Disentangled Adversarial Transfer Learning For Physiological Biosignals
42ND ANNUAL INTERNATIONAL CONFERENCES OF THE IEEE ENGINEERING IN MEDICINE AND BIOLOGY SOCIETY: ENABLING INNOVATIVE TECHNOLOGIES FOR GLOBAL HEALTHCARE EMBC'20(2020)
摘要
Recent developments in wearable sensors demonstrate promising results for monitoring physiological status in effective and comfortable ways. One major challenge of physiological status assessment is the problem of transfer learning caused by the domain inconsistency of biosignals across users or different recording sessions from the same user. We propose an adversarial inference approach for transfer learning to extract disentangled nuisance-robust representations from physiological biosignal data in stress status level assessment. We exploit the trade-off between task-related features and person-discriminative information by using both an adversary network and a nuisance network to jointly manipulate and disentangle the learned latent representations by the encoder, which are then input to a discriminative classifier. Results on cross-subjects transfer evaluations demonstrate the benefits of the proposed adversarial framework, and thus show its capabilities to adapt to a broader range of subjects. Finally we highlight that our proposed adversarial transfer learning approach is also applicable to other deep feature learning frameworks.
更多查看译文
关键词
stress level assessment, physiological biosignals, adversarial networks, transfer learning, deep neural networks, disentangled representation learning
AI 理解论文
溯源树
样例
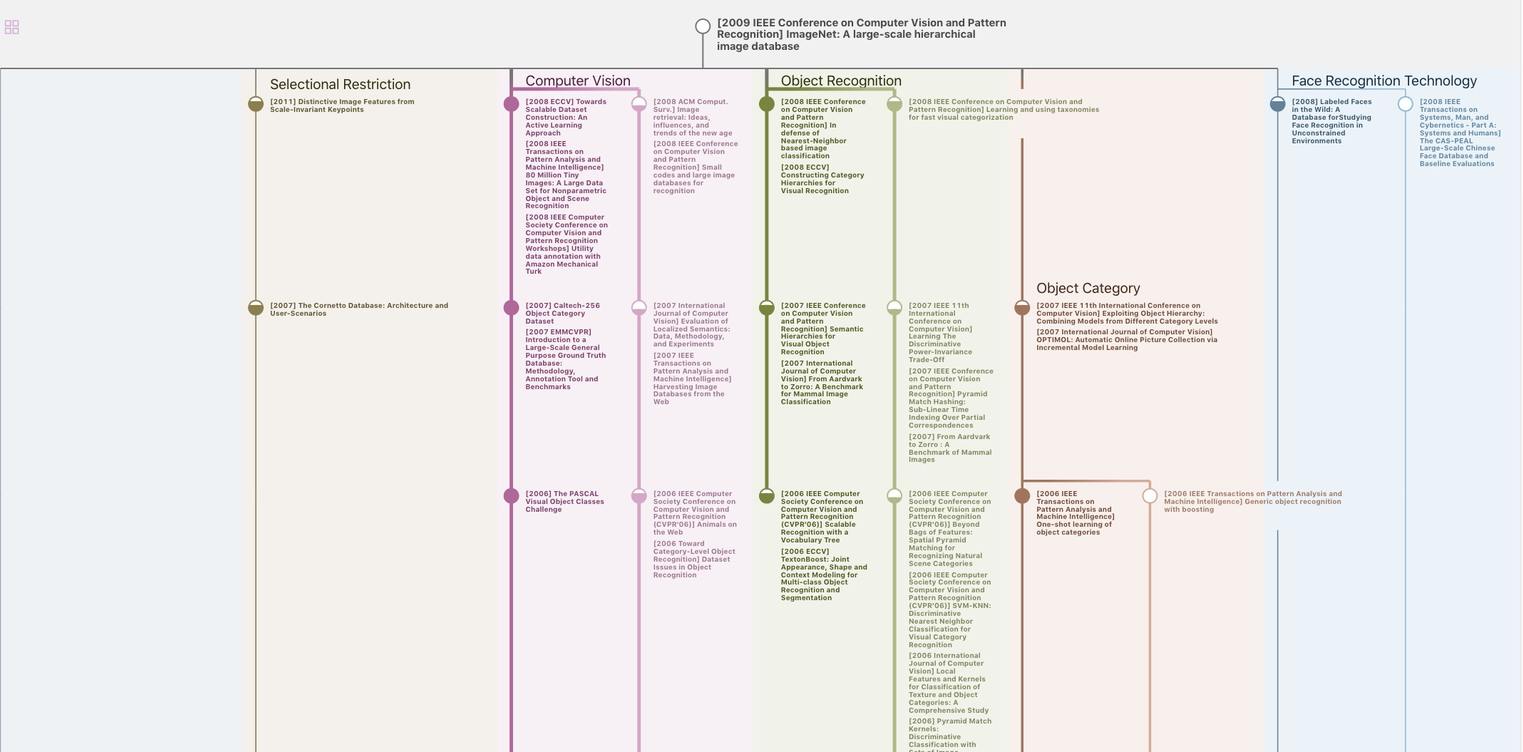
生成溯源树,研究论文发展脉络
Chat Paper
正在生成论文摘要