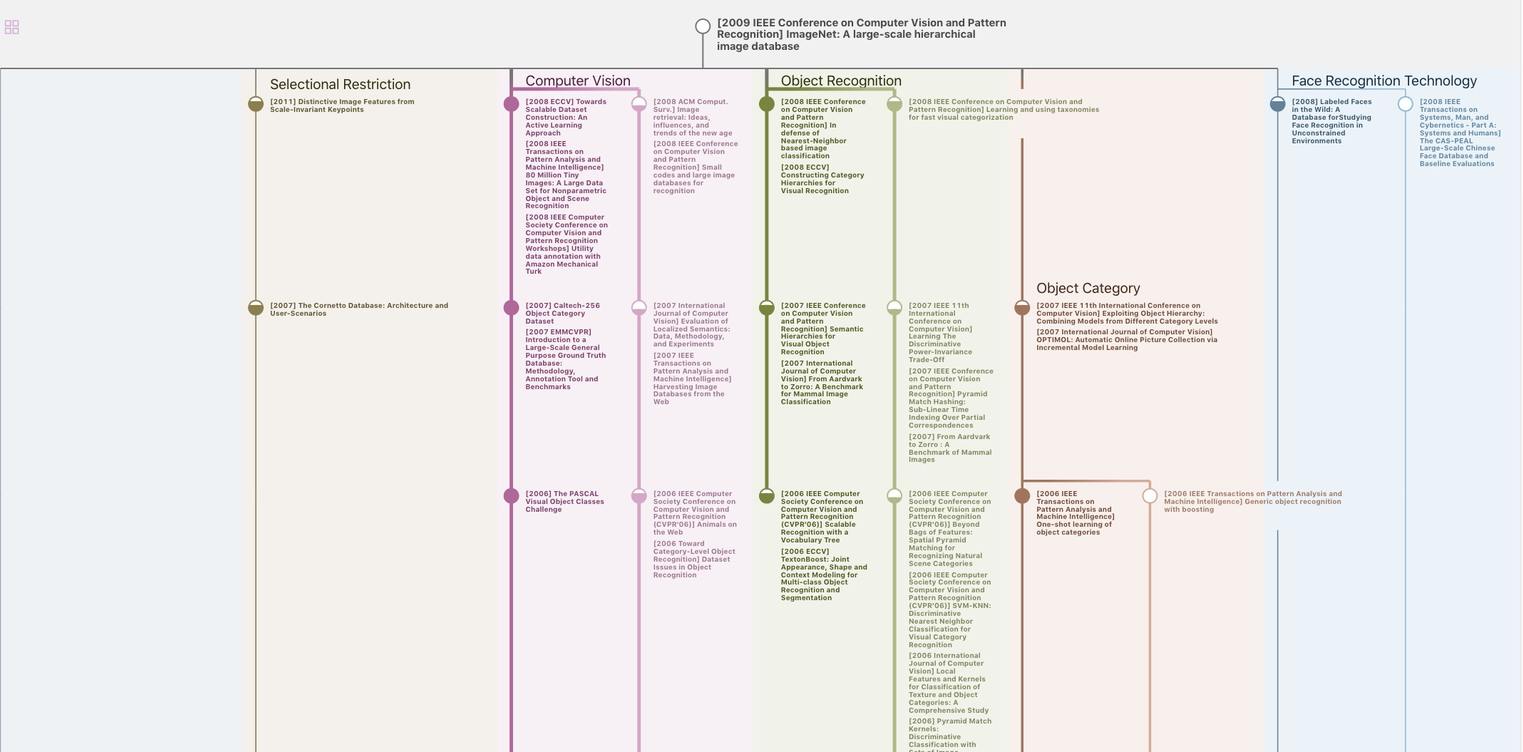
生成溯源树,研究论文发展脉络
Chat Paper
正在生成论文摘要