Anisotropic Convolution For Image Classification
IEEE TRANSACTIONS ON IMAGE PROCESSING(2020)
摘要
Convolutional neural networks are built upon simple but useful convolution modules. The traditional convolution has a limitation on feature extraction and object localization due to its fixed scale and geometric structure. Besides, the loss of spatial information also restricts the networks' performance and depth. To overcome these limitations, this paper proposes a novel anisotropic convolution by adding a scale factor and a shape factor into the traditional convolution. The anisotropic convolution augments the receptive fields flexibly and dynamically depending on the valid sizes of objects. In addition, the anisotropic convolution is a generalized convolution. The traditional convolution, dilated convolution and deformable convolution can be viewed as its special cases. Furthermore, in order to improve the training efficiency and avoid falling into a local optimum, this paper introduces a simplified implementation of the anisotropic convolution. The anisotropic convolution can be applied to arbitrary convolutional networks and the enhanced networks are called ACNs (anisotropic convolutional networks). Experimental results show that ACNs achieve better performance than many state-of-the-art methods and the baseline networks in tasks of image classification and object localization, especially in classification task of tiny images.
更多查看译文
关键词
Convolution, Shape, Kernel, Feature extraction, Task analysis, Training, Neural networks, Anisotropic convolution, image classification, object localization
AI 理解论文
溯源树
样例
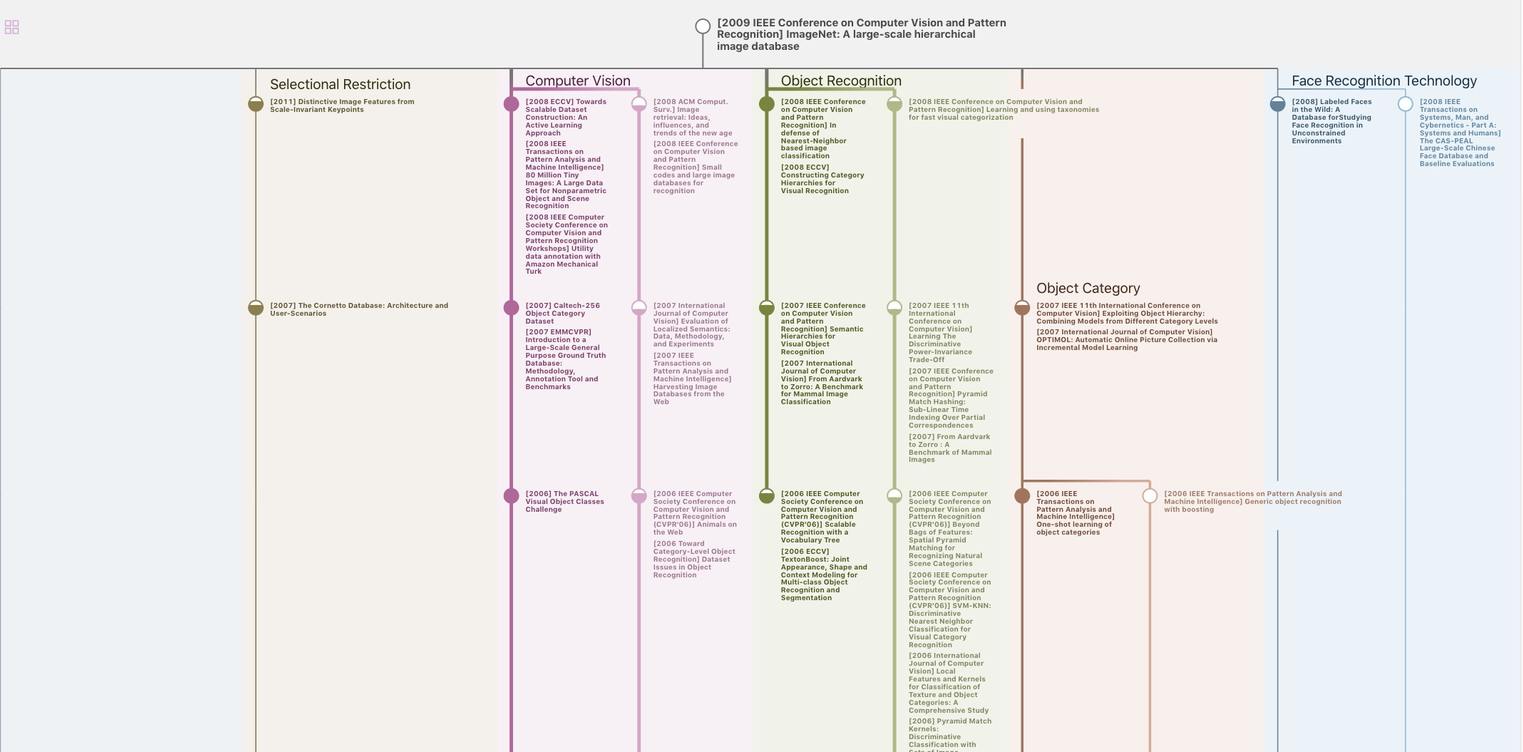
生成溯源树,研究论文发展脉络
Chat Paper
正在生成论文摘要