Densely Distilled Flow-Based Knowledge Transfer In Teacher-Student Framework For Image Classification
IEEE TRANSACTIONS ON IMAGE PROCESSING(2020)
摘要
We propose a new teacher-student framework (TSF)-based knowledge transfer method, in which knowledge in the form of dense flow across layers is distilled from a pre-trained "teacher" deep neural network (DNN) and transferred to another "student" DNN. In the case of distilled knowledge, multiple overlapped flow-based items of information from the pre-trained teacher DNN are densely extracted across layers. Transference of the densely extracted teacher information is then achieved in the TSF using repetitive sequential training from bottom to top between the teacher and student DNN models. In other words, to efficiently transmit extracted useful teacher information to the student DNN, we perform bottom-up step-by-step transfer of densely distilled knowledge. The performance of the proposed method in terms of image classification accuracy and fast optimization is compared with those of existing TSF-based knowledge transfer methods for application to reliable image datasets, including CIFAR-10, CIFAR-100, MNIST, and SVHN. When the dense flow-based sequential knowledge transfer scheme is employed in the TSF, the trained student ResNet more accurately reflects the rich information of the pre-trained teacher ResNet and exhibits superior accuracy to the existing TSF-based knowledge transfer methods for all benchmark datasets considered in this study.
更多查看译文
关键词
Knowledge transfer, Training, Computational modeling, Data mining, Optimization, Image classification, Computer architecture, Teacher-student framework, image classification, densely distilled knowledge, knowledge transfer, residual network
AI 理解论文
溯源树
样例
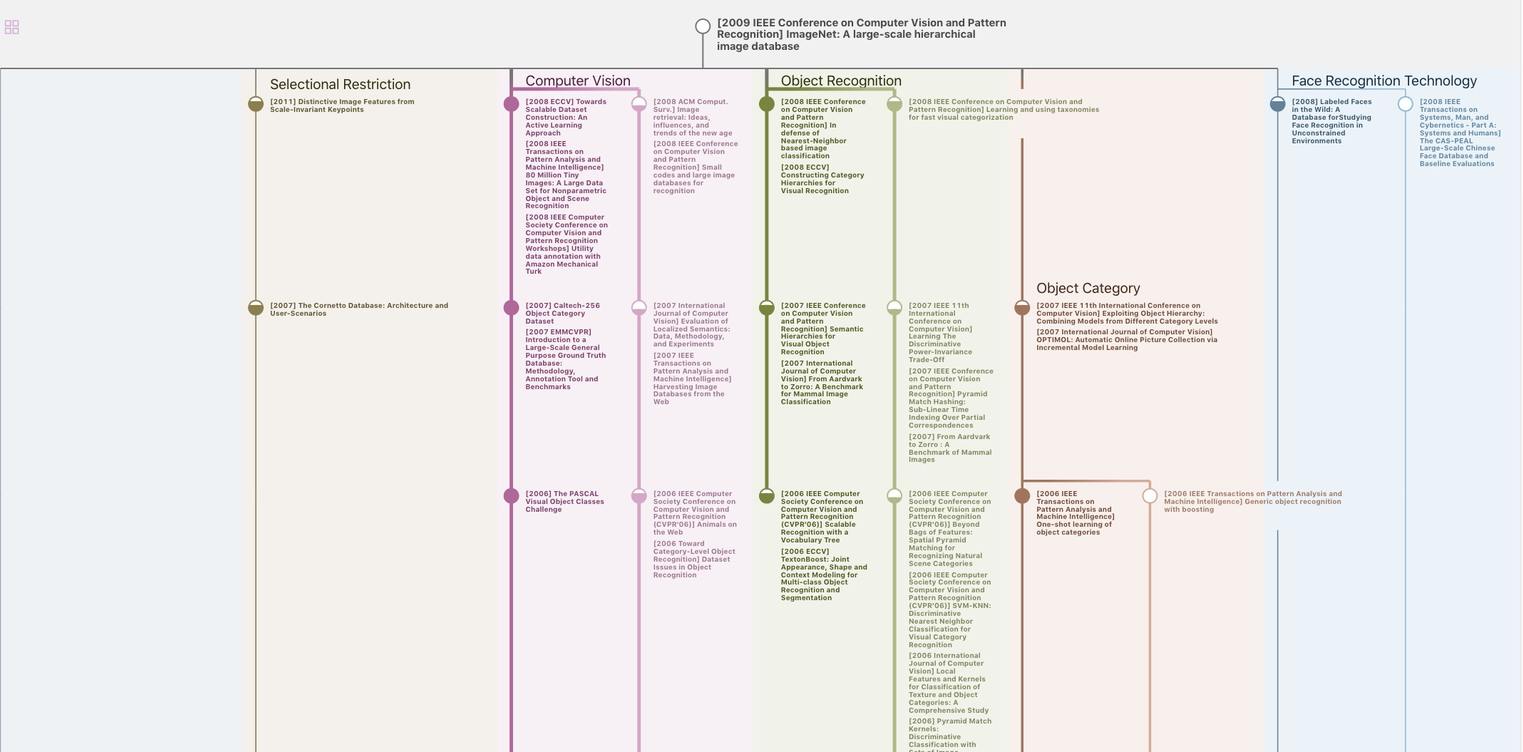
生成溯源树,研究论文发展脉络
Chat Paper
正在生成论文摘要