Learning to Classify: A Flow-Based Relation Network for Encrypted Traffic Classification
WWW '20: The Web Conference 2020 Taipei Taiwan April, 2020(2020)
摘要
As the size and source of network traffic increase, so does the challenge of monitoring and analyzing network traffic. The challenging problems of classifying encrypted traffic are the imbalanced property of network data, the generalization on an unseen dataset, and overly dependent on data size. In this paper, we propose an application of a meta-learning approach to address these problems in encrypted traffic classification, named Flow-Based Relation Network (RBRN). The RBRN is an end-to-end classification model that learns representative features from the raw flows and then classifies them in a unified framework. Moreover, we design “hallucinator” to produce additional training samples for the imbalanced classification, and then focus on meta-learning to classify unseen categories from few labeled samples. We validate the effectiveness of the RBRN on the real-world network traffic dataset, and the experimental results demonstrate that the RBRN can achieve an excellent classification performance and outperform the state-of-the-art methods on encrypted traffic classification. What is more interesting, our model trained on the real-world dataset can generalize very well to unseen datasets, outperforming multiple state-of-art methods.
更多查看译文
关键词
traffic classification, meta-learning, relational networks, data augmentation
AI 理解论文
溯源树
样例
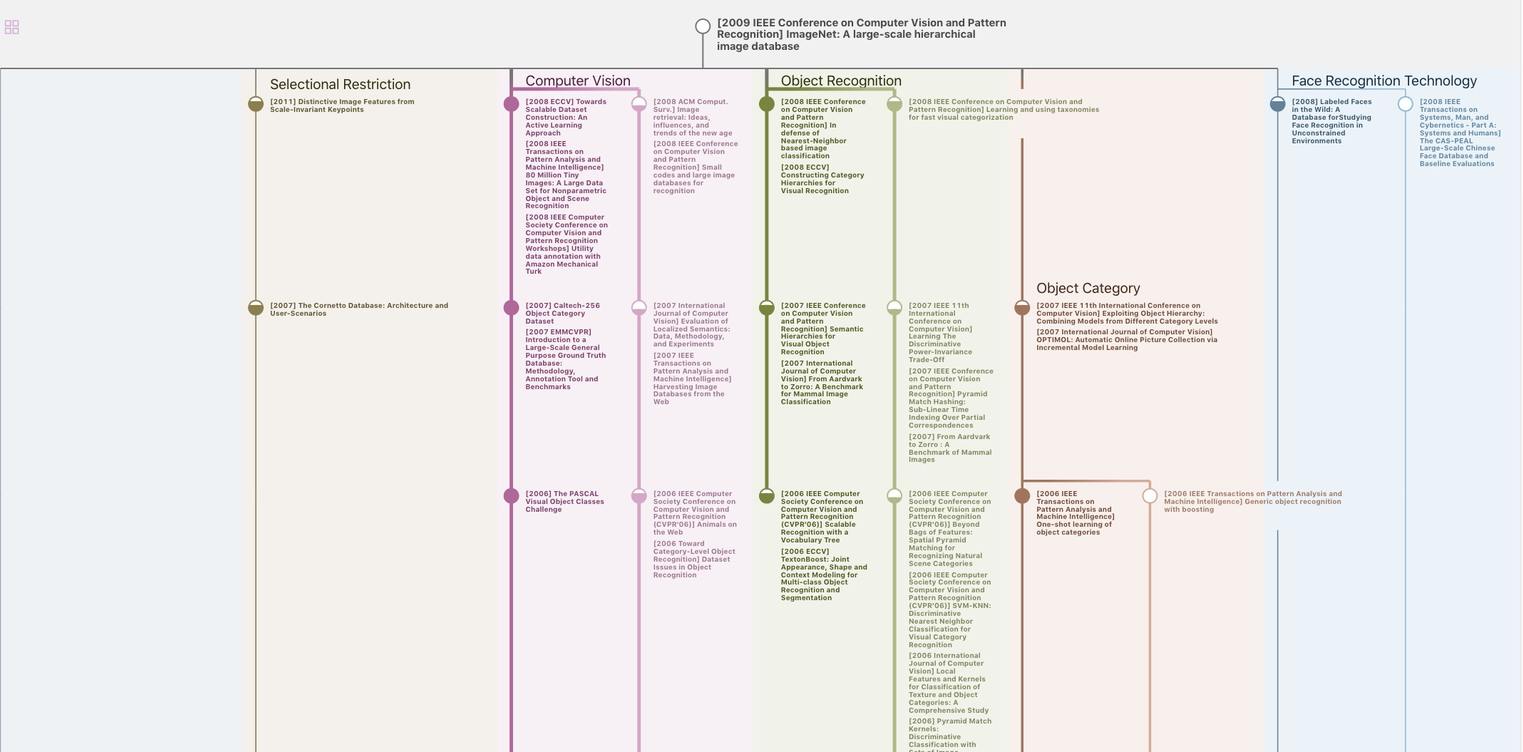
生成溯源树,研究论文发展脉络
Chat Paper
正在生成论文摘要