Unsupervised learning of wildlife behaviour for activity-driven opportunistic beacon networks
2019 13th International Conference on Sensing Technology (ICST)(2019)
摘要
Monitoring wild animals in their natural habitat and in real time constitutes an essential aspect of biological and environmental studies. Monitoring is mainly conducted through wireless wildlife monitoring systems (WMS) due to their energy-efficiency and scalability properties. However, using WMS often involves the deployment of energy demanding wireless radio technologies and protocols that significantly increase energy consumption while tracking mobile animals. Thanks to the raise of IoT devices capable of sensing, computing, and wireless networking, WMS can become more efficient and overcome the initial drawbacks. This paper, describes an activity driven beaconing mechanism based on unsupervised activity classification scheme. The algorithm is evaluated for different parameters involving the sampling rate, processing window as well as different cluster sizes. The evaluation shows that use of lightweight algorithms and low sampling rates provides the possibility to reliably monitor the activity of the animal. The evaluation results showed that the proposed mechanism could reduce energy consumption by increasing communication sleep-time while the objects were stationary.
更多查看译文
关键词
Wildlife monitoring,IoT,sensor data,BLE,unsupervised learning,Self organizing maps
AI 理解论文
溯源树
样例
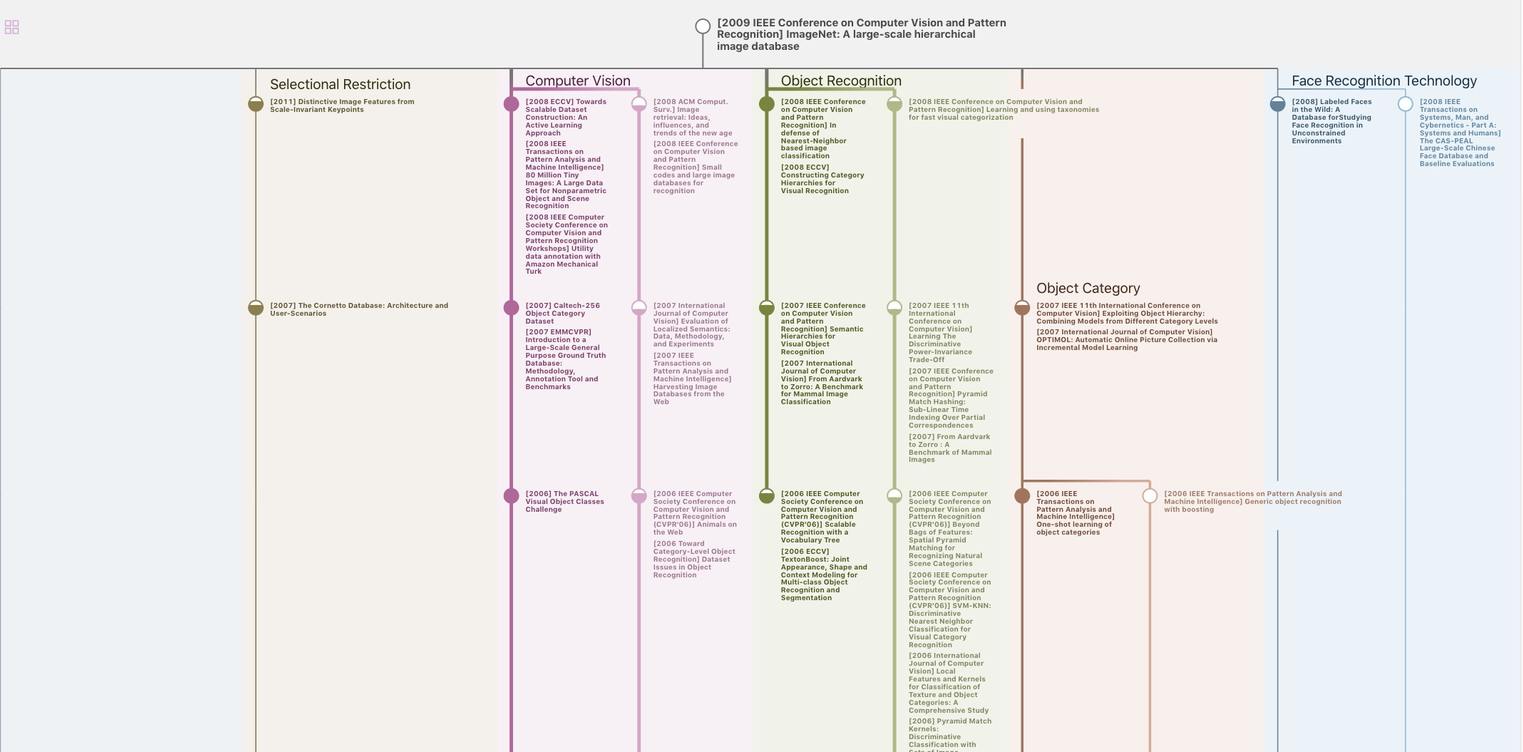
生成溯源树,研究论文发展脉络
Chat Paper
正在生成论文摘要