3-D Context Entropy Model for Improved Practical Image Compression
2020 IEEE/CVF Conference on Computer Vision and Pattern Recognition Workshops (CVPRW)(2020)
摘要
In this paper, we present our image compression framework designed for CLIC 2020 competition. Our method is based on Variational AutoEncoder (VAE) architecture which is strengthened with residual structures. In short, we make three noteworthy improvements here. First, we propose a 3-D context entropy model which can take advantage of known latent representation in current spatial locations for better entropy estimation. Second, a light-weighted residual structure is adopted for feature learning during entropy estimation. Finally, an effective training strategy is introduced for practical adaptation with different resolutions. Experiment results indicate our image compression method achieves 0.9775 MS-SSIM on CLIC validation set and 0.9809 MS-SSIM on test set.
更多查看译文
关键词
entropy estimation,light-weighted residual structure,image compression framework,CLIC 2020 competition,variational autoencoder architecture,residual structures,3D context entropy model,VAE architecture,feature learning,MS-SSIM
AI 理解论文
溯源树
样例
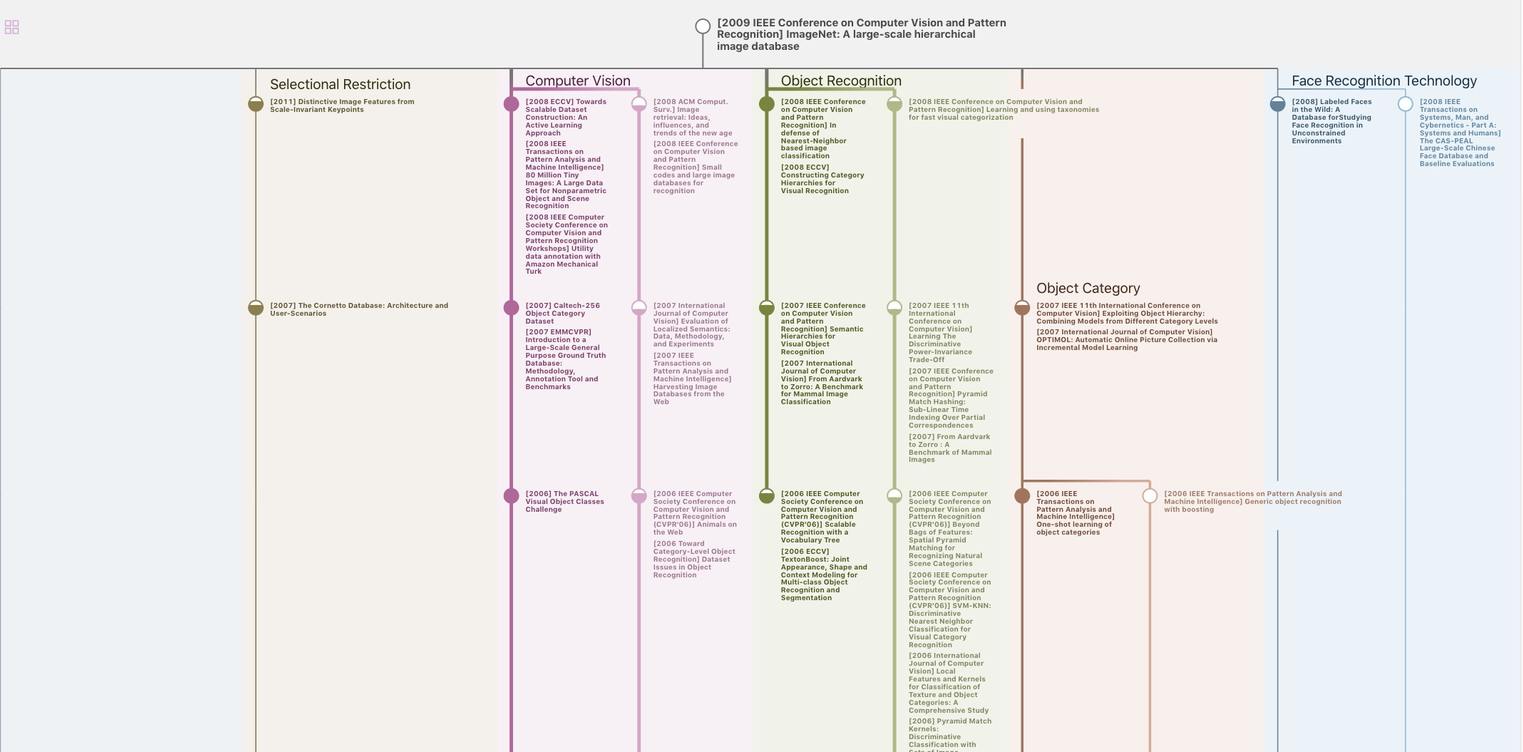
生成溯源树,研究论文发展脉络
Chat Paper
正在生成论文摘要