SLIM: Scalable Linkage of Mobility Data
SIGMOD/PODS '20: International Conference on Management of Data Portland OR USA June, 2020(2020)
摘要
We present a scalable solution to link entities across mobility datasets using their spatio-temporal information. This is a fundamental problem in many applications such as linking user identities for security, understanding privacy limitations of location based services, or producing a unified dataset from multiple sources for urban planning. Such integrated datasets are also essential for service providers to optimise their services and improve business intelligence. In this paper, we first propose a mobility based representation and similarity computation for entities. An efficient matching process is then developed to identify the final linked pairs, with an automated mechanism to decide when to stop the linkage. We scale the process with a locality-sensitive hashing (LSH) based approach that significantly reduces candidate pairs for matching. To realize the effectiveness and efficiency of our techniques in practice, we introduce an algorithm called SLIM. In the experimental evaluation, SLIM outperforms the two existing state-of-the-art approaches in terms of precision and recall. Moreover, the LSH-based approach brings two to four orders of magnitude speedup.
更多查看译文
关键词
mobility data, data integration, scalability, entity linkage
AI 理解论文
溯源树
样例
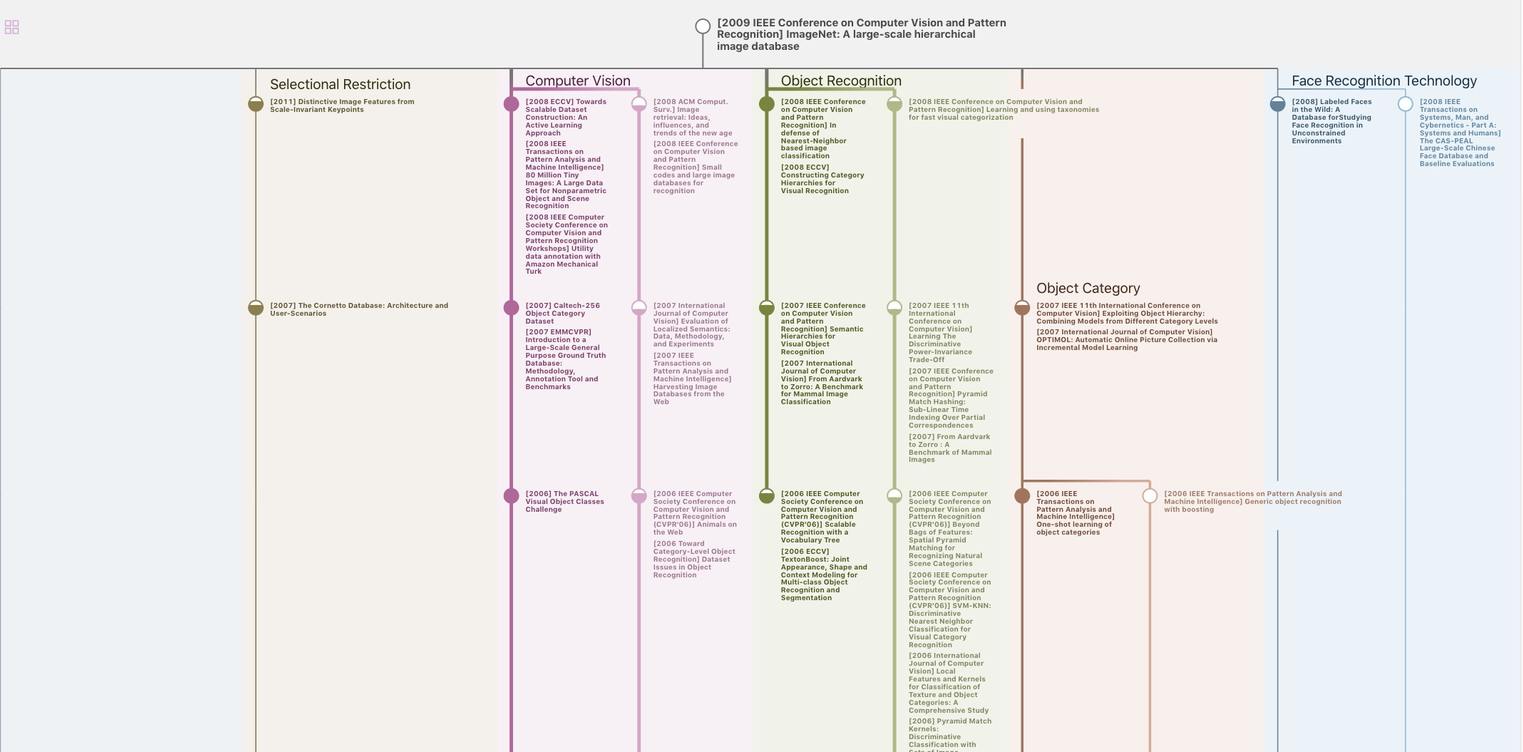
生成溯源树,研究论文发展脉络
Chat Paper
正在生成论文摘要