Speaker Identification Using Techniques Based On One-Shot Learning
PROCESAMIENTO DEL LENGUAJE NATURAL(2020)
摘要
A speaker identification system in order to be effective requires a large number of audio samples of each speaker, which are not always accessible or easy to collect. In contrast, systems based on meta-learning like one-shot learning, use a single sample to differentiate between classes. This work evaluates the potential of applying the meta-learning approach to text-independent speaker identification tasks. In the experimentation mel spectrogram, i-vectors and resample (downsampling) are used to both process the audio signal and to obtain a feature vector. This feature vector is the input of a siamese neural network that is responsible for performing the identification task. The best result was obtained by differentiating between 4 speakers with an accuracy of 0.9. The obtained results show that one-shot learning approaches have great potential to be used speaker identification and could be very useful in a real field like biometrics or forensic because of its versatility.
更多查看译文
关键词
Speaker Identification, Text independent, Meta Learning, N-Way clasification, One-Shot learning, Siamese Neural Network, Voxceleb
AI 理解论文
溯源树
样例
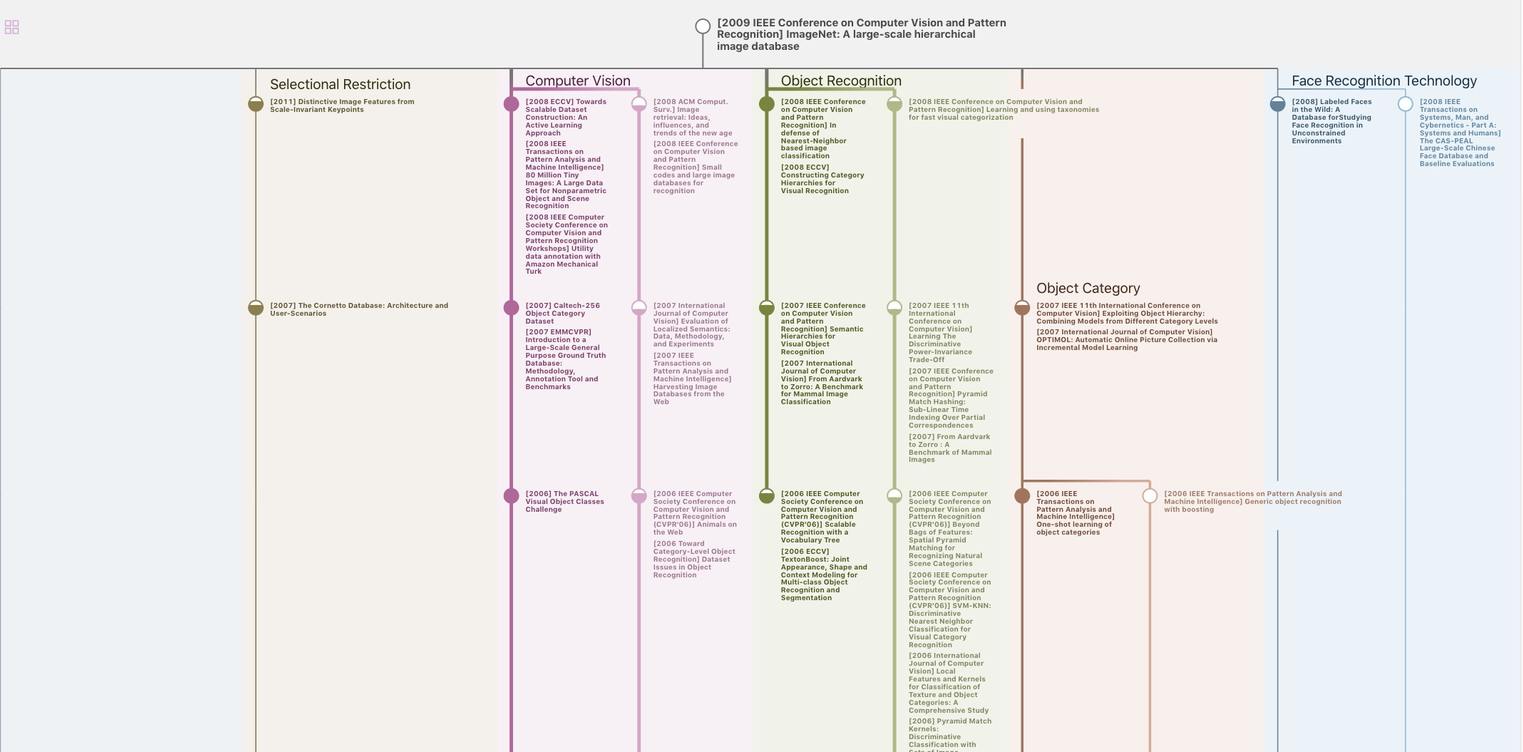
生成溯源树,研究论文发展脉络
Chat Paper
正在生成论文摘要