Condition Monitoring Of Elevator Systems Using Deep Neural Network
PROCEEDINGS OF THE 9TH INTERNATIONAL CONFERENCE ON OPERATIONS RESEARCH AND ENTERPRISE SYSTEMS (ICORES)(2020)
摘要
In this research, we propose a generic deep autoencoder model for automatic calculation of highly informative deep features from the elevator data. Random forest algorithm is used for fault detection based on extracted deep features. Maintenance actions recorded are used to label the sensor data into healthy or faulty. In our research, we have included all fault types present for each elevator. The rest of the healthy data is used for validation of the model to prove its efficacy in terms of avoiding false positives. New extracted deep features provide 100% accuracy in fault detection along with avoiding false positives, which is better than statistical features. Random forest was also used to detect faults based on statistical features to compare results. New deep features extracted from the dataset with deep autoencoder random forest outperform the statistical features. Good classification and robustness against overfitting are key characteristics of our model. This research will help to reduce unnecessary visits of service technicians to installation sites by detecting false alarms in various predictive maintenance systems.
更多查看译文
关键词
Deep Neural Network, Fault Detection, Feature Extraction, Elevator Systems
AI 理解论文
溯源树
样例
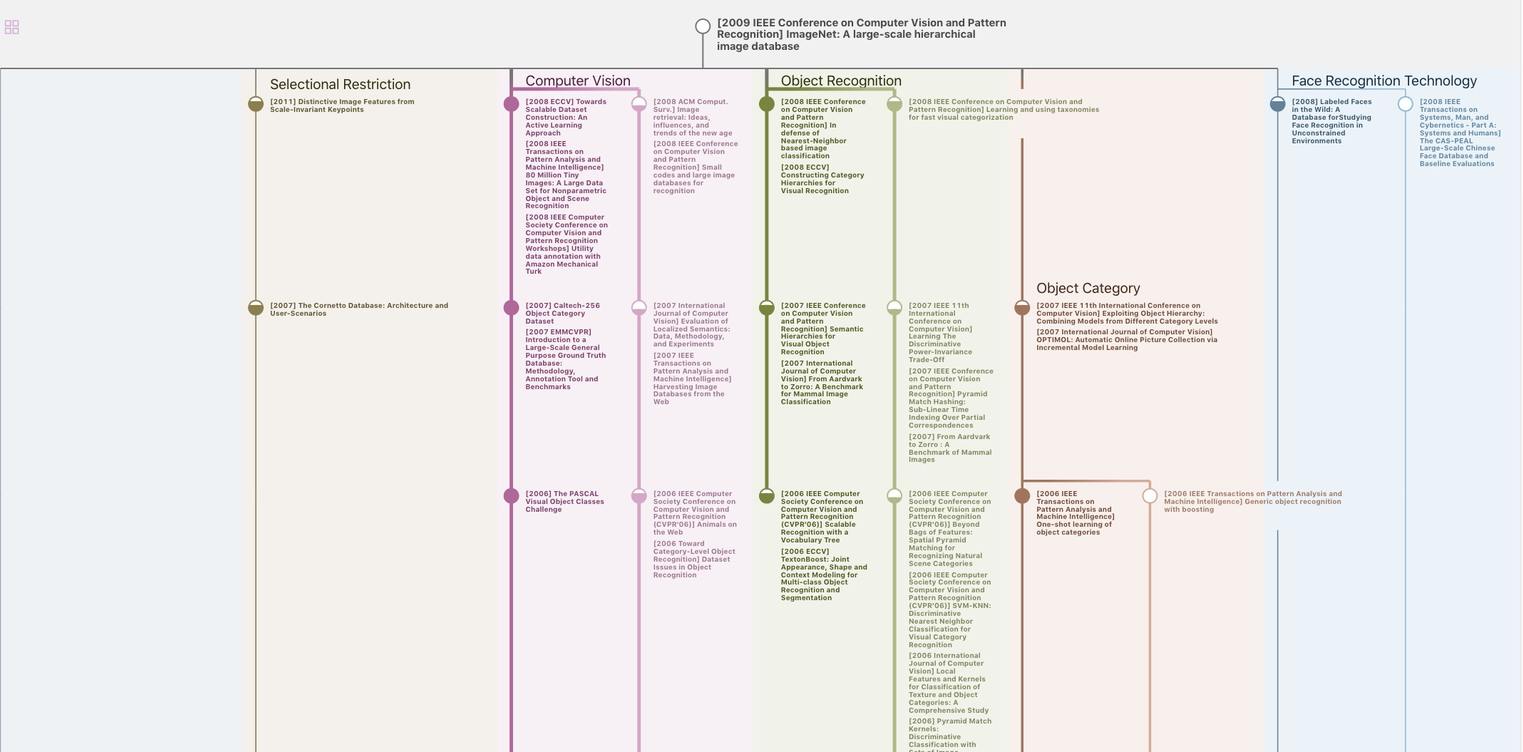
生成溯源树,研究论文发展脉络
Chat Paper
正在生成论文摘要