Learning local shape descriptors for computing non-rigid dense correspondence
Computational Visual Media(2020)
摘要
A discriminative local shape descriptor plays an important role in various applications. In this paper, we present a novel deep learning framework that derives discriminative local descriptors for deformable 3D shapes. We use local “geometry images” to encode the multi-scale local features of a point, via an intrinsic parameterization method based on geodesic polar coordinates. This new parameterization provides robust geometry images even for badly-shaped triangular meshes. Then a triplet network with shared architecture and parameters is used to perform deep metric learning; its aim is to distinguish between similar and dissimilar pairs of points. Additionally, a newly designed triplet loss function is minimized for improved, accurate training of the triplet network. To solve the dense correspondence problem, an efficient sampling approach is utilized to achieve a good compromise between training performance and descriptor quality. During testing, given a geometry image of a point of interest, our network outputs a discriminative local descriptor for it. Extensive testing of non-rigid dense shape matching on a variety of benchmarks demonstrates the superiority of the proposed descriptors over the state-of-the-art alternatives.
更多查看译文
关键词
local feature descriptor, triplet CNN, dense correspondence, geometry image, non-rigid shape
AI 理解论文
溯源树
样例
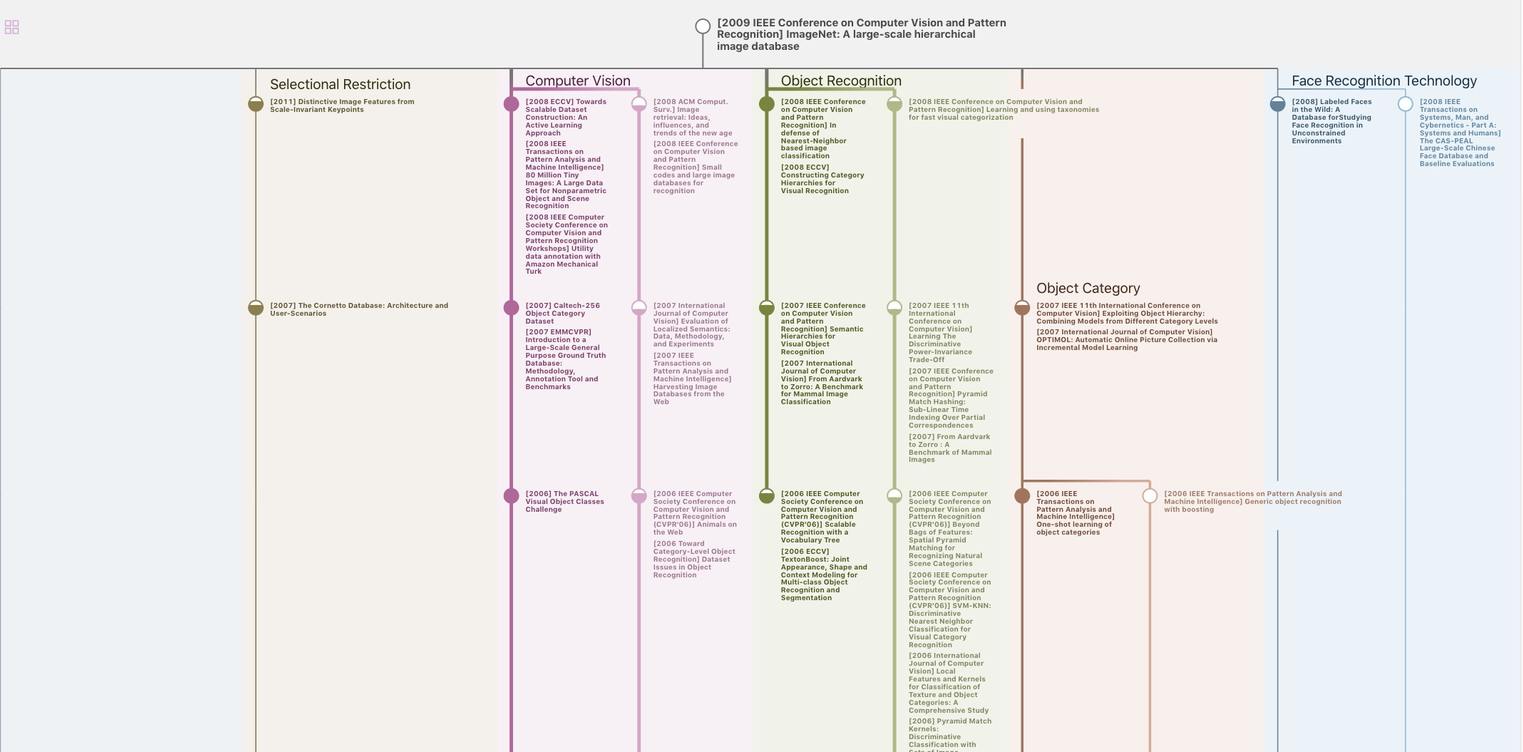
生成溯源树,研究论文发展脉络
Chat Paper
正在生成论文摘要