Deep Neural Network For Water/Fat Separation: Supervised Training, Unsupervised Training, And No Training
arxiv(2021)
摘要
Purpose To use a deep neural network (DNN) for solving the optimization problem of water/fat separation and to compare supervised and unsupervised training.Methods The current T2*-IDEAL algorithm for solving water/fat separation is dependent on initialization. Recently, DNN has been proposed to solve water/fat separation without the need for suitable initialization. However, this approach requires supervised training of DNN using the reference water/fat separation images. Here we propose 2 novel DNN water/fat separation methods: 1) unsupervised training of DNN (UTD) using the physical forward problem as the cost function during training, and 2) no training of DNN using physical cost and backpropagation to directly reconstruct a single dataset. The supervised training of DNN, unsupervised training of DNN, and no training of DNN methods were compared with the reference T2*-IDEAL.Results All DNN methods generated consistent water/fat separation results that agreed well with T2*-IDEAL under proper initialization.Conclusion The water/fat separation problem can be solved using unsupervised deep neural networks.
更多查看译文
关键词
deep learning, label free, unsupervised, water, fat separation
AI 理解论文
溯源树
样例
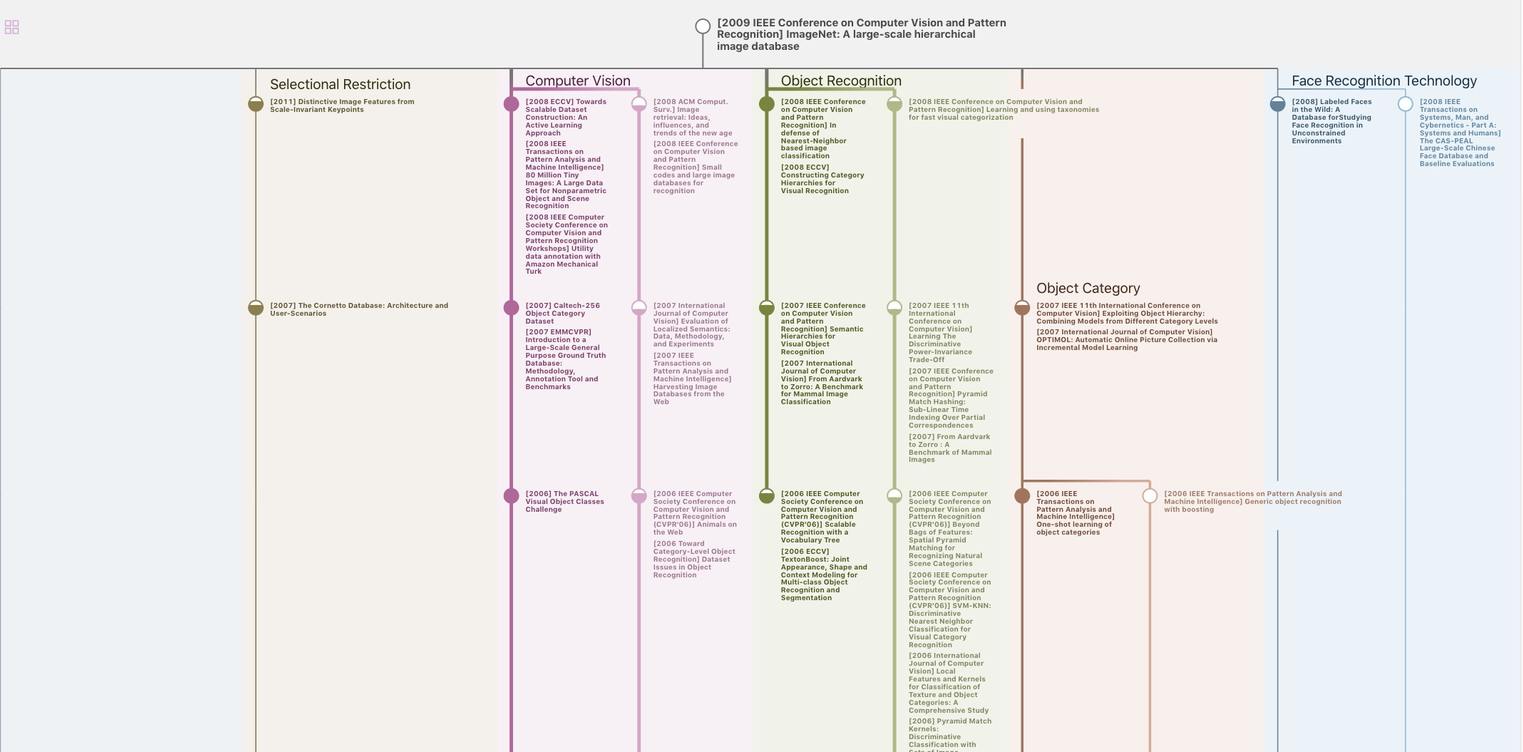
生成溯源树,研究论文发展脉络
Chat Paper
正在生成论文摘要