Variable Rate Image Compression Method with Dead-zone Quantizer
2020 IEEE/CVF Conference on Computer Vision and Pattern Recognition Workshops (CVPRW)(2020)
摘要
Deep learning based image compression methods have achieved superior performance compared with transform based conventional codec. With end-to-end Rate-Distortion Optimization (RDO) in the codec, compression model is optimized with Lagrange multiplier λ. For conventional codec, signal is decorrelated with orthonormal transformation, and uniform quantizer is introduced. We propose a variable rate image compression method with dead-zone quantizer. Firstly, the autoencoder network is trained with RaDOGAGA [6] framework, which can make the latents isometric to the metric space, such as SSIM and MSE. Then the conventional dead-zone quantization method with arbitrary step size is used in the common trained network to provide the flexible rate control. With dead-zone quantizer, the experimental results show that our method performs comparably with independently optimized models within a wide range of bitrate.
更多查看译文
关键词
variable rate image compression method,dead-zone quantization method,flexible rate control,image compression methods,transform based conventional codec,compression model,uniform quantizer,end-to-end rate-distortion optimization,autoencoder network,RaDOGAGA framework
AI 理解论文
溯源树
样例
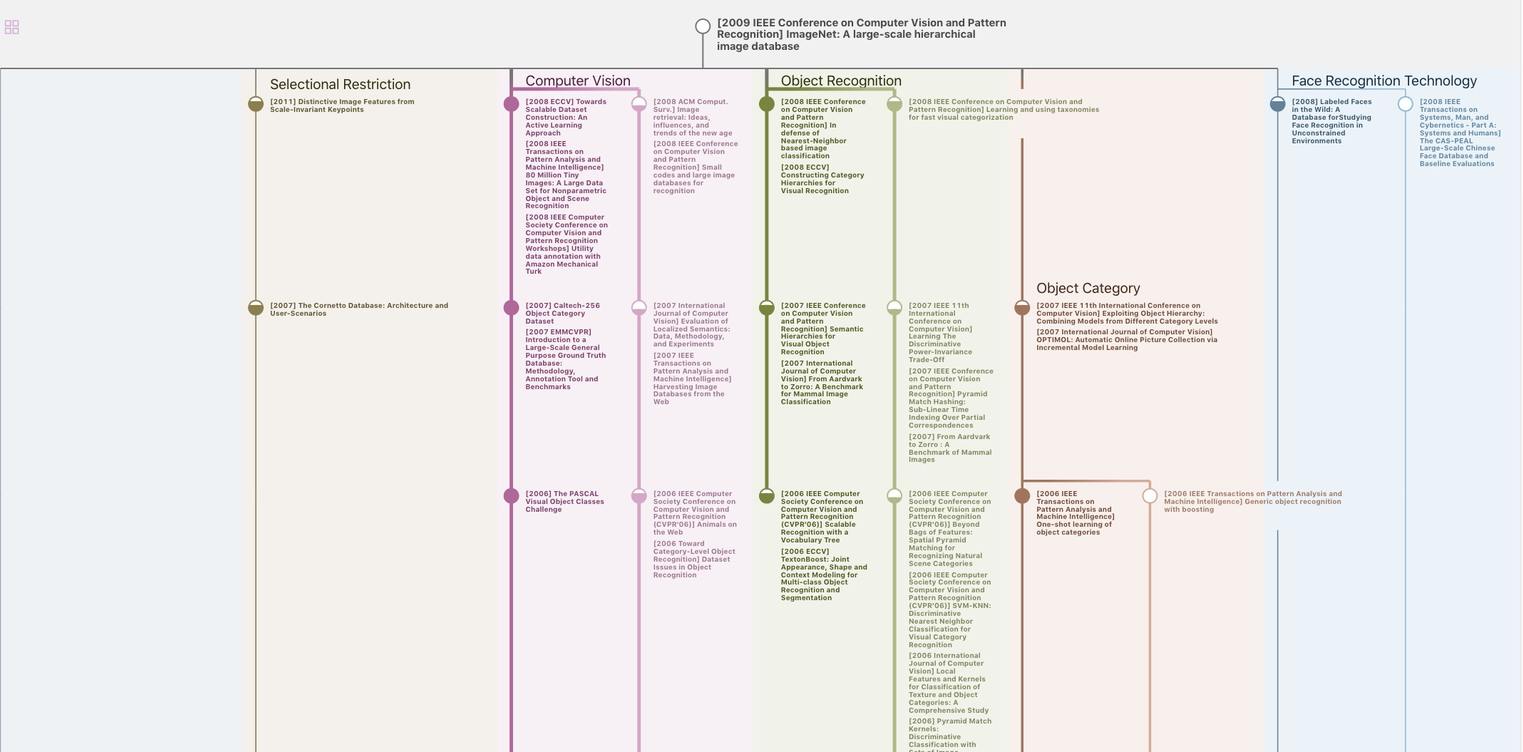
生成溯源树,研究论文发展脉络
Chat Paper
正在生成论文摘要